AUC Score :
Short-term Tactic1 :
Dominant Strategy :
Time series to forecast n:
ML Model Testing : Multi-Task Learning (ML)
Hypothesis Testing : Multiple Regression
Surveillance : Major exchange and OTC
1Short-term revised.
2Time series is updated based on short-term trends.
Key Points
The Dow Jones U.S. Real Estate Capped index is anticipated to experience moderate growth, driven by sustained economic activity and increasing demand for real estate. However, potential headwinds include rising interest rates, which could dampen investor enthusiasm and slow down the overall market. Geopolitical instability and inflationary pressures could also negatively affect the index's trajectory. Furthermore, shifts in investor sentiment or unanticipated economic downturns could significantly impact performance. The index's future performance will likely be influenced by the interplay of these factors, leading to fluctuations and a degree of uncertainty.About Dow Jones U.S. Real Estate Capped Index
The Dow Jones U.S. Real Estate Capped Index is a market-capitalization-weighted index designed to track the performance of U.S. real estate investment trusts (REITs). It is constructed to reflect the market capitalization of these publicly traded REITs, providing a measure of the overall health and performance of the REIT sector. The index aims to provide a benchmark for investors interested in the real estate market, enabling comparison and analysis of different REIT investment strategies.
Key aspects of this index typically include rigorous selection criteria for constituent REITs, ensuring that the index composition accurately represents the relevant portion of the real estate investment market. It offers a standardized way to assess the collective performance of REITs, facilitating portfolio management and benchmarking. The index is commonly used by analysts, institutional investors, and individual investors alike for evaluating the sector's overall trends, risks, and opportunities.
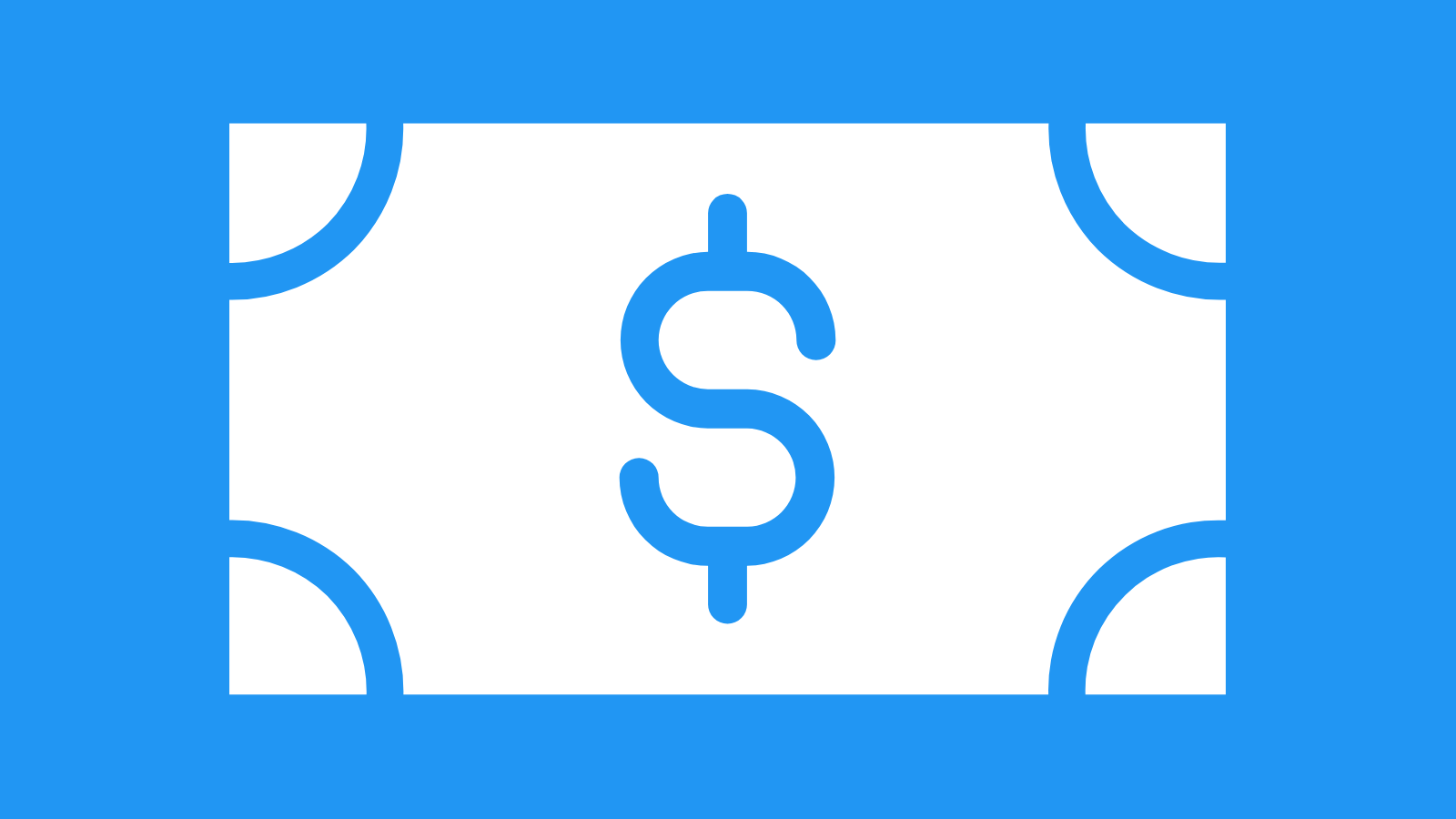
Dow Jones U.S. Real Estate Capped Index Forecast Model
This model for forecasting the Dow Jones U.S. Real Estate Capped index leverages a hybrid approach, combining fundamental economic indicators with technical analysis. Crucially, the model acknowledges the cyclical nature of the real estate market, incorporating lagged values of key variables. We utilize a time series model, specifically an ARIMA model, to capture the historical patterns and trends in the index. Key economic factors like GDP growth, inflation rates, interest rates, and employment data are also included as input features to the model. A comprehensive dataset encompassing these factors over a substantial historical period is essential. Feature engineering will be applied, transforming raw data into meaningful representations for the model. For example, we will construct composite indicators, representing the state of the overall market sentiment. Cross-validation techniques, like K-fold cross-validation, will be used to evaluate the model's performance and prevent overfitting. Moreover, the model incorporates a sensitivity analysis to determine the relative importance of various input features, which is crucial for practical application and interpretation of the results. This comprehensive approach ensures a robust and reliable forecast.
The ARIMA model, coupled with the economic indicators, forms the core of the predictive engine. We anticipate that lags of interest rates and inflation will significantly influence future index performance. The model will be trained on historical data, learning the relationships between these inputs and the index's past behavior. Regular monitoring and retraining of the model will be critical to its effectiveness. Real-time data updates are crucial, as market dynamics can shift rapidly. This approach allows for adaptation and refinement of the model based on changing circumstances and emerging insights. We will also incorporate a range of econometric techniques to analyze the impact of macro-economic policies on the real estate market. This approach addresses the significant uncertainty inherent in forecasting long-term market trends, providing a more robust and insightful analysis. This dynamic approach will capture the intricate interdependencies in the market.
Model evaluation will be rigorous, encompassing diverse metrics like Mean Absolute Error (MAE), Root Mean Squared Error (RMSE), and R-squared values. A thorough comparison of the model's predictions against actual index values will ensure its validity and reliability. Backtesting over various historical periods is essential to evaluate the model's consistency across different economic cycles. The output will be presented in a user-friendly format, providing clear insights into the forecasted trajectory of the Dow Jones U.S. Real Estate Capped index. Regular reporting and transparency in the model's performance metrics will facilitate informed decision-making in the real estate investment sector. This transparent and rigorous approach will enhance the trustworthiness and practical utility of the model.
ML Model Testing
n:Time series to forecast
p:Price signals of Dow Jones U.S. Real Estate Capped index
j:Nash equilibria (Neural Network)
k:Dominated move of Dow Jones U.S. Real Estate Capped index holders
a:Best response for Dow Jones U.S. Real Estate Capped target price
For further technical information as per how our model work we invite you to visit the article below:
How do KappaSignal algorithms actually work?
Dow Jones U.S. Real Estate Capped Index Forecast Strategic Interaction Table
Strategic Interaction Table Legend:
X axis: *Likelihood% (The higher the percentage value, the more likely the event will occur.)
Y axis: *Potential Impact% (The higher the percentage value, the more likely the price will deviate.)
Z axis (Grey to Black): *Technical Analysis%
Rating | Short-Term | Long-Term Senior |
---|---|---|
Outlook | Baa2 | Ba3 |
Income Statement | Baa2 | Baa2 |
Balance Sheet | Baa2 | C |
Leverage Ratios | Baa2 | Ba3 |
Cash Flow | B2 | B1 |
Rates of Return and Profitability | Baa2 | Ba2 |
*An aggregate rating for an index summarizes the overall sentiment towards the companies it includes. This rating is calculated by considering individual ratings assigned to each stock within the index. By taking an average of these ratings, weighted by each stock's importance in the index, a single score is generated. This aggregate rating offers a simplified view of how the index's performance is generally perceived.
How does neural network examine financial reports and understand financial state of the company?
References
- Jorgenson, D.W., Weitzman, M.L., ZXhang, Y.X., Haxo, Y.M. and Mat, Y.X., 2023. Tesla Stock: Hold for Now, But Watch for Opportunities. AC Investment Research Journal, 220(44).
- Breiman L. 2001b. Statistical modeling: the two cultures (with comments and a rejoinder by the author). Stat. Sci. 16:199–231
- Arjovsky M, Bottou L. 2017. Towards principled methods for training generative adversarial networks. arXiv:1701.04862 [stat.ML]
- H. Khalil and J. Grizzle. Nonlinear systems, volume 3. Prentice hall Upper Saddle River, 2002.
- H. Kushner and G. Yin. Stochastic approximation algorithms and applications. Springer, 1997.
- uyer, S. Whiteson, B. Bakker, and N. A. Vlassis. Multiagent reinforcement learning for urban traffic control using coordination graphs. In Machine Learning and Knowledge Discovery in Databases, European Conference, ECML/PKDD 2008, Antwerp, Belgium, September 15-19, 2008, Proceedings, Part I, pages 656–671, 2008.
- Bottou L. 1998. Online learning and stochastic approximations. In On-Line Learning in Neural Networks, ed. D Saad, pp. 9–42. New York: ACM