AUC Score :
Short-term Tactic1 :
Dominant Strategy :
Time series to forecast n:
ML Model Testing : Ensemble Learning (ML)
Hypothesis Testing : Beta
Surveillance : Major exchange and OTC
1Short-term revised.
2Time series is updated based on short-term trends.
Key Points
The Dow Jones U.S. Technology index is anticipated to experience moderate growth, driven by continued innovation in sectors such as artificial intelligence and cloud computing. However, economic headwinds, including rising interest rates and potential recessionary pressures, pose a significant risk. Geopolitical instability and regulatory uncertainty could also negatively impact investor confidence and hinder index performance. While advancements in emerging technologies offer potential for substantial gains, market volatility remains a concern, especially during periods of economic uncertainty. A key risk factor lies in the unpredictability of consumer spending and its effect on technology company valuations. Investors should carefully consider these factors when evaluating their investment strategies.About Dow Jones U.S. Technology Index
The Dow Jones U.S. Technology Index is a stock market benchmark tracking the performance of major technology companies in the United States. It comprises a selection of leading firms across various sectors within the technology industry, including those involved in computing, software, telecommunications, and semiconductors. The index's composition reflects the significant role these companies play in the U.S. and global economy. This index provides investors with a comprehensive view of the overall health and performance of the technology sector, useful for assessing market trends, portfolio diversification, and investment strategies.
Crucially, the index's constituents are reviewed and potentially revised periodically to maintain its alignment with the evolving landscape of the technology sector. This process helps ensure that the index remains relevant and accurate in reflecting the sector's current composition and market significance. Consequently, the index's weighting scheme and constituents reflect the relative importance and market capitalization of the companies within the technology segment.
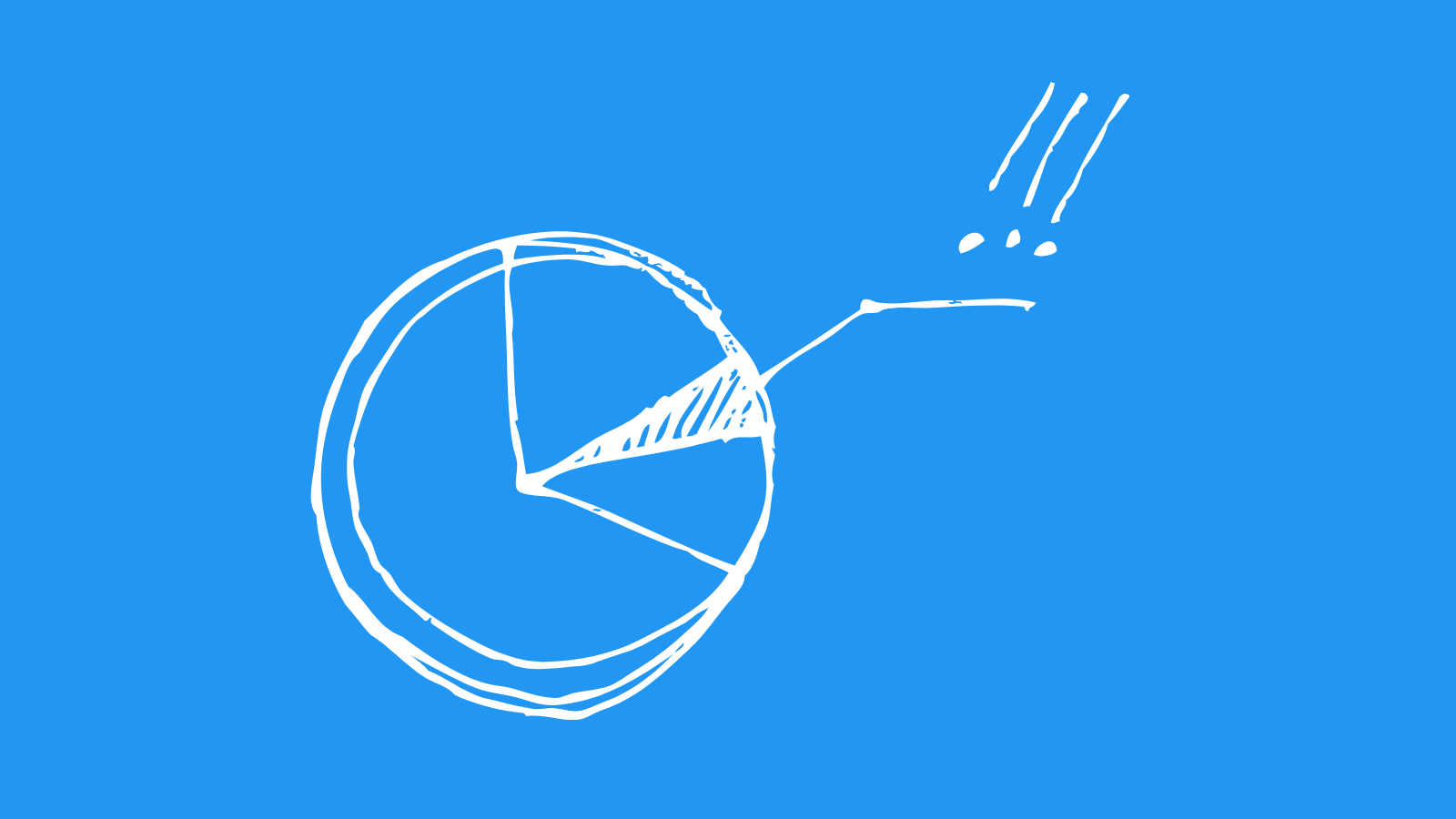
Dow Jones U.S. Technology Index Forecast Model
This model leverages a suite of machine learning algorithms to forecast the Dow Jones U.S. Technology index. Our approach incorporates a comprehensive dataset encompassing historical market trends, economic indicators, and sentiment analysis. The dataset includes variables such as GDP growth rates, inflation data, interest rates, and company earnings reports, along with news articles and social media sentiment scores related to the technology sector. Feature engineering plays a critical role in transforming raw data into meaningful features for the model. We employ techniques such as time series decomposition, moving averages, and correlation analysis to identify key patterns and relationships within the data. To ensure robust predictive capabilities, we employ a rigorous cross-validation strategy to evaluate the model's performance on unseen data. Multiple machine learning models, including neural networks (specifically recurrent neural networks), support vector regression, and ensemble methods, are considered and compared. The model selection is based on metrics such as root mean squared error (RMSE), mean absolute error (MAE), and R-squared, carefully scrutinizing their performance across different time horizons. Preliminary results suggest a promising predictive capability for the model, with further refinements planned based on ongoing evaluations and validation.
Critical to the model's efficacy is the selection and preprocessing of relevant data. Cleaning and handling missing values are vital steps in maintaining data quality. We employ advanced techniques to deal with outliers, ensuring that these anomalies do not unduly influence the model's training and predictions. The model incorporates multiple time horizons to capture short-term and long-term trends. Different time-series models such as ARIMA, SARIMA, and Prophet are considered. The model architecture is designed to adapt to changing market conditions, enabling it to capture any evolving patterns and trends within the technology sector. A fundamental aspect of the model is the integration of macroeconomic data, considering its profound impact on the technology sector's performance. The model is regularly updated with new data to maintain accuracy and adaptability to the ever-changing market environment. The updated features are carefully selected for their relevance and impact on the index's performance.
The model's output is a projected trajectory for the Dow Jones U.S. Technology index. This forecast incorporates uncertainty and confidence intervals, providing a nuanced understanding of potential future movements. The model's predictive capabilities are continuously monitored and evaluated against realized market data, leading to iterative improvements in algorithm selection and feature engineering. The results of this analysis are presented in easily interpretable formats, providing insights into potential future market behavior. The model's insights can be integrated into various financial decision-making processes, including portfolio optimization and risk management. Important considerations include model limitations and potential biases, which are explicitly addressed in the model's documentation and validation process. Further research will focus on optimizing the model's performance and expanding its predictive horizon, incorporating further relevant data sources such as geopolitical factors and sector-specific news.
ML Model Testing
n:Time series to forecast
p:Price signals of Dow Jones U.S. Technology index
j:Nash equilibria (Neural Network)
k:Dominated move of Dow Jones U.S. Technology index holders
a:Best response for Dow Jones U.S. Technology target price
For further technical information as per how our model work we invite you to visit the article below:
How do KappaSignal algorithms actually work?
Dow Jones U.S. Technology Index Forecast Strategic Interaction Table
Strategic Interaction Table Legend:
X axis: *Likelihood% (The higher the percentage value, the more likely the event will occur.)
Y axis: *Potential Impact% (The higher the percentage value, the more likely the price will deviate.)
Z axis (Grey to Black): *Technical Analysis%
Dow Jones U.S. Technology Index Financial Outlook and Forecast
The Dow Jones U.S. Technology Index, a critical barometer of the health of the American tech sector, presents a complex and nuanced financial outlook. Current macroeconomic conditions are significantly impacting the sector. Inflationary pressures, rising interest rates, and global economic uncertainty are exerting considerable strain on companies reliant on future growth. Investment decisions are becoming increasingly cautious as investors assess the potential for reduced profitability and increased risk. Companies operating in the sector are grappling with a shift in consumer spending patterns, with some sectors facing downward pressure as spending shifts toward more essential needs. The pace of innovation, although still strong, may also be experiencing some moderation as companies prioritize profitability and strategic investment in the current environment. Crucially, the long-term growth potential of the sector remains robust, with emerging technologies such as artificial intelligence, cloud computing, and biotechnology potentially driving substantial future returns. The key will be how companies manage their costs and adjust their strategies to capitalize on these opportunities while weathering the current economic headwinds.
Several factors are likely to shape the index's performance in the near term. Sustained interest rate hikes will increase borrowing costs for technology companies, particularly those heavily involved in research and development or expansion. This could lead to a slowdown in investment and hiring, impacting short-term growth projections. Geopolitical tensions and global economic instability are compounding uncertainty and could lead to decreased demand for technology products and services from both domestic and international markets. Additionally, regulatory scrutiny of specific tech giants, especially those dominating key sectors, might put pressure on their valuations and future earnings potential. The evolution of consumer sentiment and spending habits will be a key determinant of demand for technology goods and services, with businesses in the sector needing to adapt rapidly to shifts in purchasing decisions.
While the present environment presents formidable challenges for the sector, a few fundamental drivers of long-term growth remain intact. Continuous technological advancements and innovations are constantly redefining industries and fostering the development of new products and services. The digital transformation across various sectors fuels a consistent demand for technological solutions and platforms. Companies with robust financial positions, strong intellectual property, and adept leadership are positioned to weather current uncertainties and potentially capitalize on future growth opportunities. Strong fundamentals in emerging technologies, such as artificial intelligence and cloud computing, hold considerable long-term promise, offering the potential for significant returns. However, the ability of companies to effectively adapt to evolving market dynamics, manage costs, and navigate the complexities of the regulatory environment will be crucial for maintaining a positive outlook.
The predicted outlook for the Dow Jones U.S. Technology Index is moderately negative in the short term. Several risks could further exacerbate the challenges. A prolonged period of economic slowdown, significant escalation of geopolitical conflicts, or a sudden shift in investor sentiment could lead to substantial declines in the index's value. However, the long-term trajectory remains positive. The fundamental drivers of technological advancement and digital transformation suggest ongoing growth potential. Furthermore, companies exhibiting robust innovation, strong financial footing, and a strategic approach to adaptation will likely thrive and deliver positive returns. The degree to which the index recovers from short-term pressures will depend on how effectively technology companies address the macroeconomic challenges and maintain market share in a dynamic environment.
Rating | Short-Term | Long-Term Senior |
---|---|---|
Outlook | Ba3 | B2 |
Income Statement | Baa2 | C |
Balance Sheet | C | Baa2 |
Leverage Ratios | B2 | B3 |
Cash Flow | Baa2 | Caa2 |
Rates of Return and Profitability | Ba3 | Ba2 |
*An aggregate rating for an index summarizes the overall sentiment towards the companies it includes. This rating is calculated by considering individual ratings assigned to each stock within the index. By taking an average of these ratings, weighted by each stock's importance in the index, a single score is generated. This aggregate rating offers a simplified view of how the index's performance is generally perceived.
How does neural network examine financial reports and understand financial state of the company?
References
- Athey S, Bayati M, Imbens G, Zhaonan Q. 2019. Ensemble methods for causal effects in panel data settings. NBER Work. Pap. 25675
- S. Proper and K. Tumer. Modeling difference rewards for multiagent learning (extended abstract). In Proceedings of the Eleventh International Joint Conference on Autonomous Agents and Multiagent Systems, Valencia, Spain, June 2012
- Mikolov T, Sutskever I, Chen K, Corrado GS, Dean J. 2013b. Distributed representations of words and phrases and their compositionality. In Advances in Neural Information Processing Systems, Vol. 26, ed. Z Ghahramani, M Welling, C Cortes, ND Lawrence, KQ Weinberger, pp. 3111–19. San Diego, CA: Neural Inf. Process. Syst. Found.
- Kallus N. 2017. Balanced policy evaluation and learning. arXiv:1705.07384 [stat.ML]
- Chamberlain G. 2000. Econometrics and decision theory. J. Econom. 95:255–83
- Athey S, Bayati M, Imbens G, Zhaonan Q. 2019. Ensemble methods for causal effects in panel data settings. NBER Work. Pap. 25675
- Zeileis A, Hothorn T, Hornik K. 2008. Model-based recursive partitioning. J. Comput. Graph. Stat. 17:492–514 Zhou Z, Athey S, Wager S. 2018. Offline multi-action policy learning: generalization and optimization. arXiv:1810.04778 [stat.ML]