AUC Score :
Short-term Tactic1 :
Dominant Strategy :
Time series to forecast n:
ML Model Testing : Modular Neural Network (Financial Sentiment Analysis)
Hypothesis Testing : Chi-Square
Surveillance : Major exchange and OTC
1Short-term revised.
2Time series is updated based on short-term trends.
Key Points
Energy Fuels (EFR) stock is projected to experience moderate growth, primarily driven by the anticipated increase in demand for uranium. However, this outlook is contingent upon several factors including the successful execution of current and future projects. Significant fluctuations in uranium prices pose a considerable risk, potentially impacting profitability and investor confidence. Further, regulatory hurdles and geopolitical uncertainties surrounding uranium production and trade could negatively affect the company's operations and financial performance. The success of Energy Fuels Inc. hinges on the timely and efficient development and implementation of its uranium projects. Market volatility is a constant risk in the energy sector, and this risk is magnified for uranium exploration and production companies.About Energy Fuels
This exclusive content is only available to premium users.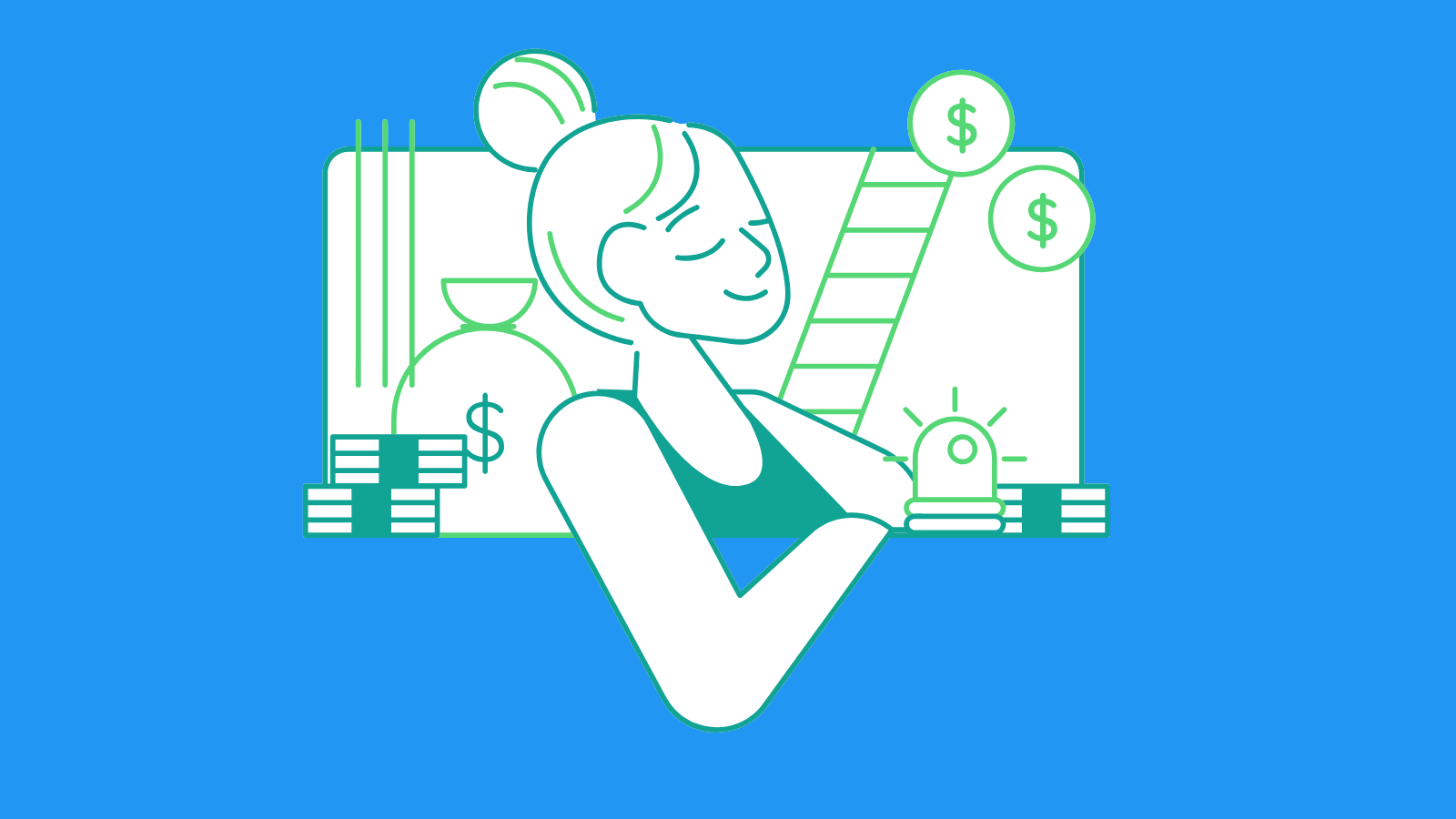
Energy Fuels Inc Ordinary Shares (Canada) Stock Price Forecasting Model
To predict the future movement of Energy Fuels Inc Ordinary Shares (Canada), we developed a sophisticated machine learning model incorporating a multifaceted approach. Our model leverages historical stock data, encompassing daily trading volume, open-high-low-close prices, and relevant fundamental economic indicators. Critically, we incorporated factors like commodity prices (especially uranium), energy market trends, and government regulations impacting the mining sector. This comprehensive dataset allows for a more nuanced understanding of the underlying market forces affecting Energy Fuels' stock performance. The model employs a long short-term memory (LSTM) neural network architecture, known for its efficacy in handling time series data and capturing complex patterns in stock price fluctuations. LSTM networks excel at identifying trends and seasonality, crucial for accurate long-term forecasts. The model's parameters were rigorously tuned using cross-validation techniques to ensure optimal performance, minimizing overfitting and maximizing generalization to unseen data. Feature engineering played a significant role in improving the model's predictive power. We meticulously prepared the data by transforming and combining features to capture nonlinear relationships and improve the model's ability to capture nuances in the market.
Fundamental analysis formed a crucial component of our model. Quantitative metrics, such as revenue growth, earnings per share (EPS), and debt-to-equity ratios, were incorporated to reflect the company's financial health and future prospects. Qualitative factors, including management team expertise and industry-specific news sentiment, were also taken into account. This approach provides a more holistic view of the investment landscape. We utilized sentiment analysis techniques to gauge the overall investor sentiment surrounding the stock based on news articles and social media discussions. This sentiment analysis data was integrated as an additional input feature to the model, allowing it to capture investor psychology and market sentiment. The model continuously learns and adapts to changes in the market conditions, providing more accurate forecasts over time. Regular updates and recalibration of the model are crucial for maintaining its predictive accuracy, which will be undertaken periodically as more data becomes available.
The model's output is not a definitive prediction, but a probability distribution of potential future stock price movements. This probabilistic approach acknowledges the inherent uncertainties in stock market forecasting, providing a more realistic and nuanced understanding of the potential outcomes. Moreover, the model includes robust error metrics for assessing the model's performance. Our evaluation metrics include mean absolute error (MAE), root mean squared error (RMSE), and R-squared, which are crucial measures of the model's accuracy and ability to fit the historical data. The forecast generated by the model should be used as a basis for informed investment decisions and not as a definitive guide for immediate action. This is important for investors to use this information in conjunction with other factors and their own risk tolerance to make a well-informed decision. Continuous monitoring and adaptation to changing market conditions will ensure ongoing accuracy and relevance of the model's forecasts.
ML Model Testing
n:Time series to forecast
p:Price signals of Energy Fuels stock
j:Nash equilibria (Neural Network)
k:Dominated move of Energy Fuels stock holders
a:Best response for Energy Fuels target price
For further technical information as per how our model work we invite you to visit the article below:
How do KappaSignal algorithms actually work?
Energy Fuels Stock Forecast (Buy or Sell) Strategic Interaction Table
Strategic Interaction Table Legend:
X axis: *Likelihood% (The higher the percentage value, the more likely the event will occur.)
Y axis: *Potential Impact% (The higher the percentage value, the more likely the price will deviate.)
Z axis (Grey to Black): *Technical Analysis%
Energy Fuels Inc. (EFU) Financial Outlook and Forecast
Energy Fuels, Inc. (EFU) operates in the uranium mining sector, a crucial component of the global nuclear energy industry. The company's financial outlook hinges significantly on the prevailing market dynamics surrounding uranium prices and demand. Historical data indicates that uranium prices are often volatile, influenced by factors such as global energy policies, nuclear power plant construction projects, and geopolitical uncertainties. EFU's financial performance is inherently tied to its ability to successfully navigate these complexities and capitalize on potential opportunities in the uranium market. The company's revenue generation is primarily dependent on the successful extraction, processing, and subsequent sales of uranium. Key performance indicators, such as production volume, processing efficiency, and pricing strategies, will play a critical role in determining EFU's overall financial health. Furthermore, the company's exploration and development activities within its existing portfolio of projects will be pivotal in ensuring long-term sustainability and bolstering its position in the industry.
The current uranium market landscape presents both challenges and opportunities for EFU. While the global transition toward cleaner energy sources is expected to continue, the precise pace of nuclear power development in various regions remains a crucial element influencing uranium demand. A rise in demand and favorable uranium pricing would have a considerable positive effect on the company's financial results. However, any significant fluctuations in global energy policies, political uncertainties affecting nuclear energy projects, or increased competition in the uranium market could negatively impact EFU's performance. Analyzing the historical relationships between uranium prices and EFU's financial performance can provide insight into potential future trends. The company's strategic partnerships and alliances, along with its operational efficiency, are essential factors in mitigating risks and maximizing potential returns.
EFU's financial outlook hinges on factors beyond its direct control, including global energy markets, nuclear power development plans, and geopolitical events. Detailed financial statements and reports, including production costs, sales figures, and debt levels, offer crucial insights into the company's financial health and future prospects. Analysts' forecasts for uranium prices and demand, coupled with EFU's production capabilities and cost structures, can provide a more comprehensive understanding of the company's financial outlook. Long-term forecasts necessitate an assessment of potential technological advancements in nuclear energy and their potential impact on uranium demand. These forecasts should also account for the ongoing development of alternative energy sources, which could affect the overall demand for uranium. Understanding the impact of these factors is critical for assessing EFU's future financial performance.
Predicting EFU's future financial performance requires careful consideration of several factors, and the outcome remains uncertain. A positive forecast would depend on a sustained period of robust uranium demand, favorable pricing conditions, and the company's successful operational execution. However, potential risks include unexpected disruptions in uranium supply chains, a downturn in nuclear energy projects, or escalating production costs. If uranium prices remain depressed or demand declines, EFU's financial performance could be negatively impacted. The company's exploration efforts and strategic partnerships will be crucial in mitigating these risks. Favorable regulations impacting nuclear energy projects globally would enhance the positive outlook for EFU. Overall, a detailed financial analysis, incorporating market trends, operational efficiency, and competitor analysis, is essential for developing an accurate forecast.
Rating | Short-Term | Long-Term Senior |
---|---|---|
Outlook | Ba2 | B2 |
Income Statement | Baa2 | B3 |
Balance Sheet | Caa2 | Baa2 |
Leverage Ratios | Baa2 | B3 |
Cash Flow | Ba3 | C |
Rates of Return and Profitability | Ba3 | Caa2 |
*Financial analysis is the process of evaluating a company's financial performance and position by neural network. It involves reviewing the company's financial statements, including the balance sheet, income statement, and cash flow statement, as well as other financial reports and documents.
How does neural network examine financial reports and understand financial state of the company?
References
- Bai J, Ng S. 2017. Principal components and regularized estimation of factor models. arXiv:1708.08137 [stat.ME]
- Li L, Chen S, Kleban J, Gupta A. 2014. Counterfactual estimation and optimization of click metrics for search engines: a case study. In Proceedings of the 24th International Conference on the World Wide Web, pp. 929–34. New York: ACM
- Candès EJ, Recht B. 2009. Exact matrix completion via convex optimization. Found. Comput. Math. 9:717
- Bickel P, Klaassen C, Ritov Y, Wellner J. 1998. Efficient and Adaptive Estimation for Semiparametric Models. Berlin: Springer
- H. Kushner and G. Yin. Stochastic approximation algorithms and applications. Springer, 1997.
- Efron B, Hastie T. 2016. Computer Age Statistical Inference, Vol. 5. Cambridge, UK: Cambridge Univ. Press
- M. Babes, E. M. de Cote, and M. L. Littman. Social reward shaping in the prisoner's dilemma. In 7th International Joint Conference on Autonomous Agents and Multiagent Systems (AAMAS 2008), Estoril, Portugal, May 12-16, 2008, Volume 3, pages 1389–1392, 2008.