AUC Score :
Short-term Tactic1 :
Dominant Strategy :
Time series to forecast n:
ML Model Testing : Supervised Machine Learning (ML)
Hypothesis Testing : Logistic Regression
Surveillance : Major exchange and OTC
1Short-term revised.
2Time series is updated based on short-term trends.
Key Points
The DJ Commodity Energy index is projected to exhibit moderate volatility. A significant factor influencing its trajectory will be the interplay of global energy demand and supply dynamics. Increased demand, potentially driven by economic growth or geopolitical events, could lead to price increases. Conversely, supply disruptions or a slowdown in economic activity could result in price decreases. Geopolitical tensions and regulatory changes in key energy-producing regions will also contribute to price fluctuations. The risk associated with these predictions includes the possibility of unforeseen events significantly impacting energy markets, leading to substantial price swings that could exceed anticipated levels of volatility. Also, the accuracy of demand forecasts and supply assessments is inherently uncertain, which contributes to the overall risk in predicting the index's future performance.About DJ Commodity Energy Index
The DJ Commodity Energy Index is a market-capitalization-weighted index that tracks the performance of publicly traded companies primarily involved in the energy sector. Comprising a diverse group of companies engaged in exploration, production, refining, and distribution of various energy products like oil, natural gas, and coal, the index provides a broad perspective on the overall health and trajectory of the energy sector. It serves as a key benchmark for investors seeking exposure to energy-related equities and allows for a comparison of different energy company performance. The index's composition and methodology are regularly reviewed and adjusted to ensure accuracy and relevance to the current market conditions.
Fluctuations in the index reflect various market forces impacting the energy sector, including supply and demand dynamics, geopolitical events, regulatory changes, and technological advancements. The index's historical performance provides insights into the sector's long-term trends, and its current performance can serve as a guide for investors navigating potential market risks and opportunities within the energy sector. Changes in the index often reflect the market's assessment of these factors influencing the value and outlook of energy companies.
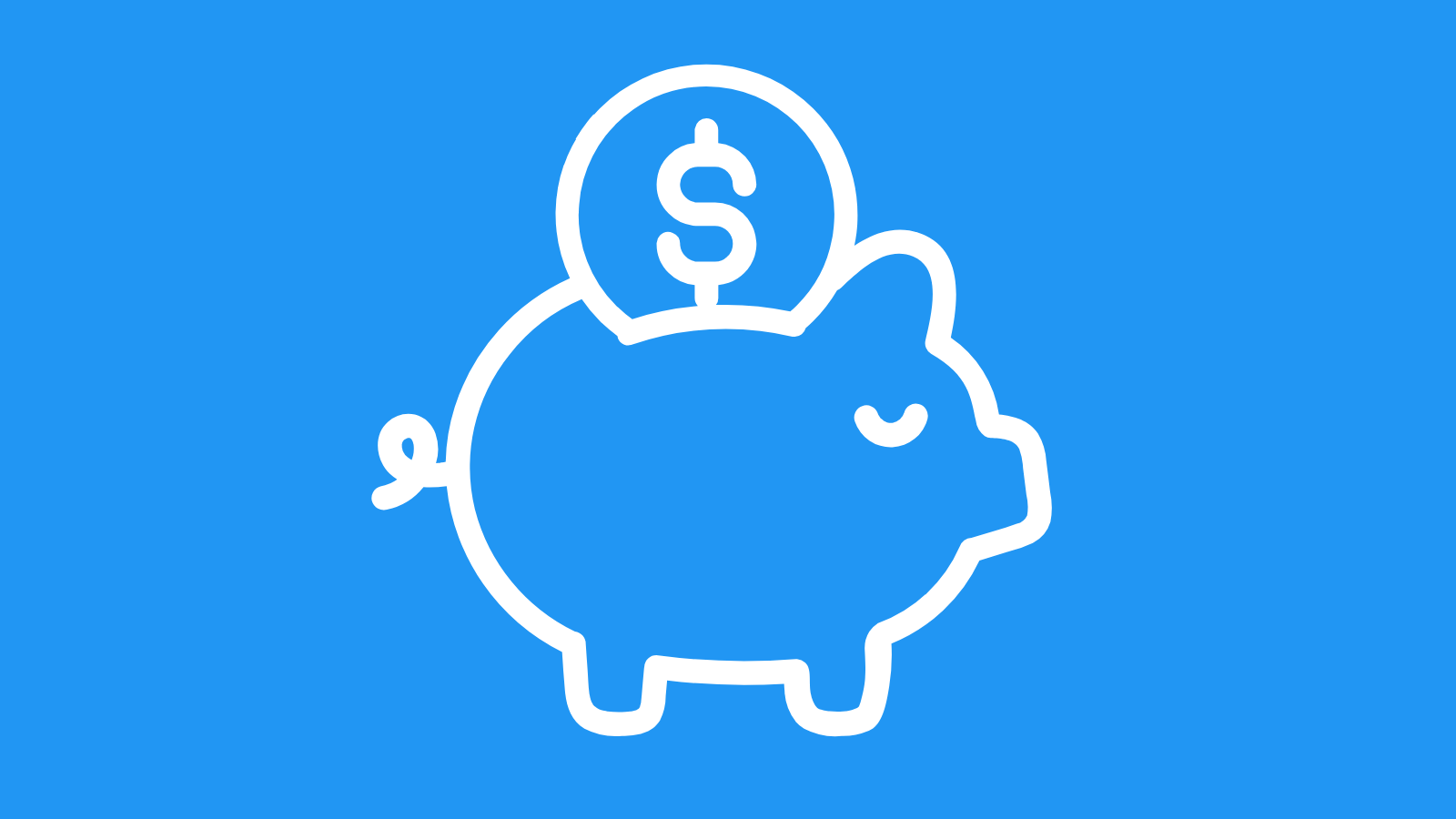
DJ Commodity Energy Index Forecast Model
To predict the future trajectory of the DJ Commodity Energy Index, a multi-faceted approach combining machine learning techniques with economic indicators is employed. Initial steps involve data preprocessing and feature engineering. Historical data encompassing a wide range of relevant factors, including global energy demand projections, geopolitical events (e.g., supply disruptions, conflicts), crude oil prices, and production capacity, are meticulously collected and cleaned. Crucial variables, such as the availability of renewable energy sources and their increasing penetration into energy markets, are also incorporated. This comprehensive dataset is then transformed into suitable formats for machine learning algorithms. We utilize a time series approach, employing models capable of capturing trends, seasonality, and cyclical patterns inherent in the energy sector. This dataset will allow the model to identify historical patterns and correlations, which will eventually predict the future movements of the index.
Several machine learning models are evaluated and compared in terms of their predictive performance. Recurrent Neural Networks (RNNs), particularly Long Short-Term Memory (LSTM) networks, are favored due to their capacity to handle sequential data and long-term dependencies. Furthermore, a suite of traditional time series models, such as ARIMA and GARCH, are also included for comparison. Model selection is based on metrics such as Mean Absolute Error (MAE), Root Mean Squared Error (RMSE), and R-squared values. Careful consideration of model complexity and overfitting is implemented to ensure robust performance. The goal is to develop a model that generalizes well to future data points, ensuring the accuracy of the predictions.
After training and evaluating the chosen model, a thorough sensitivity analysis is performed. This involves examining the influence of individual features on the model's predictions to understand the drivers of energy price fluctuations. Further, the inclusion of expert knowledge is considered as well as external economic factors that could influence the predictions. A comprehensive report on the model's performance, including predicted values, key metrics, and potential limitations, is generated to facilitate informed decision-making. Model deployment and monitoring will involve a continuous process of retraining and updating the model with new data and insights to maintain its accuracy and adaptability to market fluctuations. Ongoing monitoring is essential to detect and respond to shifts in energy market dynamics.
ML Model Testing
n:Time series to forecast
p:Price signals of DJ Commodity Energy index
j:Nash equilibria (Neural Network)
k:Dominated move of DJ Commodity Energy index holders
a:Best response for DJ Commodity Energy target price
For further technical information as per how our model work we invite you to visit the article below:
How do KappaSignal algorithms actually work?
DJ Commodity Energy Index Forecast Strategic Interaction Table
Strategic Interaction Table Legend:
X axis: *Likelihood% (The higher the percentage value, the more likely the event will occur.)
Y axis: *Potential Impact% (The higher the percentage value, the more likely the price will deviate.)
Z axis (Grey to Black): *Technical Analysis%
DJ Commodity Energy Index Financial Outlook and Forecast
The DJ Commodity Energy Index, a benchmark tracking the performance of energy commodities, presents a complex financial outlook shaped by a multitude of interwoven factors. Recent trends point to both potential opportunities and significant challenges. Supply chain disruptions, geopolitical instability, and fluctuations in global demand are among the key drivers influencing the index's trajectory. Analysis of historical data, current market conditions, and expert opinions suggest a mixed picture. Factors such as technological advancements, evolving regulations, and shifts in consumer behavior play critical roles in determining the index's future performance. A comprehensive understanding of these dynamic elements is crucial for navigating the intricacies of this market segment.
Several key indicators provide insights into the expected direction of the DJ Commodity Energy Index. Rising global energy demand, particularly from developing economies, coupled with the increasing need for reliable energy sources, could contribute to sustained price increases. However, challenges related to energy production capacity and environmental concerns pose significant headwinds. Furthermore, the ongoing geopolitical landscape and its implications for energy production and trade are critical determinants. The ongoing transition to renewable energy sources, albeit gradual, introduces uncertainty and could potentially constrain price appreciation for fossil fuels. Government regulations regarding emissions and energy efficiency also influence the overall outlook. These are but some of the critical factors driving the complexities of this market.
Forecasting the precise trajectory of the DJ Commodity Energy Index remains a challenging endeavor. While optimistic predictions anticipate continued growth driven by elevated energy demand, particularly in emerging markets, the increasing adoption of renewable energy technologies suggests a more muted or potentially negative long-term outlook. Economic downturns and energy conservation measures could lead to diminished demand and, consequently, impact the price of commodities. A combination of various factors – including technological advancements, policy shifts, and global market dynamics – could produce a dynamic and unpredictable future for the index. It is essential to acknowledge the potential for substantial volatility and the importance of diversification to mitigate associated risks.
Predicting the future performance of the DJ Commodity Energy Index carries inherent risks. A positive prediction, based on sustained global energy demand and limited availability of sustainable alternatives, could be challenged by heightened environmental regulations or a swift shift towards renewable energy sources. This transition could significantly impact the value proposition of fossil fuels. Conversely, if demand remains moderate or weakens in the face of economic headwinds, this could translate into a negative outlook, impacting commodity prices. Risks associated with geopolitical instability, supply chain disruptions, and significant price fluctuations are significant factors to consider. Therefore, any prediction regarding the index's future direction should be approached with caution and a clear understanding of the associated inherent risks. Diversification across various asset classes within the energy sector and beyond is crucial for mitigating potential negative impacts on investment portfolios.
Rating | Short-Term | Long-Term Senior |
---|---|---|
Outlook | Ba3 | Ba3 |
Income Statement | Baa2 | B2 |
Balance Sheet | Baa2 | Caa2 |
Leverage Ratios | Baa2 | B3 |
Cash Flow | C | Baa2 |
Rates of Return and Profitability | B2 | Baa2 |
*An aggregate rating for an index summarizes the overall sentiment towards the companies it includes. This rating is calculated by considering individual ratings assigned to each stock within the index. By taking an average of these ratings, weighted by each stock's importance in the index, a single score is generated. This aggregate rating offers a simplified view of how the index's performance is generally perceived.
How does neural network examine financial reports and understand financial state of the company?
References
- Holland PW. 1986. Statistics and causal inference. J. Am. Stat. Assoc. 81:945–60
- C. Wu and Y. Lin. Minimizing risk models in Markov decision processes with policies depending on target values. Journal of Mathematical Analysis and Applications, 231(1):47–67, 1999
- Van der Vaart AW. 2000. Asymptotic Statistics. Cambridge, UK: Cambridge Univ. Press
- Batchelor, R. P. Dua (1993), "Survey vs ARCH measures of inflation uncertainty," Oxford Bulletin of Economics Statistics, 55, 341–353.
- Breusch, T. S. A. R. Pagan (1979), "A simple test for heteroskedasticity and random coefficient variation," Econometrica, 47, 1287–1294.
- J. Peters, S. Vijayakumar, and S. Schaal. Natural actor-critic. In Proceedings of the Sixteenth European Conference on Machine Learning, pages 280–291, 2005.
- Jorgenson, D.W., Weitzman, M.L., ZXhang, Y.X., Haxo, Y.M. and Mat, Y.X., 2023. Can Neural Networks Predict Stock Market?. AC Investment Research Journal, 220(44).