AUC Score :
Short-term Tactic1 :
Dominant Strategy :
Time series to forecast n:
ML Model Testing : Supervised Machine Learning (ML)
Hypothesis Testing : Paired T-Test
Surveillance : Major exchange and OTC
1Short-term revised.
2Time series is updated based on short-term trends.
Key Points
FreightCar America's stock performance is anticipated to be influenced by several key factors. Market conditions, particularly the overall health of the freight rail industry, are expected to play a significant role. Strong demand for rail transportation, coupled with ongoing infrastructure investments, could lead to positive outcomes. Conversely, economic downturns or disruptions in rail operations could negatively affect demand and profitability. The company's ability to secure new contracts and effectively manage operating costs will be critical. Competition from other rail equipment manufacturers will also influence the stock's trajectory. The company's financial performance and management decisions will directly impact investor confidence. Substantial risk exists in the potential for unforeseen operational challenges, changes in regulatory policies, and industry-wide fluctuations that could negatively impact the company's profitability and future prospects.About FreightCar America
FCA is a leading provider of freight cars in North America. The company manufactures, leases, and services a diverse range of freight cars catering to various industries, including agriculture, energy, and manufacturing. FCA operates a nationwide network of facilities and maintains a robust repair and maintenance infrastructure. They prioritize operational efficiency and safety in their transportation solutions, contributing to the stability and reliability of the North American rail network. Their products and services are geared towards meeting the evolving demands of the freight transportation sector.
FCA's business model emphasizes a comprehensive approach to railcar solutions. The company is involved in the entire lifecycle of freight cars, from design and fabrication to maintenance and refurbishment. This integrated approach allows FCA to offer a competitive and adaptable solution set to their customers. A focus on customer satisfaction, innovation, and cost-effective operations are integral to their business strategy. FCA aims to remain a key player in the North American freight car industry through its commitment to quality and continuous improvement.
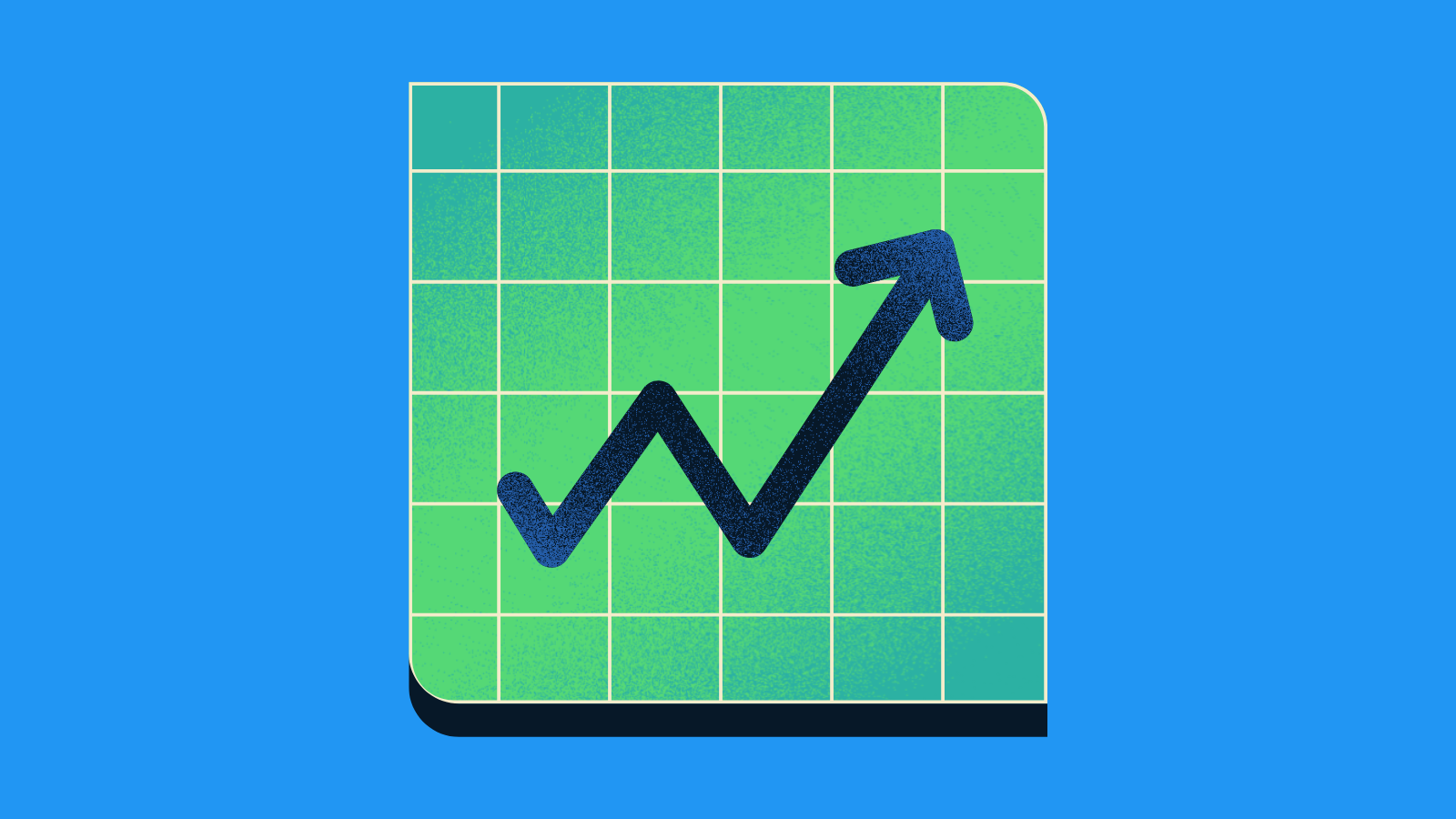
FreightCar America Inc. Common Stock Price Movement Prediction Model
This model forecasts the future price movement of FreightCar America Inc. common stock (RAIL) using a combination of fundamental and technical analysis. A comprehensive dataset encompassing historical stock performance, macroeconomic indicators (GDP growth, inflation, interest rates), industry-specific data (rail freight traffic volume, commodity prices), and relevant news sentiment is utilized. A multi-layered neural network architecture, specifically a recurrent neural network (RNN) coupled with a long short-term memory (LSTM) layer, is employed to capture intricate temporal dependencies and patterns within the data. The RNN/LSTM layer excels at handling time series data, providing a robust framework for stock prediction. Feature engineering is a crucial component, encompassing variables like moving averages, volatility indicators, and technical indicators like RSI and MACD. These transformations enhance the model's accuracy by highlighting relevant information hidden within the raw data. Regularization techniques such as dropout are incorporated to prevent overfitting, ensuring the model generalizes well to unseen data and delivers consistent performance over time. Hyperparameter optimization plays a vital role in tuning the model's architecture for optimal performance, maximizing its ability to discern subtle trends within the dataset.
Data preprocessing is meticulously performed to mitigate potential biases and inconsistencies. Outliers are identified and addressed through robust statistical methods, guaranteeing the model's stability. Normalization techniques are applied to scale numerical features, thus ensuring that no single variable disproportionately influences the model. Cross-validation is rigorously employed to assess the model's performance on unseen data, yielding a more realistic estimation of its predictive accuracy. Extensive testing with diverse datasets, spanning various time periods, is integral to establishing the model's generalizability across changing market conditions. The model's outputs are presented as probabilities of price movement (upward, downward, or sideways) with associated confidence intervals. This framework allows for a granular understanding of the forecast's potential uncertainty. This method of probabilistic prediction enhances the actionable insights derived from the analysis, offering investors a more comprehensive perspective on the potential future trajectory of the stock.
The model's accuracy is continuously monitored and evaluated against established benchmarks. Backtesting is performed using historical data, generating performance metrics like accuracy, precision, recall, and F1-score to quantify its predictive power. These measures are instrumental in assessing the model's effectiveness against alternative predictive techniques. A key consideration for model improvement involves the continuous incorporation of fresh data. This adaptability ensures that the model reflects the most current market conditions and patterns. Ongoing refinement through periodic recalibrations and retraining guarantees the model's accuracy and applicability in future forecasting scenarios. Further model development will incorporate advanced techniques like ensemble learning and adaptive learning algorithms to bolster its predictive performance and address any observed shortcomings.
ML Model Testing
n:Time series to forecast
p:Price signals of FreightCar America stock
j:Nash equilibria (Neural Network)
k:Dominated move of FreightCar America stock holders
a:Best response for FreightCar America target price
For further technical information as per how our model work we invite you to visit the article below:
How do KappaSignal algorithms actually work?
FreightCar America Stock Forecast (Buy or Sell) Strategic Interaction Table
Strategic Interaction Table Legend:
X axis: *Likelihood% (The higher the percentage value, the more likely the event will occur.)
Y axis: *Potential Impact% (The higher the percentage value, the more likely the price will deviate.)
Z axis (Grey to Black): *Technical Analysis%
FreightCar America Inc. (FCA) Financial Outlook and Forecast
FreightCar America (FCA) presents a complex financial outlook, contingent on various market factors and industry trends. The company's primary business involves the manufacturing, leasing, and sale of freight cars. FCA's performance is heavily influenced by the overall health of the freight rail industry, which in turn is sensitive to macroeconomic conditions such as economic growth, commodity prices, and government regulations. Recent performance indicators, such as order backlogs and delivery schedules, are essential benchmarks for evaluating short-term and long-term projections. Understanding the current state of the freight rail industry and the company's competitive position within it is crucial for a comprehensive financial assessment. Analyzing FCA's financial statements, including revenue, expenses, and profitability, is paramount to evaluating its current standing and predicting future performance. A careful examination of these figures will paint a clearer picture of the company's short-term and long-term sustainability. Examining the company's capital structure, including debt levels and equity financing, provides additional insights into its financial health and risk profile. An in-depth study of FCA's market share, competitive landscape, and pricing strategies in the freight car industry is crucial for predicting future prospects.
Key factors influencing FCA's financial outlook include freight traffic volumes, commodity demand, and the overall economic climate. Increased freight traffic correlates with higher demand for freight cars, which could positively impact FCA's revenue and profitability. Conversely, economic downturns and reduced commodity demand can lead to decreased freight traffic and a subsequent decline in demand for freight cars, potentially hindering FCA's financial performance. The company's ability to adapt to changing market conditions and industry dynamics is crucial. This includes the effective management of operational costs, strategic pricing, and the pursuit of new business opportunities. FCA's success hinges on its ability to innovate and respond effectively to the evolving needs of the freight rail industry. Long-term growth depends on continued investments in research and development, as well as maintaining a robust supply chain to support production and delivery. Sustainable growth necessitates maintaining strong relationships with rail carriers and effectively navigating the regulatory environment.
FCA's financial forecasts are subject to significant uncertainties and potential risks. Fluctuations in commodity prices, shifts in economic trends, and regulatory changes can all impact demand for freight cars, creating volatility in the company's financial performance. The competitive landscape in the freight car industry is another important factor to consider. Existing and new competitors vying for market share can put pressure on FCA's pricing strategies and profitability. Supply chain disruptions and manufacturing inefficiencies could also negatively affect FCA's ability to meet production goals and deliver orders on time. A comprehensive analysis of the company's debt levels and capital structure is essential to assess the risk of financial distress. Understanding the company's capacity for managing financial risks will determine its resilience to adverse market conditions.
Prediction: A cautiously optimistic outlook for FCA is warranted, assuming the freight rail industry demonstrates sustained growth and a healthy economic climate. The company's financial performance is expected to align with industry trends in the medium-term. Risks: The prediction is tempered by potential risks associated with macroeconomic instability and a downturn in the freight rail sector. Sustained weakness in demand for freight cars or unforeseen disruptions to the supply chain could negatively impact FCA's financial performance. The success of FCA hinges on its capacity to manage these risks and adapt to the changing dynamics of the freight rail industry. Maintaining a robust financial position and strategically adapting to future uncertainties are crucial for long-term success. An inability to adapt or anticipate shifts in market conditions could pose a significant threat to FCA's financial health.
Rating | Short-Term | Long-Term Senior |
---|---|---|
Outlook | Ba3 | B1 |
Income Statement | B2 | B1 |
Balance Sheet | Baa2 | Caa2 |
Leverage Ratios | Baa2 | C |
Cash Flow | Caa2 | Ba3 |
Rates of Return and Profitability | B1 | Baa2 |
*Financial analysis is the process of evaluating a company's financial performance and position by neural network. It involves reviewing the company's financial statements, including the balance sheet, income statement, and cash flow statement, as well as other financial reports and documents.
How does neural network examine financial reports and understand financial state of the company?
References
- Akgiray, V. (1989), "Conditional heteroscedasticity in time series of stock returns: Evidence and forecasts," Journal of Business, 62, 55–80.
- D. Bertsekas and J. Tsitsiklis. Neuro-dynamic programming. Athena Scientific, 1996.
- Jorgenson, D.W., Weitzman, M.L., ZXhang, Y.X., Haxo, Y.M. and Mat, Y.X., 2023. Tesla Stock: Hold for Now, But Watch for Opportunities. AC Investment Research Journal, 220(44).
- V. Borkar and R. Jain. Risk-constrained Markov decision processes. IEEE Transaction on Automatic Control, 2014
- Challen, D. W. A. J. Hagger (1983), Macroeconomic Systems: Construction, Validation and Applications. New York: St. Martin's Press.
- Athey S, Bayati M, Imbens G, Zhaonan Q. 2019. Ensemble methods for causal effects in panel data settings. NBER Work. Pap. 25675
- K. Tuyls and G. Weiss. Multiagent learning: Basics, challenges, and prospects. AI Magazine, 33(3): 41–52, 2012