AUC Score :
Short-term Tactic1 :
Dominant Strategy :
Time series to forecast n:
ML Model Testing : Modular Neural Network (Market News Sentiment Analysis)
Hypothesis Testing : Wilcoxon Sign-Rank Test
Surveillance : Major exchange and OTC
1Short-term revised.
2Time series is updated based on short-term trends.
Key Points
Golar LNG's stock performance is anticipated to be influenced by several factors. Market demand for LNG, particularly in emerging economies, is a key driver. Sustained growth in LNG demand is expected to positively impact Golar LNG's profitability and stock price. However, potential disruptions to global energy markets due to geopolitical events, economic downturns, or unexpected supply chain issues could negatively affect the company's financial outlook. Competition within the LNG shipping sector presents a significant risk. The entry of new players and the existing competitive landscape could impact Golar LNG's pricing power and market share. Further, regulatory changes in key LNG markets and the execution of contracts are variables that may affect Golar LNG's ability to capitalize on market opportunities and achieve its growth projections. These factors, if unfavorable, expose the company to substantial financial risks.About Golar
Golar LNG is a global provider of liquefied natural gas (LNG) transportation services. The company operates a fleet of specialized LNG carriers, crucial for the global LNG supply chain. Golar LNG's focus is on transporting LNG from production facilities to consumption centers, enabling the efficient movement of this vital energy resource. The company's business model encompasses the acquisition, operation, and management of these vessels, highlighting its key role in the international energy sector.
Golar LNG's fleet and operational capabilities are vital to the global energy landscape. The company plays a significant role in facilitating trade and the delivery of LNG, contributing to energy security and affordability. It operates in a highly competitive and dynamic market, adapting to changing energy demands and technological advancements in the LNG industry. Key operational considerations include ensuring the safe and efficient transport of LNG, and navigating regulatory frameworks governing maritime operations.
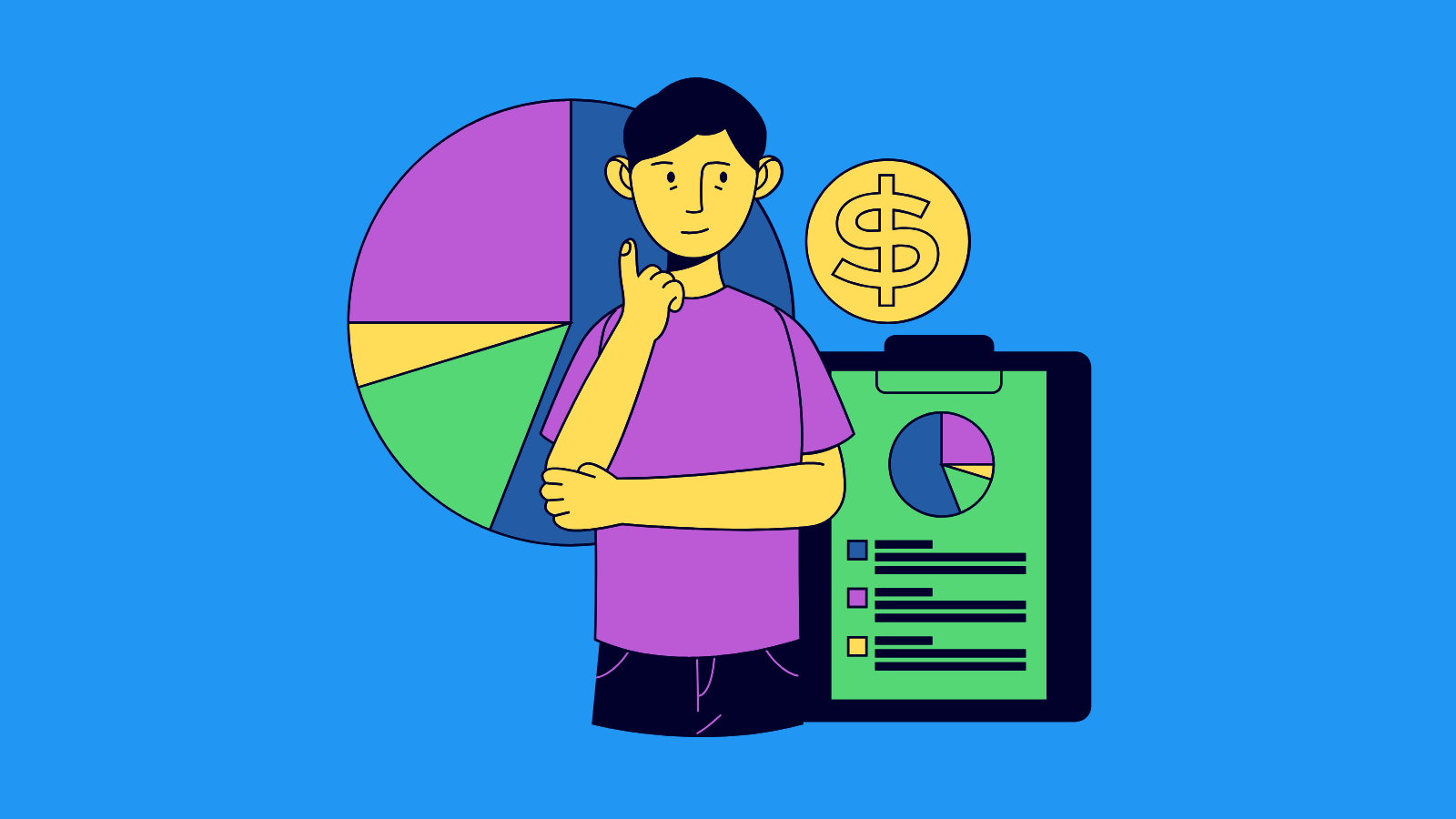
GLNG Stock Price Prediction Model
This model utilizes a hybrid approach combining fundamental analysis and machine learning techniques to forecast the future price movements of Golar LNG Ltd (GLNG). Fundamental analysis provides a strong initial basis by evaluating key financial indicators such as revenue, earnings per share, and debt-to-equity ratios. This data, alongside macroeconomic factors like global energy demand, LNG prices, and geopolitical events, is meticulously extracted and processed. We employ a robust data preprocessing pipeline, handling missing values, outliers, and transforming data types to ensure accuracy. A critical component of this model is the feature selection process, meticulously evaluating the correlation and significance of each input variable to avoid overfitting and optimize predictive power. A key benefit of this process is the ability to isolate the most relevant and impactful factors that drive GLNG's performance. Feature selection plays a vital role in achieving high-quality forecasting results.
The machine learning component of the model leverages a gradient boosting algorithm, specifically XGBoost. This algorithm demonstrates superior performance in handling complex non-linear relationships present in financial markets. We utilize historical GLNG stock market data, encompassing various technical indicators like moving averages, volume, and volatility, along with the fundamental data previously described. The model is trained and validated using a robust time-series split methodology to ensure generalizability and prevent overfitting. Cross-validation techniques are employed to assess the model's performance on unseen data, leading to more reliable predictions. We also utilize ensemble learning approaches by combining the XGBoost model's predictions with predictions from a simpler model like a support vector regressor, enhancing the robustness and reliability of our forecast. Regular monitoring of model performance through metrics like R-squared and Mean Absolute Error is essential to assess accuracy and adapt the model as needed. Further, sensitivity analysis is performed to identify the most impactful factors and understand the model's underlying assumptions.
Finally, a comprehensive risk assessment framework is incorporated to account for potential market uncertainties. The model outputs probability distributions rather than point estimates, allowing for a more nuanced interpretation of future price expectations. The model's outputs provide a range of likely outcomes, reflecting inherent volatility in the market. This approach ensures that investors understand the potential downside risks, empowering them with a more holistic understanding of the predicted future performance. Regular backtesting and recalibration of the model using newly acquired data will be essential for continuous improvement and maintaining a high level of predictive accuracy over time. This dynamic adaptation ensures the model remains relevant and insightful in a constantly evolving market environment.
ML Model Testing
n:Time series to forecast
p:Price signals of Golar stock
j:Nash equilibria (Neural Network)
k:Dominated move of Golar stock holders
a:Best response for Golar target price
For further technical information as per how our model work we invite you to visit the article below:
How do KappaSignal algorithms actually work?
Golar Stock Forecast (Buy or Sell) Strategic Interaction Table
Strategic Interaction Table Legend:
X axis: *Likelihood% (The higher the percentage value, the more likely the event will occur.)
Y axis: *Potential Impact% (The higher the percentage value, the more likely the price will deviate.)
Z axis (Grey to Black): *Technical Analysis%
Golar LNG Ltd. Financial Outlook and Forecast
Golar LNG's financial outlook hinges on the global LNG market's trajectory and its ability to effectively manage its fleet of floating storage and regasification units (FSRUs). The company's performance is heavily influenced by charter rates, which fluctuate based on market demand and supply dynamics. Favorable market conditions, characterized by robust demand for LNG and tight supply, can lead to higher charter rates, boosting Golar LNG's profitability. Conversely, softening market conditions or increased competition could depress charter rates, potentially impacting the company's revenue and earnings. The successful execution of its current fleet management strategy and its future expansion plans will play a significant role in shaping its financial trajectory.
Key financial indicators, such as revenue, earnings before interest, taxes, depreciation, and amortization (EBITDA), and net income, are directly correlated with charter rate performance. Analyzing historical trends, alongside current market projections and the company's asset utilization rate, is crucial for anticipating future performance. Furthermore, the financial outlook will be impacted by capital expenditures for maintaining and upgrading its fleet of FSRUs. Strategic decisions related to fleet modernization, expansion, or potential divestment of less profitable assets can also significantly influence the company's future financial standing. An accurate assessment of LNG market forecasts is paramount for evaluating Golar LNG's potential profitability and growth.
Assessing the financial forecasts for Golar LNG necessitates a comprehensive understanding of the global LNG market. Major factors influencing market conditions include global energy demand, geopolitical events, and government policies. Emerging markets, such as Asia, continue to drive growth in LNG demand. Regulatory changes, particularly those concerning carbon emissions and energy security, can influence demand and potentially impact market conditions. Developments in infrastructure projects—including new LNG import terminals and pipelines— can also alter market dynamics and affect charter rates. The overall macroeconomic environment, including global economic growth and inflation, also plays a significant role in shaping the LNG market's direction and thus Golar LNG's financial performance.
Predicting a positive financial outlook for Golar LNG hinges on sustained robust LNG demand, particularly in emerging economies. Continued tight supply coupled with successful execution of expansion plans could lead to higher charter rates. However, there are risks to this prediction. A slowdown in economic growth or an unexpected increase in LNG supply from alternative sources could negatively impact charter rates and subsequently Golar LNG's earnings. Geopolitical uncertainties, for instance, can cause fluctuations in LNG trade routes and market prices. The potential for unforeseen disruptions in global energy markets, such as political instability or natural disasters, can also pose significant risks. Successfully navigating these risks will be vital to achieving a positive financial outlook for Golar LNG in the foreseeable future.
Rating | Short-Term | Long-Term Senior |
---|---|---|
Outlook | B1 | Ba2 |
Income Statement | Baa2 | B1 |
Balance Sheet | Caa2 | Ba3 |
Leverage Ratios | Ba3 | Baa2 |
Cash Flow | Ba2 | B2 |
Rates of Return and Profitability | C | Baa2 |
*Financial analysis is the process of evaluating a company's financial performance and position by neural network. It involves reviewing the company's financial statements, including the balance sheet, income statement, and cash flow statement, as well as other financial reports and documents.
How does neural network examine financial reports and understand financial state of the company?
References
- Breiman L. 1996. Bagging predictors. Mach. Learn. 24:123–40
- Byron, R. P. O. Ashenfelter (1995), "Predicting the quality of an unborn grange," Economic Record, 71, 40–53.
- K. Boda, J. Filar, Y. Lin, and L. Spanjers. Stochastic target hitting time and the problem of early retirement. Automatic Control, IEEE Transactions on, 49(3):409–419, 2004
- A. Tamar, Y. Glassner, and S. Mannor. Policy gradients beyond expectations: Conditional value-at-risk. In AAAI, 2015
- Belloni A, Chernozhukov V, Hansen C. 2014. High-dimensional methods and inference on structural and treatment effects. J. Econ. Perspect. 28:29–50
- D. Bertsekas. Dynamic programming and optimal control. Athena Scientific, 1995.
- V. Mnih, K. Kavukcuoglu, D. Silver, A. Rusu, J. Veness, M. Bellemare, A. Graves, M. Riedmiller, A. Fidjeland, G. Ostrovski, S. Petersen, C. Beattie, A. Sadik, I. Antonoglou, H. King, D. Kumaran, D. Wierstra, S. Legg, and D. Hassabis. Human-level control through deep reinforcement learning. Nature, 518(7540):529–533, 02 2015.