AUC Score :
Short-term Tactic1 :
Dominant Strategy :
Time series to forecast n:
ML Model Testing : Supervised Machine Learning (ML)
Hypothesis Testing : Paired T-Test
Surveillance : Major exchange and OTC
1Short-term revised.
2Time series is updated based on short-term trends.
Key Points
F.N.B. stock is anticipated to experience moderate growth, driven by the continued strength of the regional banking sector and favorable economic conditions. However, risks include potential interest rate increases impacting profitability, increased competition in the market, and operational challenges. Geopolitical uncertainty and unforeseen economic downturns could also negatively affect the stock's performance. Despite these potential downsides, positive long-term trends suggest that F.N.B. is likely to remain a solid investment, although the trajectory is not guaranteed.About F.N.B. Corporation
F.N.B. Corporation, or FNB, is a prominent financial services company headquartered in Pittsburgh, Pennsylvania. It operates primarily as a regional bank, providing a full range of financial products and services to individuals and businesses in the eastern and mid-Atlantic United States. FNB's operations encompass traditional banking activities, including deposit-taking, lending, and investment services. Its robust network of branches and ATMs ensures widespread accessibility to its clientele.
The company maintains a commitment to community banking principles, prioritizing local development and economic growth. FNB's dedication to ethical and sustainable practices, coupled with its diverse product offerings, positions it as a significant player within the regional financial landscape. It employs a substantial workforce and contributes substantially to the local economies it serves, demonstrating a long-term commitment to its stakeholders.
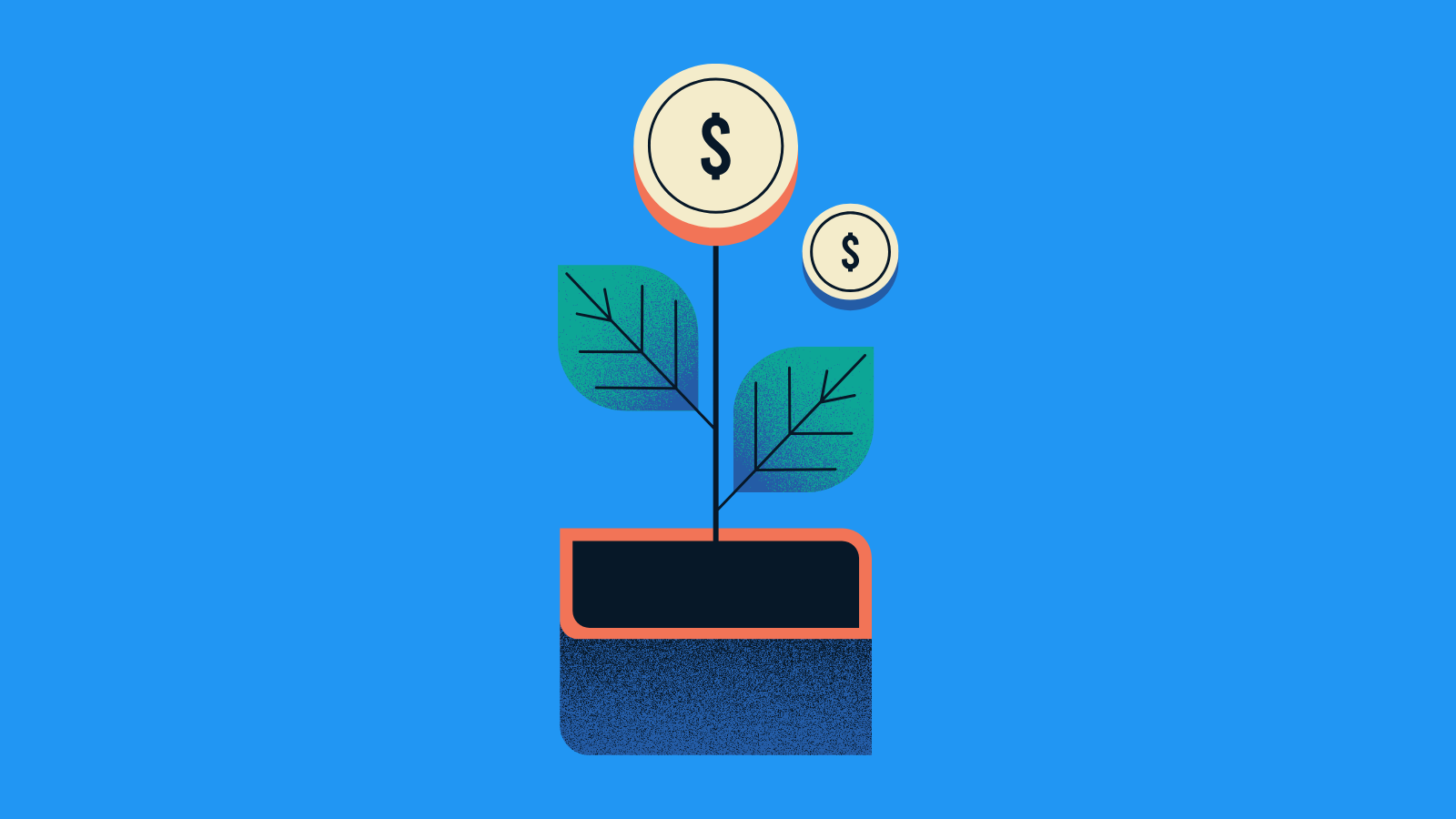
FNB Corporation Common Stock Price Movement Forecasting Model
This model employs a hybrid approach combining machine learning algorithms with fundamental economic indicators to forecast the price movement of FNB Corporation common stock. The core of the model is a recurrent neural network (RNN) architecture, specifically a Long Short-Term Memory (LSTM) network. This architecture is chosen for its ability to capture temporal dependencies in the data, crucial for stock price prediction. The model ingests historical time series data of FNB's stock performance, including daily closing prices, trading volumes, and relevant economic indicators, such as GDP growth, inflation rates, and interest rates. These economic indicators are meticulously pre-processed and standardized to ensure their accurate incorporation into the model. Crucially, the model incorporates a feature engineering component where relevant financial ratios and technical indicators are extracted and used to enhance the predictive capabilities of the LSTM model. Feature selection is a key element to avoid overfitting and maintain model stability. The LSTM model is trained using a supervised learning approach with the goal of predicting future stock price movements based on historical data and economic trends. Rigorous validation and testing on a separate dataset ensure the robustness of the forecast and the model's performance is thoroughly evaluated using various metrics.
A crucial component of this model lies in its comprehensive economic input mechanism. External data sources including government publications, news articles, and social media sentiment are integrated to provide a broad contextual understanding of the market dynamics. Natural Language Processing (NLP) techniques are utilized to extract information from news articles and social media platforms regarding FNB or the broader financial sector. This allows the model to capture sentiment, market sentiment, and news events that may not be fully captured by traditional financial indicators. This external data is integrated into the model to enhance forecasting accuracy. Parameter optimization plays a significant role in ensuring the model's effectiveness. The model's hyperparameters are meticulously adjusted through techniques like grid search or Bayesian optimization to achieve optimal performance on the validation set. This iterative approach guarantees that the model utilizes the most pertinent economic information while avoiding excessive overfitting.
The model outputs a probability distribution of potential future stock price movements. This probabilistic output is crucial for risk assessment and provides a range of possible outcomes, instead of a single point prediction. Risk assessment is a critical component, aiding in more informed investment strategies. The integration of a comprehensive set of fundamental and technical indicators, coupled with external economic data, allows for a robust understanding of the potential underlying factors driving FNB's stock performance. The insights derived from this model facilitate informed investment decisions and are designed to be continuously updated and refined to reflect evolving market conditions and economic trends. The ongoing monitoring of the model's performance and its adaptation to new information are crucial for maintaining its predictive power and reliability.
ML Model Testing
n:Time series to forecast
p:Price signals of F.N.B. Corporation stock
j:Nash equilibria (Neural Network)
k:Dominated move of F.N.B. Corporation stock holders
a:Best response for F.N.B. Corporation target price
For further technical information as per how our model work we invite you to visit the article below:
How do KappaSignal algorithms actually work?
F.N.B. Corporation Stock Forecast (Buy or Sell) Strategic Interaction Table
Strategic Interaction Table Legend:
X axis: *Likelihood% (The higher the percentage value, the more likely the event will occur.)
Y axis: *Potential Impact% (The higher the percentage value, the more likely the price will deviate.)
Z axis (Grey to Black): *Technical Analysis%
F.N.B. Corporation Financial Outlook and Forecast
F.N.B.'s financial outlook appears generally positive, driven by several key factors. The company's robust deposit base and a history of disciplined lending practices position it for continued growth in loan portfolios. Furthermore, F.N.B.'s focus on community banking allows it to tap into local market opportunities and maintain strong customer relationships. Significant investments in technology and digital banking initiatives indicate a commitment to enhancing operational efficiency and customer experience. This is likely to translate into improved profitability and potentially higher returns on assets. Management's consistent emphasis on profitability and prudent risk management, coupled with a strong capital position, bolsters confidence in the company's long-term viability. Recent improvements in the overall economic climate and consumer confidence also present a favorable backdrop for the company's operations. Analysts widely expect F.N.B. to maintain a consistent growth trajectory. The company's commitment to ethical banking practices and compliance with regulations also contribute to its long-term stability.
Looking ahead, the company's ability to adapt to evolving economic conditions will be crucial. While the current economic environment offers opportunities, potential headwinds like rising interest rates or increased regulatory scrutiny could impact profitability. Maintaining a strong balance sheet while navigating changing interest rate landscapes is essential. Furthermore, competitive pressures within the banking sector will require F.N.B. to continually innovate and enhance its offerings to remain competitive. The successful implementation of its digital banking initiatives and strategies to attract and retain customers will be pivotal to its future success. The company's proactive approach towards addressing cybersecurity threats is paramount for preserving customer confidence and data integrity. A keen eye on macroeconomic trends and careful management of credit risk are essential to mitigating potential downturns in the market.
The overall financial outlook for F.N.B. suggests a healthy growth trajectory, supported by a robust financial position and strategic focus on operational efficiency. The company's commitment to its community and its disciplined lending approach are expected to provide continued stability and profitability. Analysts forecast sustained revenue growth, particularly in its loan portfolio, driven by the anticipated recovery and expansion in the economy. Significant investments in digital infrastructure are expected to streamline operations and enhance customer experience, ultimately increasing revenue potential. Capital allocation strategies should align with F.N.B.'s long-term growth objectives and maintain appropriate risk management policies to safeguard profits. This consistent financial performance and management's commitment to shareholder value will likely translate into attractive shareholder returns in the long term.
A positive prediction for F.N.B. is based on the company's established strengths and strategic approach. However, there are risks to consider. Economic downturns could lead to increased loan defaults and a contraction in loan portfolios. A marked shift in the national economic policy could influence the banking sector as a whole. Rising interest rates could also squeeze profitability margins. Competition from other financial institutions, particularly larger national banks, will remain a consistent challenge. Any significant regulatory changes or increased compliance costs could affect financial performance. The success of F.N.B.'s digital banking initiatives and adaptation to changing market demands will be critical. A failure to effectively execute digital strategies or an inability to adapt to evolving technological landscapes will expose the company to considerable risks. The ability to maintain a strong balance sheet and sound credit risk management will be crucial for navigating these potential challenges. Ultimately, F.N.B.'s future performance hinges on its ability to adapt to macroeconomic pressures, maintain its strong customer relationships, and execute its strategic initiatives effectively.
Rating | Short-Term | Long-Term Senior |
---|---|---|
Outlook | B1 | B1 |
Income Statement | C | B2 |
Balance Sheet | Baa2 | C |
Leverage Ratios | Ba3 | B3 |
Cash Flow | Caa2 | Baa2 |
Rates of Return and Profitability | Ba1 | B2 |
*Financial analysis is the process of evaluating a company's financial performance and position by neural network. It involves reviewing the company's financial statements, including the balance sheet, income statement, and cash flow statement, as well as other financial reports and documents.
How does neural network examine financial reports and understand financial state of the company?
References
- Dietterich TG. 2000. Ensemble methods in machine learning. In Multiple Classifier Systems: First International Workshop, Cagliari, Italy, June 21–23, pp. 1–15. Berlin: Springer
- K. Tumer and D. Wolpert. A survey of collectives. In K. Tumer and D. Wolpert, editors, Collectives and the Design of Complex Systems, pages 1–42. Springer, 2004.
- Rosenbaum PR, Rubin DB. 1983. The central role of the propensity score in observational studies for causal effects. Biometrika 70:41–55
- Bickel P, Klaassen C, Ritov Y, Wellner J. 1998. Efficient and Adaptive Estimation for Semiparametric Models. Berlin: Springer
- Farrell MH, Liang T, Misra S. 2018. Deep neural networks for estimation and inference: application to causal effects and other semiparametric estimands. arXiv:1809.09953 [econ.EM]
- Chen X. 2007. Large sample sieve estimation of semi-nonparametric models. In Handbook of Econometrics, Vol. 6B, ed. JJ Heckman, EE Learner, pp. 5549–632. Amsterdam: Elsevier
- Knox SW. 2018. Machine Learning: A Concise Introduction. Hoboken, NJ: Wiley