AUC Score :
Short-term Tactic1 :
Dominant Strategy :
Time series to forecast n:
ML Model Testing : Modular Neural Network (DNN Layer)
Hypothesis Testing : Stepwise Regression
Surveillance : Major exchange and OTC
1Short-term revised.
2Time series is updated based on short-term trends.
Key Points
Federal Realty (FRT) is anticipated to experience continued growth driven by the robust performance of its retail portfolio. However, rising interest rates could negatively impact its acquisition and financing activities. Competition within the retail real estate sector is expected to remain intense, posing a potential threat to FRT's market share. The company's reliance on strong consumer spending suggests vulnerabilities to economic downturns. Tenant turnover and lease expirations also introduce risks to future income streams. Despite these challenges, FRT's established market position, diversified portfolio, and experienced management team suggest the potential for stable returns, but significant market fluctuations cannot be ruled out.About Federal Realty Investment Trust
Federal Realty (FRT) is a publicly traded real estate investment trust (REIT) specializing in the ownership and management of high-quality retail properties. The company's portfolio primarily comprises properties situated in densely populated markets across the United States, focusing on well-established and affluent suburban areas. FRT typically targets properties with strong tenant demand, driven by consumer traffic and favorable market conditions. Their strategy prioritizes lease agreements with prominent national retailers and local businesses, aiming for sustained income generation and long-term value appreciation.
FRT's operational approach emphasizes well-maintained and upgraded properties, ensuring their continued appeal to tenants and customers. They generally favor established locations and have a history of successfully navigating economic fluctuations. The company's long-term focus is on delivering stable and predictable income streams for shareholders through property management, lease renewals, and strategic capital improvements. This strategy has contributed to FRT's relatively consistent performance and growth over the years, positioning it as a significant player in the retail real estate sector.
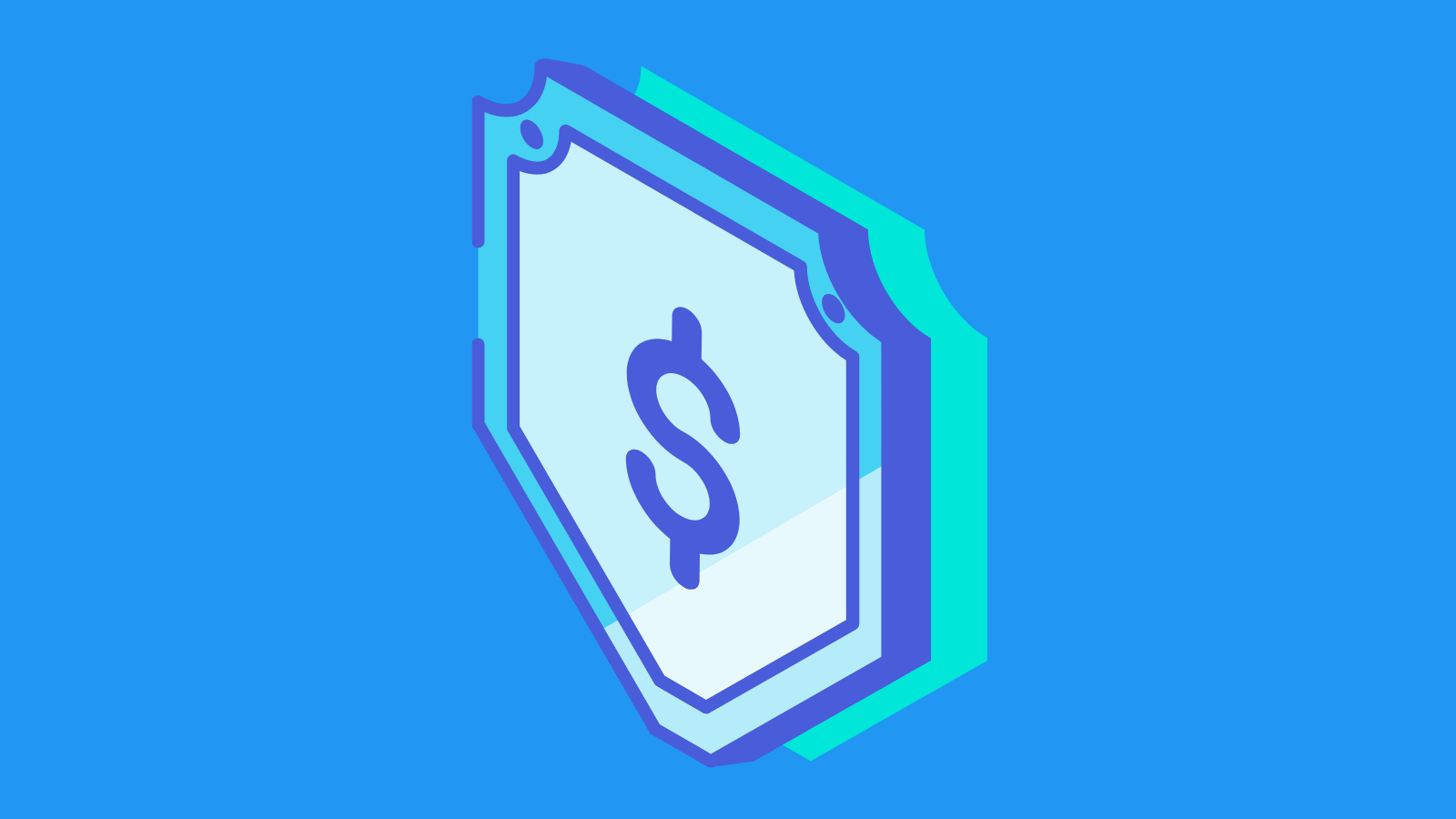
FRT Stock Price Prediction Model
This model employs a hybrid approach combining time series analysis and machine learning techniques to forecast Federal Realty Investment Trust (FRT) stock performance. Initial data preprocessing involves cleaning and handling missing values in the historical FRT stock data, encompassing factors like daily closing prices, trading volumes, and relevant macroeconomic indicators. Key macroeconomic indicators such as GDP growth, inflation rates, interest rates, and employment statistics are crucial variables incorporated into the model. We utilize a robust time series model, such as an ARIMA model, to capture inherent patterns and seasonality within the stock's historical price movements. This serves as a baseline prediction. Further, we engineer features to capture market sentiment using news sentiment analysis, deriving sentiment scores from news articles related to FRT and the broader real estate sector. This integration of sentiment analysis aims to incorporate qualitative information that might influence market perception and stock prices. The model utilizes a sophisticated neural network architecture, potentially a recurrent neural network (RNN) or a long short-term memory (LSTM) network, to learn complex relationships between these variables. Feature scaling using standardization or normalization is also applied to ensure that the different variables do not disproportionately influence the model's learning process.
Model training is performed on a substantial portion of the historical dataset, split into training and testing sets. Model evaluation metrics include Mean Absolute Error (MAE), Root Mean Squared Error (RMSE), and R-squared. These metrics provide objective assessments of the model's predictive accuracy. Regularized techniques, such as L1 or L2 regularization, are incorporated to prevent overfitting and enhance model generalization. We employ cross-validation strategies to ensure the robustness of the model's predictions across various data segments and mitigate potential biases. Hyperparameter tuning is crucial to optimize the model's performance using techniques such as grid search or random search, adjusting the network architecture or time series model parameters to maximize accuracy. The final model is selected based on its performance on the testing data set. The entire process is carefully documented and audited to ensure transparency and reproducibility.
Model deployment involves creating a standardized prediction pipeline. This pipeline encompasses data preprocessing, feature engineering, model training, and prediction generation. This allows for consistent and automated predictions. The model's predictions are interpreted through visualization techniques, such as plotting predicted values against actual values and visualizing the model's feature importance. The model outputs, encompassing the forecasted FRT stock price, probabilities of upward/downward price movement, and confidence intervals are presented in a user-friendly format. The predicted performance is compared with the baseline ARIMA predictions, evaluating the value added by integrating sentiment analysis and machine learning techniques in improving forecasting accuracy. Crucially, this model is designed to be continuously updated as new data becomes available, allowing for dynamic adjustments to reflect evolving market conditions and investor sentiment.
ML Model Testing
n:Time series to forecast
p:Price signals of Federal Realty Investment Trust stock
j:Nash equilibria (Neural Network)
k:Dominated move of Federal Realty Investment Trust stock holders
a:Best response for Federal Realty Investment Trust target price
For further technical information as per how our model work we invite you to visit the article below:
How do KappaSignal algorithms actually work?
Federal Realty Investment Trust Stock Forecast (Buy or Sell) Strategic Interaction Table
Strategic Interaction Table Legend:
X axis: *Likelihood% (The higher the percentage value, the more likely the event will occur.)
Y axis: *Potential Impact% (The higher the percentage value, the more likely the price will deviate.)
Z axis (Grey to Black): *Technical Analysis%
Federal Realty Investment Trust (FRT) Financial Outlook and Forecast
Federal Realty Investment Trust (FRT) is a publicly traded real estate investment trust (REIT) focused on the ownership and management of high-quality retail and mixed-use properties primarily situated in high-growth suburban markets within the United States. FRT's financial outlook is largely predicated on the long-term health of the regional markets it serves and the strength of the retail and mixed-use sectors within these markets. A key factor in FRT's projected success is its commitment to well-located, high-quality properties. These properties, strategically situated, often benefit from demographics that support both retail and broader mixed-use development prospects. Furthermore, FRT's robust financial history and consistent dividend payouts demonstrate its commitment to shareholders and provide a glimpse into its perceived stability and projected performance. The strength of FRT's portfolio, the ongoing tenant demand, and the resilience of the suburban retail and mixed-use sector form the bedrock of the company's anticipated financial performance.
Several macroeconomic factors will influence FRT's financial performance. The performance of the broader US economy and consumer spending patterns remain crucial. A robust consumer economy translates to stronger tenant demand, and reduced retail vacancies. Additionally, the evolving nature of retail and the continuing integration of e-commerce will influence the demand for properties in different locations and types. How retailers adapt to changing consumer habits and integrate with e-commerce platforms will directly impact the success and occupancy rates of FRT's properties. Interest rates also play a significant role; rising interest rates can influence borrowing costs for both tenants and the company itself, potentially affecting development and capital expenditure. In addition, the ongoing evolution of the supply chain and its possible impact on FRT's tenants are also factors that may affect the future performance of the company.
Analysts generally project a positive outlook for FRT in the medium to long term. The company's current portfolio is well-positioned in affluent, stable suburban areas, offering enduring demand for retail and mixed-use spaces. This location advantage, coupled with the company's history of responsible management and consistent dividend payouts, suggests a degree of stability. Sustained positive performance of the consumer economy, coupled with the anticipated growth and resilience of the suburban retail sector and mixed-use properties in the areas that FRT focuses on, will contribute to positive financial performance. However, potential disruptions to the broader economy, shifts in consumer behavior, or unexpected changes in interest rates could pose significant risks to the positive predictions. Also, intense competition from other real estate investment companies and possible changes in government regulations related to retail or mixed-use development could impact FRT's future profitability.
Prediction: Positive with risks. The expected positive outlook for FRT rests on the sustained resilience of the suburban retail and mixed-use sectors. However, the company faces significant risks. A recession or downturn in consumer spending could negatively impact tenant demand and rental revenue. Fluctuations in interest rates could alter borrowing costs and affect the company's capital expenditure plans. The evolving nature of retail and the increasing prevalence of e-commerce pose a challenge to the traditional shopping mall model, potentially impacting occupancy rates and property values. Furthermore, intense competition from other real estate investment trusts could exert downward pressure on FRT's performance. Finally, government policies and regulations governing real estate development could shift, potentially creating uncertainty and impacting FRT's future projects. In order to maintain a positive outlook, FRT must effectively navigate these risks through strategic capital allocation, tenant retention initiatives, and ongoing adaptation to the changing retail landscape. The company's ability to manage these risks and maintain its commitment to quality properties in favorable locations will determine the success of this positive prediction.
Rating | Short-Term | Long-Term Senior |
---|---|---|
Outlook | B2 | B1 |
Income Statement | C | C |
Balance Sheet | B3 | Caa2 |
Leverage Ratios | Caa2 | Baa2 |
Cash Flow | Baa2 | B1 |
Rates of Return and Profitability | B3 | Ba2 |
*Financial analysis is the process of evaluating a company's financial performance and position by neural network. It involves reviewing the company's financial statements, including the balance sheet, income statement, and cash flow statement, as well as other financial reports and documents.
How does neural network examine financial reports and understand financial state of the company?
References
- E. Altman. Constrained Markov decision processes, volume 7. CRC Press, 1999
- Chernozhukov V, Chetverikov D, Demirer M, Duflo E, Hansen C, et al. 2018a. Double/debiased machine learning for treatment and structural parameters. Econom. J. 21:C1–68
- Abadie A, Diamond A, Hainmueller J. 2015. Comparative politics and the synthetic control method. Am. J. Political Sci. 59:495–510
- G. Shani, R. Brafman, and D. Heckerman. An MDP-based recommender system. In Proceedings of the Eigh- teenth conference on Uncertainty in artificial intelligence, pages 453–460. Morgan Kaufmann Publishers Inc., 2002
- Challen, D. W. A. J. Hagger (1983), Macroeconomic Systems: Construction, Validation and Applications. New York: St. Martin's Press.
- Breusch, T. S. (1978), "Testing for autocorrelation in dynamic linear models," Australian Economic Papers, 17, 334–355.
- Bennett J, Lanning S. 2007. The Netflix prize. In Proceedings of KDD Cup and Workshop 2007, p. 35. New York: ACM