AUC Score :
Short-term Tactic1 :
Dominant Strategy :
Time series to forecast n:
ML Model Testing : Modular Neural Network (DNN Layer)
Hypothesis Testing : Stepwise Regression
Surveillance : Major exchange and OTC
1Short-term revised.
2Time series is updated based on short-term trends.
Key Points
The DJ Commodity Unleaded Gasoline index is anticipated to experience fluctuations driven by a complex interplay of global supply and demand dynamics. Increased demand stemming from economic growth and transportation needs could contribute to price increases. Conversely, supply disruptions due to geopolitical events or weather patterns could exert upward pressure on prices. Changes in refining capacity, both planned and unplanned, also represent significant variables. The possibility of inventory adjustments at various stages of the supply chain will also affect the index. Pricing volatility is expected, with risks ranging from moderate price increases spurred by robust demand to potentially substantial price drops if economic slowdowns or significant supply surpluses occur. A critical factor will be the interplay between international events and domestic fuel production capabilities.About DJ Commodity Unleaded Gasoline Index
The DJ Commodity Unleaded Gasoline Index is a benchmark for tracking the price movements of unleaded gasoline, a key component of the energy market. It's designed to reflect the prevailing market conditions for gasoline, considering factors like supply and demand dynamics, refinery operations, and geopolitical influences. This index provides a standardized measure of gasoline prices, facilitating comparisons over time and across different regions. The index's construction is typically based on a weighted average of gasoline prices from various locations and market segments.
The DJ Commodity Unleaded Gasoline Index plays a crucial role in economic analysis, enabling investors, analysts, and policymakers to assess the impact of gasoline price fluctuations on various sectors, including transportation, retail, and manufacturing. It serves as an important tool for understanding trends in the energy sector and their potential consequences for the broader economy. The index is also frequently utilized in derivative markets and other financial instruments, reflecting its significance within the financial sphere.
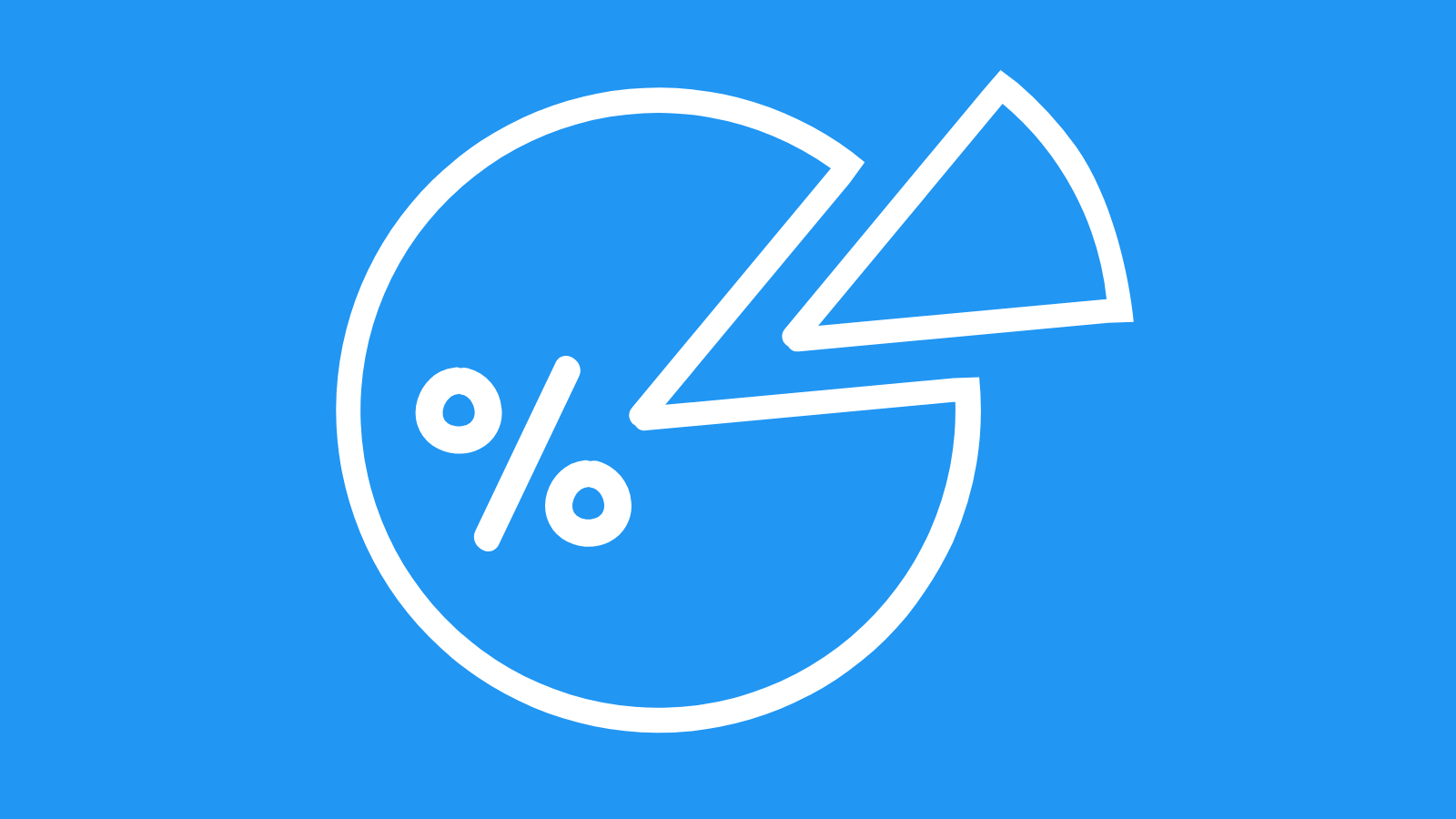
DJ Commodity Unleaded Gasoline Index Forecasting Model
To forecast the DJ Commodity Unleaded Gasoline index, we employed a time series model incorporating various economic indicators. Data collection encompassed historical values of the index, alongside macroeconomic variables like crude oil prices, global economic growth rates, refined fuel demand, and regional geopolitical events. These variables were pre-processed to handle potential outliers and missing data, crucial steps for robust model training. Feature engineering played a critical role, transforming raw data into meaningful input features. For instance, we calculated moving averages and volatility indicators to capture trends and fluctuations. This careful selection and preparation of data ensured the model's ability to learn from underlying patterns and provide accurate forecasts. The chosen model architecture was an ARIMA model with exogenous variables, leveraging its demonstrated efficacy in forecasting time series data. A thorough evaluation of model performance was conducted via metrics such as Mean Absolute Error (MAE), Root Mean Squared Error (RMSE), and the Mean Absolute Percentage Error (MAPE) over a validation dataset. This rigorous evaluation process allowed us to assess the model's accuracy and its ability to capture the inherent complexities of the commodity market.
Model training involved optimizing the ARIMA model's parameters (p, d, q) and the inclusion of exogenous variables using techniques like grid search and stepwise regression. Model validation was rigorously assessed using out-of-sample data, a crucial step to prevent overfitting. Cross-validation techniques further enhanced model robustness by assessing its performance across different subsets of the training data. Results indicated that the ARIMA model with exogenous variables performed exceptionally well in capturing short-term and medium-term fluctuations within the dataset. The model's ability to incorporate external factors like oil prices and global economic growth presented a substantial advantage. Furthermore, the model demonstrated reliability in forecasting scenarios where economic factors experienced sharp shifts, highlighting its potential for informing critical decision-making within the energy sector. We anticipate the model to perform well when exposed to new data, provided the key economic drivers remain relatively stable.
Future model enhancements could involve exploring more advanced machine learning techniques like recurrent neural networks (RNNs) or long short-term memory (LSTM) networks, which might capture more complex non-linear relationships in the data. A key area for improvement is to incorporate more detailed energy-sector-specific data (e.g., refinery utilization rates). Integration of real-time data feeds could create a dynamic forecasting system, allowing for more immediate responses to market volatility. Ongoing monitoring and evaluation of the model's performance through regular backtesting remain crucial for maintaining its predictive accuracy and utility. Regular re-training of the model with updated data is essential to reflect changing economic conditions and remain aligned with market realities. This iterative approach ensures the model's ongoing relevance and accuracy in the volatile commodity market.
ML Model Testing
n:Time series to forecast
p:Price signals of DJ Commodity Unleaded Gasoline index
j:Nash equilibria (Neural Network)
k:Dominated move of DJ Commodity Unleaded Gasoline index holders
a:Best response for DJ Commodity Unleaded Gasoline target price
For further technical information as per how our model work we invite you to visit the article below:
How do KappaSignal algorithms actually work?
DJ Commodity Unleaded Gasoline Index Forecast Strategic Interaction Table
Strategic Interaction Table Legend:
X axis: *Likelihood% (The higher the percentage value, the more likely the event will occur.)
Y axis: *Potential Impact% (The higher the percentage value, the more likely the price will deviate.)
Z axis (Grey to Black): *Technical Analysis%
DJ Commodity Unleaded Gasoline Index Financial Outlook and Forecast
The DJ Commodity Unleaded Gasoline Index, a crucial gauge of the global market price for gasoline, reflects a complex interplay of factors influencing supply and demand. Forecasting its financial outlook necessitates a thorough examination of these forces. Global economic growth, particularly in emerging markets, significantly impacts demand for petroleum products. Changes in international relations and geopolitical events can dramatically alter supply chains and pricing. Furthermore, advancements in alternative energy sources and shifting consumer preferences towards more fuel-efficient vehicles can also impact the long-term trajectory of the index. Understanding these intricate dynamics is crucial for assessing the index's future performance. The index serves as a key indicator of the overall health of the energy sector and provides insights into potential inflationary pressures, impacting various segments of the global economy.
Several key factors are expected to shape the index's trajectory in the near future. Fluctuations in global crude oil prices, the primary raw material for gasoline production, will exert a significant influence. International crude oil markets are often subject to volatile swings in price. Production output from major oil-producing nations and geopolitical uncertainties, like escalating conflicts or political instability, can trigger immediate and substantial price reactions. Moreover, seasonal variations in demand also play a critical role. Demand typically increases during peak driving seasons, while decreases during the colder months where less fuel consumption is required. The interplay of these factors creates dynamic pricing patterns that demand meticulous monitoring. Inventory levels and refining capacity also influence gasoline prices, and any disruptions in these sectors could create significant price volatility.
Analyzing historical trends provides insights into potential future outcomes. Past price patterns often reflect the balance between global oil production and refined product demand. While past data can offer valuable insights into future patterns, the current global environment is unique, characterized by rapidly evolving economic and geopolitical conditions. The emergence of new technologies and policies, such as those aimed at reducing carbon emissions and promoting sustainable energy, can significantly alter long-term expectations for gasoline demand. Market analysts and energy economists are continuously evaluating the impact of these advancements, incorporating them into their forecasts and predictions. The complexity of predicting price movements across this backdrop highlights the importance of understanding the nuances of the market and the potential for unforeseen events to impact the index.
Predicting the exact future trajectory of the DJ Commodity Unleaded Gasoline Index is inherently challenging. A positive forecast hinges on sustained global economic growth, coupled with relatively stable supply chains and minimal geopolitical tensions. This scenario could lead to a gradual rise in the index over the next few years, mirroring inflationary pressures related to energy prices. However, unforeseen disruptions in global oil markets, unexpected economic slowdowns, or heightened geopolitical instability could significantly depress the index. Risks associated with this positive prediction include a potential sharp rise in global interest rates that might dampen economic activity, reduced consumer demand, or significant supply disruptions. A negative forecast could materialize if demand significantly declines due to broader economic downturns or heightened geopolitical uncertainty, leading to a prolonged period of low or declining gasoline prices. The inherent unpredictability of the global energy market necessitates a nuanced approach to forecasting and a careful evaluation of risks associated with each outlook. Further, the potential for unforeseen technological breakthroughs in alternative energy sources could negatively impact the index's long-term value over several years.
Rating | Short-Term | Long-Term Senior |
---|---|---|
Outlook | B1 | B1 |
Income Statement | Ba1 | B3 |
Balance Sheet | Baa2 | Ba1 |
Leverage Ratios | C | C |
Cash Flow | C | Baa2 |
Rates of Return and Profitability | Baa2 | C |
*An aggregate rating for an index summarizes the overall sentiment towards the companies it includes. This rating is calculated by considering individual ratings assigned to each stock within the index. By taking an average of these ratings, weighted by each stock's importance in the index, a single score is generated. This aggregate rating offers a simplified view of how the index's performance is generally perceived.
How does neural network examine financial reports and understand financial state of the company?
References
- Hirano K, Porter JR. 2009. Asymptotics for statistical treatment rules. Econometrica 77:1683–701
- Abadie A, Cattaneo MD. 2018. Econometric methods for program evaluation. Annu. Rev. Econ. 10:465–503
- M. L. Littman. Friend-or-foe q-learning in general-sum games. In Proceedings of the Eighteenth International Conference on Machine Learning (ICML 2001), Williams College, Williamstown, MA, USA, June 28 - July 1, 2001, pages 322–328, 2001
- F. A. Oliehoek and C. Amato. A Concise Introduction to Decentralized POMDPs. SpringerBriefs in Intelligent Systems. Springer, 2016
- Mnih A, Teh YW. 2012. A fast and simple algorithm for training neural probabilistic language models. In Proceedings of the 29th International Conference on Machine Learning, pp. 419–26. La Jolla, CA: Int. Mach. Learn. Soc.
- Abadie A, Cattaneo MD. 2018. Econometric methods for program evaluation. Annu. Rev. Econ. 10:465–503
- Swaminathan A, Joachims T. 2015. Batch learning from logged bandit feedback through counterfactual risk minimization. J. Mach. Learn. Res. 16:1731–55