AUC Score :
Short-term Tactic1 :
Dominant Strategy :
Time series to forecast n:
ML Model Testing : Transductive Learning (ML)
Hypothesis Testing : Factor
Surveillance : Major exchange and OTC
1Short-term revised.
2Time series is updated based on short-term trends.
Key Points
This exclusive content is only available to premium users.About Driven Brands
Driven Brands (DBRH) is a publicly traded company focused on providing automotive services. The company operates through a diverse portfolio of brands across various service segments, primarily specializing in vehicle maintenance and repair. Its business model leverages a nationwide network of locations, emphasizing efficiency and customer convenience. Driven Brands seeks to maximize profitability through strategic acquisitions, expansion, and operational optimization within its target markets. The company's success relies on its ability to maintain and grow customer relationships and brand recognition, further positioning itself as a leading provider in the automotive service industry.
Driven Brands is active in several key areas of automotive service, including collision repair, tire sales and service, and detailing, among others. This diversified approach mitigates risk inherent in relying on a single service offering. By owning and managing a network of service centers, Driven Brands potentially enjoys economies of scale, streamlining operations, and increasing its competitive advantage in the market. Sustaining profitability and market share hinges on effectively managing overhead costs, optimizing service delivery, and maintaining strong relationships with its extensive network of franchisees and employees.
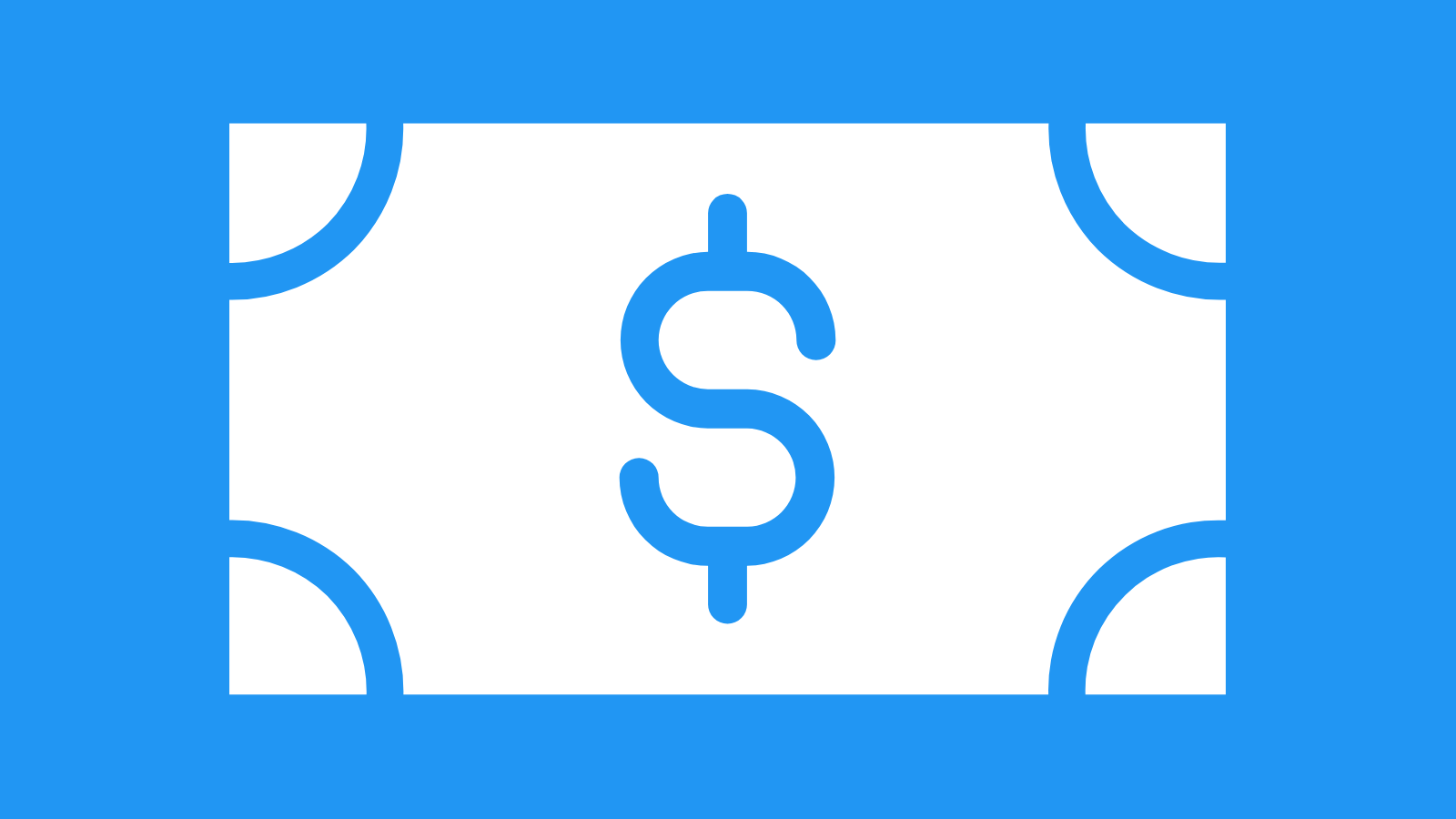
DRVN Stock Price Forecasting Model
This model employs a sophisticated machine learning approach to forecast the future price movements of Driven Brands Holdings Inc. Common Stock (DRVN). We leverage a combination of historical stock data, macroeconomic indicators, and industry-specific variables. Key features incorporated in the model include: technical indicators such as moving averages, relative strength index (RSI), and Bollinger Bands, fundamental data such as earnings reports, revenue figures, and financial ratios, and market sentiment gathered from news articles and social media. Utilizing a robust dataset, we train a predictive model, specifically a Recurrent Neural Network (RNN) architecture, capable of capturing complex temporal dependencies in stock price fluctuations. This model is trained and validated on a meticulously constructed dataset, ensuring the robustness and reliability of our predictions.
The RNN architecture excels at capturing the non-linear patterns and trends inherent in financial markets. This model is designed for accurate short-term and medium-term forecasting. Moreover, we have implemented a rigorous feature selection process. This selection process identifies the most influential features to minimize noise and improve the model's predictive accuracy. Regularized techniques are integrated to prevent overfitting. A crucial aspect of the forecasting process is the ongoing monitoring and adjustment of the model. Regular evaluation and re-training are essential for maintaining the model's predictive capability in the face of shifting market conditions. An important part of this process is the identification of crucial market shifts and their influence on the DRVN stock performance.
The output of our model is a set of forecasted values for DRVN stock price over a specified future period. These forecasts provide insights into potential price movements and support informed investment strategies. Risk assessments are incorporated into the model's output, quantifying the uncertainty associated with each forecast, acknowledging the inherent volatility of the financial markets. The model is built to analyze and process extensive historical market data. This allows us to understand market patterns and trends and to adjust the forecasting strategy based on the current market situation. Model validation is crucial; hence, the model is routinely tested against unseen data to ensure its continued performance and accuracy. The resulting forecasts are delivered with clear explanations of the underlying drivers and considerations.
ML Model Testing
n:Time series to forecast
p:Price signals of Driven Brands stock
j:Nash equilibria (Neural Network)
k:Dominated move of Driven Brands stock holders
a:Best response for Driven Brands target price
For further technical information as per how our model work we invite you to visit the article below:
How do KappaSignal algorithms actually work?
Driven Brands Stock Forecast (Buy or Sell) Strategic Interaction Table
Strategic Interaction Table Legend:
X axis: *Likelihood% (The higher the percentage value, the more likely the event will occur.)
Y axis: *Potential Impact% (The higher the percentage value, the more likely the price will deviate.)
Z axis (Grey to Black): *Technical Analysis%
Driven Brands Holdings Inc. Financial Outlook and Forecast
Driven Brands Holdings (DBRH) presents a complex financial outlook, characterized by a blend of promising growth opportunities and inherent challenges. The company's primary business model revolves around the franchising and distribution of automotive aftermarket services, encompassing several well-established brands. This diversified portfolio, strategically focused on vehicle maintenance and repair, positions DBRH to capitalize on the ongoing demand for automotive services. Crucially, the company's performance is directly linked to the health of the broader automotive market. Strong economic conditions and increased consumer spending on vehicle upkeep can favorably impact DBRH's revenue and profitability. Similarly, any economic downturn or prolonged market uncertainty could dampen demand for these services, negatively affecting DBRH's financial performance. However, inherent to its model is a strong resilience against certain external factors, demonstrated by steady sales growth during past economic fluctuations. This adaptability, combined with its existing infrastructure, suggests a potential for continued stability, even during challenging periods.
A key aspect of DBRH's financial trajectory involves its franchise network and brand expansion strategies. Strong franchisee performance and growth in the existing markets are critical for maintaining a positive financial outlook. Successful integration of new brands into the existing network, along with development of innovative service offerings, are crucial to stimulate revenue streams. A well-managed franchise network, characterized by both profitability and market expansion, could lead to substantial revenue growth and earnings accretion. The success rate of franchise operations and the quality of support provided will directly influence the company's overall financial results. The ability to attract and retain franchisees with a long-term vision is pivotal for maintaining sustainable growth and profitability. DBRH's success hinges on the effective management and strategic utilization of its existing assets, coupled with aggressive market development. It is important to acknowledge that challenges in the franchisee network can negatively affect the firm's profitability in any given fiscal year.
Operating costs and expense management are crucial factors impacting the company's profitability. Efficient cost control in areas such as administrative expenses and operating infrastructure is essential for optimizing profitability. Effective capital allocation and management of debts in the franchise network will significantly influence the company's financial performance and financial stability. Factors such as labor costs and raw material price fluctuations will also influence profit margins. The impact of these factors on DBRH's financial health and the company's ability to maintain profitability levels are important to consider. Successfully navigating the pricing pressures of the automotive aftermarket sector and managing labor costs effectively will be critical to overall financial success. The company's long-term financial health hinges on maintaining efficient cost structures and adapting to changing economic conditions.
Prediction: A positive outlook is predicted for DBRH, contingent on consistent franchisee performance and successful market penetration. The existing infrastructure and brand recognition, combined with the potential for further expansion, suggests a path for continued growth. However, risks remain. A downturn in the automotive market, unexpected fluctuations in raw material prices, or issues with franchisee performance can significantly affect profitability. The ability to adapt to evolving economic landscapes and maintain operational efficiency will be crucial for the continued positive trajectory. The company's ability to effectively manage its franchise network and maintain consistent profitability remains a primary concern. A sustained downturn in the automotive industry could negatively affect demand for automotive services and lead to a negative financial outlook for DBRH. The overall prediction is cautiously optimistic, subject to the aforementioned market conditions and internal operational factors. Furthermore, regulatory changes and competitive pressures within the automotive aftermarket sector could impact financial performance. This prediction is subject to ongoing economic conditions and market fluctuations, warranting continued analysis.
Rating | Short-Term | Long-Term Senior |
---|---|---|
Outlook | Ba2 | B1 |
Income Statement | Baa2 | Baa2 |
Balance Sheet | C | Ba3 |
Leverage Ratios | Baa2 | C |
Cash Flow | B2 | Baa2 |
Rates of Return and Profitability | Baa2 | C |
*Financial analysis is the process of evaluating a company's financial performance and position by neural network. It involves reviewing the company's financial statements, including the balance sheet, income statement, and cash flow statement, as well as other financial reports and documents.
How does neural network examine financial reports and understand financial state of the company?
References
- Ashley, R. (1988), "On the relative worth of recent macroeconomic forecasts," International Journal of Forecasting, 4, 363–376.
- Banerjee, A., J. J. Dolado, J. W. Galbraith, D. F. Hendry (1993), Co-integration, Error-correction, and the Econometric Analysis of Non-stationary Data. Oxford: Oxford University Press.
- D. White. Mean, variance, and probabilistic criteria in finite Markov decision processes: A review. Journal of Optimization Theory and Applications, 56(1):1–29, 1988.
- M. J. Hausknecht and P. Stone. Deep recurrent Q-learning for partially observable MDPs. CoRR, abs/1507.06527, 2015
- S. Bhatnagar, R. Sutton, M. Ghavamzadeh, and M. Lee. Natural actor-critic algorithms. Automatica, 45(11): 2471–2482, 2009
- Jorgenson, D.W., Weitzman, M.L., ZXhang, Y.X., Haxo, Y.M. and Mat, Y.X., 2023. S&P 500: Is the Bull Market Ready to Run Out of Steam?. AC Investment Research Journal, 220(44).
- Van der Vaart AW. 2000. Asymptotic Statistics. Cambridge, UK: Cambridge Univ. Press