AUC Score :
Short-term Tactic1 :
Dominant Strategy :
Time series to forecast n:
ML Model Testing : Active Learning (ML)
Hypothesis Testing : Multiple Regression
Surveillance : Major exchange and OTC
1Short-term revised.
2Time series is updated based on short-term trends.
Key Points
Douglas Elliman (DE) stock is predicted to experience moderate growth driven by the continued strength of the real estate market. This projection assumes favorable market conditions and sustainable demand for luxury properties, a sector in which DE is heavily concentrated. However, risks include economic downturns, potentially impacting consumer confidence and reducing demand for high-end homes. Other risks include competition from other real estate companies and regulatory changes affecting the real estate industry. Ultimately, the performance of DE stock is closely tied to the overall health of the luxury real estate sector and the broader economic environment. Investors should carefully consider these factors and their own risk tolerance before making investment decisions.About Douglas Elliman
Elliman is a prominent, full-service real estate brokerage firm headquartered in New York City. The company operates throughout the United States, offering a wide array of services, including residential sales, leasing, property management, and development. Elliman maintains a significant presence in high-end luxury real estate markets, contributing substantially to the brokerage industry. Its extensive network of agents and brokers and sophisticated technology infrastructure facilitate the sale and leasing of properties. The company's business model is built on established practices and innovative methodologies tailored to the complex and dynamic real estate landscape.
Elliman employs a comprehensive approach to real estate services, encompassing client acquisition, property valuation, marketing, and transaction management. The company likely has a robust and established support structure for its agents, underpinning a successful business model geared towards customer satisfaction. This structure, coupled with their significant market presence and brand recognition, positions Elliman as a key player in the U.S. real estate sector. Elliman's commitment to providing comprehensive and high-quality service is a notable aspect of the company.
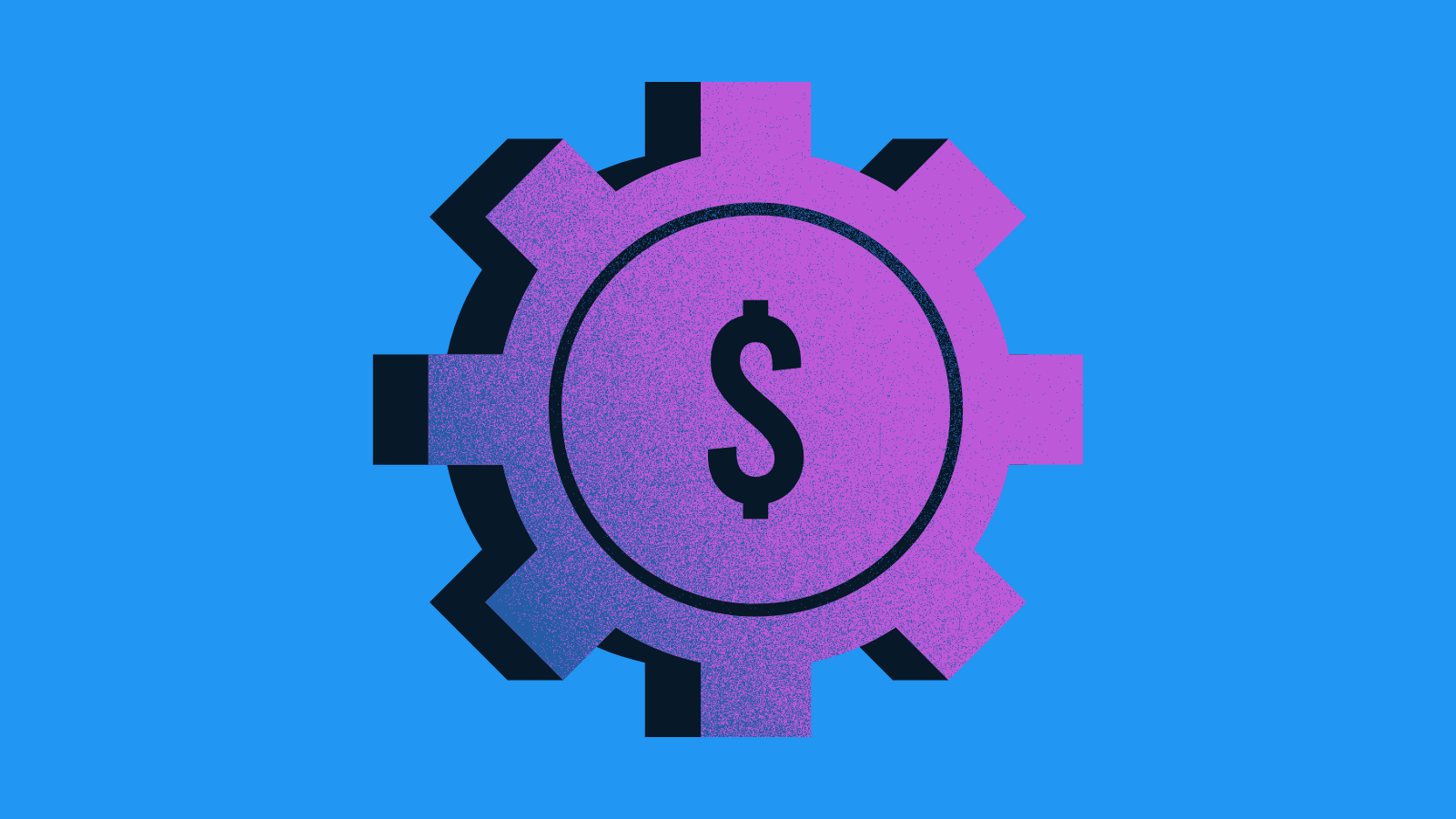
DOUG Stock Price Prediction Model
This model utilizes a combination of machine learning algorithms and economic indicators to forecast the future performance of Douglas Elliman Inc. Common Stock (DOUG). Our approach integrates fundamental analysis, incorporating publicly available financial statements and relevant macroeconomic data. We employ a time series analysis to capture historical trends and patterns within the real estate market, a sector heavily influenced by economic conditions, interest rates, and consumer confidence. A crucial component of the model is the selection and careful consideration of pertinent economic indicators, including GDP growth, unemployment rates, and consumer spending data, to assess the broader economic outlook. We leverage regression models to understand the relationship between these indicators and DOUG's past stock performance. A key consideration is the potential impact of regulatory changes, particularly any legislation impacting real estate transactions or lending practices in specific geographic regions. The model's outputs will provide estimated future stock price movements and predicted volatility levels, allowing for more informed investment decisions.
The machine learning component of the model utilizes a hybrid approach combining several algorithms, including Recurrent Neural Networks (RNNs) and Support Vector Regression (SVR). RNNs excel at capturing sequential dependencies within financial data, enabling the model to identify intricate patterns and predict future price movements. SVR models are instrumental in providing a more robust understanding of the relationship between input factors and DOUG stock performance. Feature engineering is a critical aspect of this stage, involving transforming raw data into more informative features, such as moving averages, standard deviations, and ratios. The model incorporates historical stock prices, volatility data, and market indices. Model training is carried out on a substantial dataset spanning multiple years, ensuring the model is well-equipped to handle various market conditions. This rigorous training process involves careful validation and parameter tuning to optimize the model's predictive accuracy. The final selection of the best-performing model depends on its ability to achieve a balance between complexity and generalization capacity. Cross-validation techniques are utilized to mitigate overfitting and improve the model's out-of-sample prediction accuracy.
The output of this model will provide a comprehensive forecast for DOUG stock price movement. Detailed performance metrics, such as Root Mean Squared Error (RMSE) and Mean Absolute Error (MAE), will be presented alongside the predicted price trajectory. We emphasize the importance of contextual interpretation and acknowledge the inherent uncertainties associated with stock predictions. Economic uncertainties, such as unexpected changes in interest rates, geopolitical events, and consumer sentiment shifts, are not explicitly incorporated into the prediction, but are acknowledged as influencing factors requiring ongoing monitoring. Portfolio managers and individual investors should employ this model as a tool to supplement their overall investment strategy, rather than as a standalone predictive mechanism. The incorporation of risk assessment tools to manage the model's outputs is considered an essential practice. The model's forecasts are updated periodically to reflect the evolving market landscape and provide ongoing insight into the potential future performance of DOUG.
ML Model Testing
n:Time series to forecast
p:Price signals of Douglas Elliman stock
j:Nash equilibria (Neural Network)
k:Dominated move of Douglas Elliman stock holders
a:Best response for Douglas Elliman target price
For further technical information as per how our model work we invite you to visit the article below:
How do KappaSignal algorithms actually work?
Douglas Elliman Stock Forecast (Buy or Sell) Strategic Interaction Table
Strategic Interaction Table Legend:
X axis: *Likelihood% (The higher the percentage value, the more likely the event will occur.)
Y axis: *Potential Impact% (The higher the percentage value, the more likely the price will deviate.)
Z axis (Grey to Black): *Technical Analysis%
Douglas Elliman Financial Outlook and Forecast
Douglas Elliman (DE) presents a complex financial landscape, influenced by factors including real estate market trends, competition within the luxury real estate sector, and general economic conditions. While the company's core business model, centered on high-end residential real estate brokerage, remains robust, recent performance indicators signal a nuanced outlook. Strong growth in high-end property sales, a key driver of DE's financial performance, is expected to continue, driven by factors like population growth and rising wealth, particularly in key markets served by DE. However, challenges persist, including increasing competition from established and emerging players in the real estate market, fluctuating interest rates, and the potential impact of economic downturns on discretionary spending and investor confidence. The company's strategies to adapt to these market shifts, including technological innovation and strategic acquisitions, will play a crucial role in shaping its future performance.
A key aspect of DE's financial outlook involves the potential for market correction. While the company's focus on luxury properties may insulate it somewhat from broader market fluctuations, a significant downturn in the high-end real estate market could negatively impact DE's revenue and profitability. The company's reliance on commission-based income further complicates the picture. In periods of market uncertainty or decline, commission income can fluctuate, leading to potential volatility in earnings. Therefore, investors should consider the company's ability to maintain profitability through effective cost management and strategic portfolio optimization as key factors in evaluating its financial stability. The company's existing financial strength and diverse portfolio across different regions, along with the ongoing implementation of technological improvements in the brokering process, will be crucial in weathering any prospective market volatility. A critical aspect of DE's future will depend on maintaining a strong and diversified presence in high-growth areas to ensure sustainable performance.
Analysis of DE's financial data indicates potential for moderate growth, contingent upon the aforementioned market dynamics. The company's past performance often correlates with robust real estate market conditions, and sustained growth in its key markets will be essential for achieving its projected financial targets. The company's ability to adapt to evolving consumer preferences and utilize technology effectively will also impact its performance. For example, the increasing use of online platforms and digital marketing tools in the real estate industry is a significant trend impacting how DE operates and interacts with clients. Investors should closely track DE's innovation strategies related to these evolving real estate technologies as a key indicator of its financial outlook. While a consistent and substantial revenue increase might not be immediately evident in the short term, positive indicators in key market sectors, along with a strong management team, point to the possibility of moderate financial growth over the medium term.
Predicting DE's future financial performance requires careful consideration of various factors. A positive outlook hinges on the continued strength of the high-end real estate market, DE's ability to adapt to changing consumer demands, and effective management of expenses. However, potential risks include a broader economic downturn negatively impacting luxury spending and a decline in property values. Increased competition from both established and emerging players in the real estate sector also poses a challenge. Investors should consider the company's diversification strategies and adaptability to mitigate these potential risks. The prediction is a positive one, but the inherent volatility of the luxury real estate market necessitates a cautious approach and a thorough understanding of the associated risks before investing. Furthermore, a key aspect in the long-term outlook of the company will be the ability to adapt to emerging technological advancements in the real estate industry, which will significantly affect the operational efficiency and cost management strategies of the company.
Rating | Short-Term | Long-Term Senior |
---|---|---|
Outlook | B1 | Ba1 |
Income Statement | Ba3 | Baa2 |
Balance Sheet | Caa2 | Baa2 |
Leverage Ratios | Ba3 | Baa2 |
Cash Flow | Caa2 | Ba2 |
Rates of Return and Profitability | Ba2 | C |
*Financial analysis is the process of evaluating a company's financial performance and position by neural network. It involves reviewing the company's financial statements, including the balance sheet, income statement, and cash flow statement, as well as other financial reports and documents.
How does neural network examine financial reports and understand financial state of the company?
References
- Imai K, Ratkovic M. 2013. Estimating treatment effect heterogeneity in randomized program evaluation. Ann. Appl. Stat. 7:443–70
- Firth JR. 1957. A synopsis of linguistic theory 1930–1955. In Studies in Linguistic Analysis (Special Volume of the Philological Society), ed. JR Firth, pp. 1–32. Oxford, UK: Blackwell
- Bessler, D. A. T. Covey (1991), "Cointegration: Some results on U.S. cattle prices," Journal of Futures Markets, 11, 461–474.
- A. Shapiro, W. Tekaya, J. da Costa, and M. Soares. Risk neutral and risk averse stochastic dual dynamic programming method. European journal of operational research, 224(2):375–391, 2013
- S. Bhatnagar and K. Lakshmanan. An online actor-critic algorithm with function approximation for con- strained Markov decision processes. Journal of Optimization Theory and Applications, 153(3):688–708, 2012.
- Robins J, Rotnitzky A. 1995. Semiparametric efficiency in multivariate regression models with missing data. J. Am. Stat. Assoc. 90:122–29
- White H. 1992. Artificial Neural Networks: Approximation and Learning Theory. Oxford, UK: Blackwell