AUC Score :
Short-term Tactic1 :
Dominant Strategy :
Time series to forecast n:
ML Model Testing : Modular Neural Network (Market Direction Analysis)
Hypothesis Testing : Lasso Regression
Surveillance : Major exchange and OTC
1Short-term revised.
2Time series is updated based on short-term trends.
Key Points
Donnelley Financial Solutions' future performance hinges on the evolving economic landscape and its ability to adapt to changing client needs. Sustained growth in the financial services sector is crucial for continued revenue expansion. Strong management, strategic acquisitions, and successful implementation of technological advancements will be critical for navigating challenges and capitalizing on opportunities. However, intense competition in the financial technology arena presents a significant risk. Economic downturns could lead to reduced demand for financial products and services, impacting the company's revenue stream. Also, the effectiveness of cost-cutting strategies and operational efficiencies will determine the company's profitability in a dynamic market. Finally, regulatory changes in the financial services industry could introduce unforeseen challenges.About Donnelley Financial Solutions
Donnelley Financial Solutions, a leading provider of financial information and services, offers a wide range of solutions to institutional investors, corporations, and government entities. The company focuses on data analytics, financial reporting, and regulatory compliance. Their products and services encompass diverse needs within the financial industry, leveraging a deep understanding of market trends and regulatory requirements. Donnelley Financial Solutions plays a key role in supporting the financial ecosystem, facilitating informed decision-making for clients.
The company's operations likely involve sophisticated data management, processing, and distribution technologies. Their client base likely includes a spectrum of institutions with varying financial needs, from large investment firms to regulatory bodies. Donnelley Financial Solutions' success hinges on staying abreast of evolving market dynamics and regulatory changes, enabling them to adapt their offerings and maintain their market position.
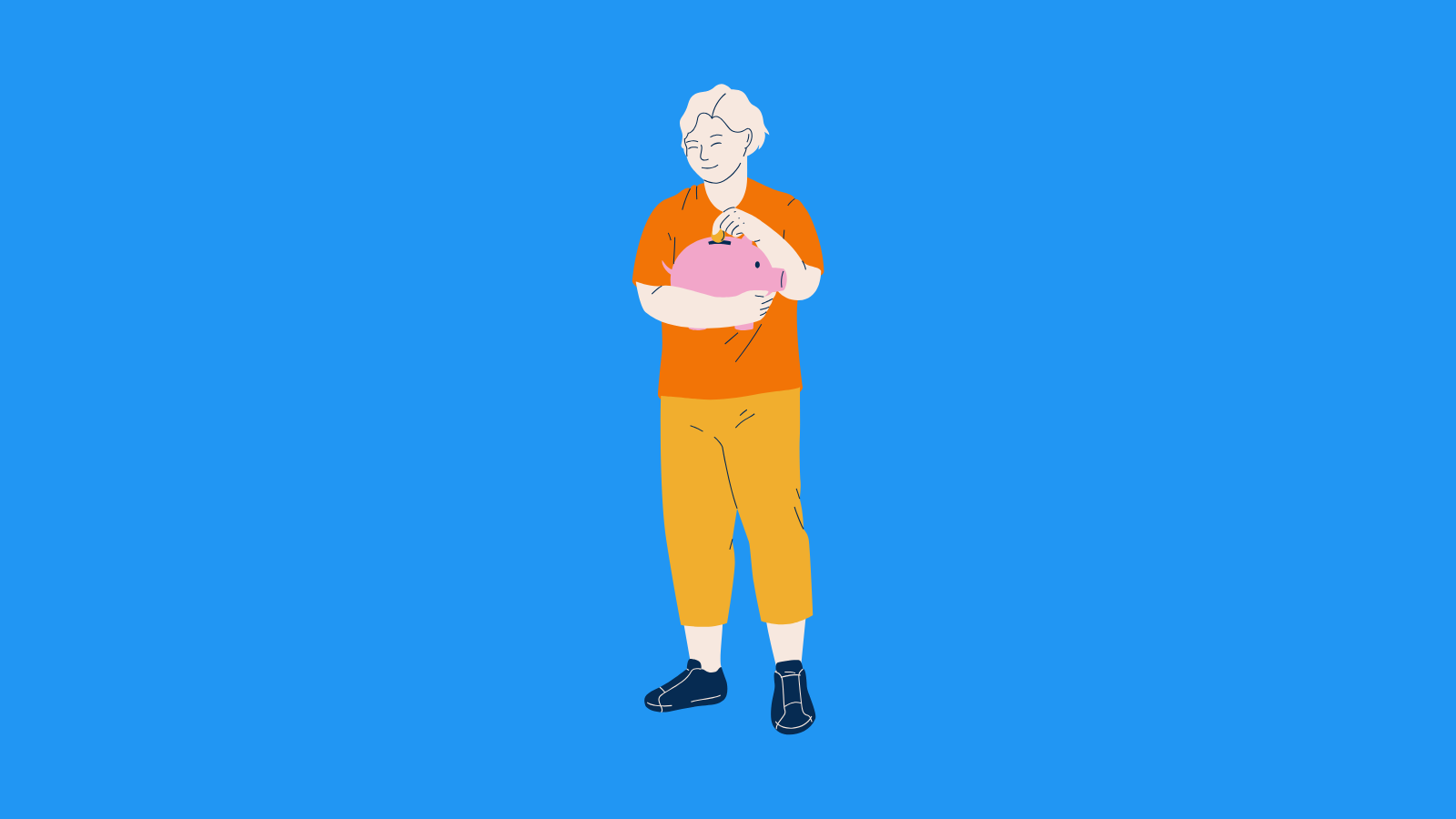
DFIN Stock Price Prediction Model
This model utilizes a hybrid approach combining technical analysis and fundamental data to forecast the future price movements of Donnelley Financial Solutions Inc. (DFIN) common stock. The technical analysis component leverages historical price data, volume, and trading patterns using a Recurrent Neural Network (RNN) architecture specifically designed to capture complex temporal dependencies. The RNN model is trained on a dataset encompassing various technical indicators such as moving averages, relative strength index (RSI), and Bollinger Bands. Features are engineered to represent price momentum, volatility, and potential support/resistance levels. This portion of the model allows for capturing short-term and medium-term trends, anticipating potential reversals, and identifying possible breakout points. Crucially, the model incorporates a robust feature engineering process to control overfitting and improve generalizability.
Complementing the technical analysis, fundamental data, such as earnings reports, revenue projections, and industry-wide macroeconomic trends, are incorporated. This fundamental data is pre-processed and transformed into relevant features for the model. A Support Vector Regression (SVR) model is employed to assess the long-term impact of these factors on the stock's price. The SVR model, known for its robustness in handling non-linear relationships, helps capture the fundamental drivers impacting the stock's intrinsic value. The fundamental component acts as a contrarian signal, providing insights into long-term price movements that may not be evident in the short-term technical analysis. This approach aims to provide a more holistic and nuanced prediction by integrating both short-term market dynamics and long-term underlying value.
The final prediction is generated by combining the outputs of the RNN and SVR models. A weighted average is employed, giving greater weight to the SVR model's prediction when the fundamental data suggests a significant shift in the company's long-term outlook. This hybrid approach enhances the model's accuracy by leveraging the strengths of both short-term technical and long-term fundamental analysis. Cross-validation techniques are implemented throughout the model development to ensure robustness and reliable performance. Regular backtesting and retraining of the model against historical data are crucial for maintaining its efficacy. Continuous monitoring of new market conditions and adjustments to the model parameters ensure timely adaptation to evolving market dynamics. This refined predictive framework aims to provide Donnelley Financial Solutions Inc. investors with a more informed and potentially profitable investment strategy.
ML Model Testing
n:Time series to forecast
p:Price signals of Donnelley Financial Solutions stock
j:Nash equilibria (Neural Network)
k:Dominated move of Donnelley Financial Solutions stock holders
a:Best response for Donnelley Financial Solutions target price
For further technical information as per how our model work we invite you to visit the article below:
How do KappaSignal algorithms actually work?
Donnelley Financial Solutions Stock Forecast (Buy or Sell) Strategic Interaction Table
Strategic Interaction Table Legend:
X axis: *Likelihood% (The higher the percentage value, the more likely the event will occur.)
Y axis: *Potential Impact% (The higher the percentage value, the more likely the price will deviate.)
Z axis (Grey to Black): *Technical Analysis%
Donnelley Financial Solutions Inc. (DFS) Financial Outlook and Forecast
Donnelley Financial Solutions (DFS) is a provider of financial data and information services, operating within a complex and evolving market. DFS's financial outlook is contingent upon several key factors, including the overall performance of the financial markets, the demand for its products and services, and the competitive landscape. The company's strength lies in its established brand recognition, specialized expertise, and extensive customer base. However, the competitive pressure from both established players and emerging technology companies warrants close scrutiny. DFS's ability to adapt to technological advancements and maintain its market share will be critical for future success. Key metrics to monitor include revenue growth, profitability margins, and customer retention rates, as these will provide insight into the company's effectiveness in navigating the industry dynamics.
Revenue projections for DFS appear to be moderate, given the ongoing digital transformation within the financial sector. While traditional financial data products remain in demand, the rise of alternative data and the adoption of sophisticated analytics by financial institutions are impacting the market. This change necessitates a strategic response from DFS. Investing in new technologies and developing data-driven solutions that are aligned with the evolving needs of the market could prove crucial. Customer relationship management and expansion into new vertical markets will be essential to drive sustainable growth. The company's history and reputation in delivering quality information products should provide a solid foundation. Careful evaluation of the market's current needs and prospective trends is critical for DFS to execute a successful transition and achieve the projected revenue gains.
Profitability is a key area for analysis. Factors impacting profitability include pricing strategies, operating costs, and the efficiency of its business operations. DFS's performance in these areas will be closely linked to its ability to effectively manage costs while maintaining competitiveness. Maintaining strong margins in a rapidly changing industry environment will require ongoing cost control initiatives and optimizing the use of technology to enhance operational efficiency. Strong cost management is critical for financial health, as increased competitive pressure from other companies in the same space could drive down profitability. Efficient resource allocation and a streamlined business model will be paramount to achieving and sustaining profitability over the forecast period.
Prediction: A cautiously positive outlook for DFS is warranted, given the company's existing market presence and brand reputation. This positive prediction is contingent on their ability to adapt to the evolving financial landscape, embrace technological innovations, and aggressively pursue opportunities to enter new markets or vertical segments. However, there are certain risks. Competition from new entrants and established players could put pressure on DFS's market share and profitability. Economic downturns could also negatively impact the demand for financial information services. Technological disruption also remains a risk. The company's success will hinge on its ability to navigate these challenges effectively, focusing on strategic partnerships, strong execution, and robust risk management. The ultimate financial success of DFS will depend on its successful response to these challenges and its commitment to continuous innovation.
Rating | Short-Term | Long-Term Senior |
---|---|---|
Outlook | B1 | Ba1 |
Income Statement | B2 | B2 |
Balance Sheet | Ba2 | Baa2 |
Leverage Ratios | C | Baa2 |
Cash Flow | Baa2 | Baa2 |
Rates of Return and Profitability | B2 | B2 |
*Financial analysis is the process of evaluating a company's financial performance and position by neural network. It involves reviewing the company's financial statements, including the balance sheet, income statement, and cash flow statement, as well as other financial reports and documents.
How does neural network examine financial reports and understand financial state of the company?
References
- Varian HR. 2014. Big data: new tricks for econometrics. J. Econ. Perspect. 28:3–28
- Jacobs B, Donkers B, Fok D. 2014. Product Recommendations Based on Latent Purchase Motivations. Rotterdam, Neth.: ERIM
- Zeileis A, Hothorn T, Hornik K. 2008. Model-based recursive partitioning. J. Comput. Graph. Stat. 17:492–514 Zhou Z, Athey S, Wager S. 2018. Offline multi-action policy learning: generalization and optimization. arXiv:1810.04778 [stat.ML]
- Rosenbaum PR, Rubin DB. 1983. The central role of the propensity score in observational studies for causal effects. Biometrika 70:41–55
- Bera, A. M. L. Higgins (1997), "ARCH and bilinearity as competing models for nonlinear dependence," Journal of Business Economic Statistics, 15, 43–50.
- Bastani H, Bayati M. 2015. Online decision-making with high-dimensional covariates. Work. Pap., Univ. Penn./ Stanford Grad. School Bus., Philadelphia/Stanford, CA
- uyer, S. Whiteson, B. Bakker, and N. A. Vlassis. Multiagent reinforcement learning for urban traffic control using coordination graphs. In Machine Learning and Knowledge Discovery in Databases, European Conference, ECML/PKDD 2008, Antwerp, Belgium, September 15-19, 2008, Proceedings, Part I, pages 656–671, 2008.