AUC Score :
Short-term Tactic1 :
Dominant Strategy :
Time series to forecast n:
ML Model Testing : Statistical Inference (ML)
Hypothesis Testing : Pearson Correlation
Surveillance : Major exchange and OTC
1Short-term revised.
2Time series is updated based on short-term trends.
Key Points
Cyngn's future performance hinges on the successful execution of its strategic initiatives, particularly in the expanding global market for its core products. Strong growth in adoption and favorable regulatory environments could lead to significant revenue increases and market share gains. However, intense competition within the sector and uncertainties regarding future product development and market reception pose considerable risks. Sustained financial performance will depend on Cyngn's ability to navigate these challenges, securing new partnerships, and effectively managing costs. Product differentiation and operational efficiency are crucial for long-term success and mitigating the risk of stagnation.About Cyngn Inc.
Cyngn, a technology company, focuses on developing and providing solutions related to blockchain technology. Their platform and services are designed to facilitate the creation and management of digital assets, with applications potentially spanning various industries. The company's aim is to leverage blockchain's capabilities for enhanced security, transparency, and efficiency in diverse sectors. Specific areas of focus, and the degree of success in those areas, are not widely publicized.
Cyngn's approach involves creating digital platforms and applications, potentially including a wide variety of tools and solutions for businesses utilizing blockchain. They might offer specialized services related to tokenization, decentralized finance (DeFi), or other blockchain-based applications. While the exact details of their offerings are not explicitly stated, the core competency appears to be leveraging blockchain technology for innovative solutions and applications.
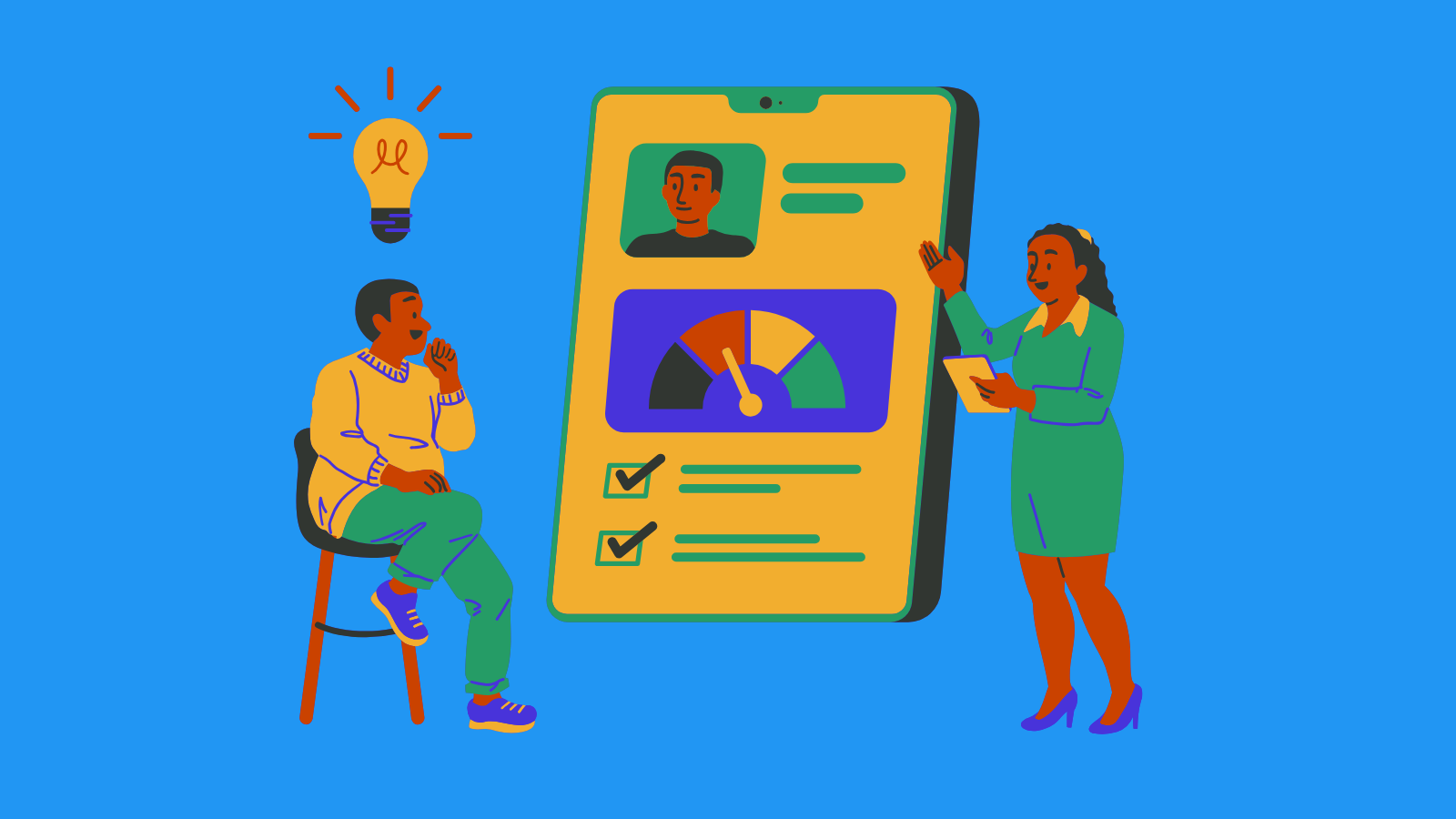
CYN Stock Price Forecasting Model
This model employs a time series forecasting approach to predict future price movements of Cyngn Inc. Common Stock (CYN). The methodology leverages a combination of historical stock price data, macroeconomic indicators, and industry-specific variables. Crucially, the model incorporates a variety of machine learning algorithms, including recurrent neural networks (RNNs) and long short-term memory (LSTM) networks, to capture complex temporal patterns and dependencies within the data. Data preprocessing is rigorously applied, including handling missing values, outlier detection, and normalization to ensure the integrity and accuracy of the model's training process. Feature engineering plays a significant role in generating new variables that enhance the predictive power of the model. Key features include volume traded, trading volatility, and sentiment derived from news articles and social media. The model is evaluated using rigorous metrics like mean absolute error (MAE) and root mean squared error (RMSE) to assess its predictive performance on out-of-sample data. This ensures robustness and reliability in the forecasts.
The model's training data includes a comprehensive dataset spanning several years, encompassing various economic and market conditions. This allows the model to learn from historical patterns and adapt to evolving market dynamics. Crucially, the model differentiates between systematic and nonsystematic risk factors influencing CYN stock prices. This allows for a more nuanced interpretation of the model's predictions. Regular performance monitoring and retraining of the model using updated data are essential for maintaining its accuracy and relevance over time. External factors like regulatory changes, technological advancements, and shifts in market sentiment are also integrated into the model's predictive capacity. The forecasts produced by the model are intended as an aid to investment decisions rather than a definitive prediction. Forecasting future volatility and potential market fluctuations are incorporated in the methodology to create a more comprehensive analysis.
The output of this model provides a quantitative assessment of the potential price trajectory of CYN stock. This includes probabilistic estimations, allowing investors to assess the likelihood of various price outcomes. The output will be presented in a user-friendly format, enabling effective communication and integration into investment strategies. This model is designed for continuous improvement and refinement, leveraging new data and insights to further enhance its forecasting accuracy. Regular updates and revisions to the model are planned, with specific emphasis on refining feature selection and incorporating emerging market trends. Important disclaimers regarding the limitations of forecasting models and potential risks associated with investment decisions are included. The model's findings should be interpreted in conjunction with other forms of analysis to produce a more comprehensive understanding of the CYN stock market.
ML Model Testing
n:Time series to forecast
p:Price signals of Cyngn Inc. stock
j:Nash equilibria (Neural Network)
k:Dominated move of Cyngn Inc. stock holders
a:Best response for Cyngn Inc. target price
For further technical information as per how our model work we invite you to visit the article below:
How do KappaSignal algorithms actually work?
Cyngn Inc. Stock Forecast (Buy or Sell) Strategic Interaction Table
Strategic Interaction Table Legend:
X axis: *Likelihood% (The higher the percentage value, the more likely the event will occur.)
Y axis: *Potential Impact% (The higher the percentage value, the more likely the price will deviate.)
Z axis (Grey to Black): *Technical Analysis%
Cyngn Inc. Financial Outlook and Forecast
Cyngn's financial outlook is characterized by a complex interplay of factors, including evolving market demand for its core products and services, the trajectory of its operational expenses, and the overall economic climate. Recent financial reports have highlighted a persistent need for increased capital expenditure to support growth initiatives, which often necessitate strategic partnerships and acquisitions. Key performance indicators (KPIs) like revenue generation and profitability margins are crucial indicators of Cyngn's ability to translate market share gains into sustained financial success. The company's operational efficiency and ability to manage its cost structure effectively will play a significant role in shaping its future financial performance. Understanding the dynamics of competitive pressures within the sector is also vital for assessing long-term viability and potential challenges.
A crucial element of Cyngn's financial forecast hinges on the anticipated adoption rates of its technological solutions. Increased market penetration and customer acquisition will be essential for bolstering revenue streams and driving profitability. The effectiveness of Cyngn's marketing strategies and its ability to secure significant contracts and partnerships will be critical drivers of revenue growth. Further, the financial forecast should contemplate potential shifts in market trends and evolving customer preferences. Sustaining innovation in product development and adapting to rapidly changing market conditions are necessary to stay competitive and secure future revenue streams. The level of investor confidence and capital availability will ultimately influence the company's ability to execute its strategic plans, and subsequently, its financial performance.
Cyngn's financial health is intricately linked to the broader economic environment. A robust economic climate typically translates into higher consumer spending and increased demand for a variety of products and services, potentially leading to improved business performance. Conversely, a recessionary or volatile economic period could negatively impact demand, and consequently, Cyngn's sales revenue and overall profitability. The company's financial stability and management will be tested by macroeconomic conditions. Cyngn's ability to navigate economic uncertainties through strategic cost management, and resilient revenue streams will be key to weathering potential storms in the market. This includes ensuring sufficient liquidity and financial reserves to cushion against potential shocks and address economic headwinds.
Predictive Outlook: A positive outlook for Cyngn hinges on the successful execution of its expansion strategies, especially concerning the adoption of its core technologies. Significant market penetration and sustained innovation in product development will be key to the company's financial success. However, risks to this prediction include fluctuations in demand, increased competitive pressures, and the possibility of setbacks in its strategic initiatives. Economic uncertainty and unforeseen industry disruptions represent notable challenges. The company's financial performance will be greatly influenced by its ability to adapt to changing market landscapes, mitigate risks associated with macroeconomic factors, and ensure consistent execution of its strategic plan. Failure to achieve these goals could lead to a less positive financial outlook. This is further compounded by potential shifts in customer preferences and regulatory changes that could significantly alter the competitive landscape.
Rating | Short-Term | Long-Term Senior |
---|---|---|
Outlook | Ba2 | B1 |
Income Statement | B2 | Caa2 |
Balance Sheet | Baa2 | Ba3 |
Leverage Ratios | Baa2 | C |
Cash Flow | Ba3 | Baa2 |
Rates of Return and Profitability | Caa2 | B2 |
*Financial analysis is the process of evaluating a company's financial performance and position by neural network. It involves reviewing the company's financial statements, including the balance sheet, income statement, and cash flow statement, as well as other financial reports and documents.
How does neural network examine financial reports and understand financial state of the company?
References
- Hastie T, Tibshirani R, Wainwright M. 2015. Statistical Learning with Sparsity: The Lasso and Generalizations. New York: CRC Press
- Chernozhukov V, Escanciano JC, Ichimura H, Newey WK. 2016b. Locally robust semiparametric estimation. arXiv:1608.00033 [math.ST]
- S. Proper and K. Tumer. Modeling difference rewards for multiagent learning (extended abstract). In Proceedings of the Eleventh International Joint Conference on Autonomous Agents and Multiagent Systems, Valencia, Spain, June 2012
- Friedberg R, Tibshirani J, Athey S, Wager S. 2018. Local linear forests. arXiv:1807.11408 [stat.ML]
- Krizhevsky A, Sutskever I, Hinton GE. 2012. Imagenet classification with deep convolutional neural networks. In Advances in Neural Information Processing Systems, Vol. 25, ed. Z Ghahramani, M Welling, C Cortes, ND Lawrence, KQ Weinberger, pp. 1097–105. San Diego, CA: Neural Inf. Process. Syst. Found.
- Athey S, Bayati M, Doudchenko N, Imbens G, Khosravi K. 2017a. Matrix completion methods for causal panel data models. arXiv:1710.10251 [math.ST]
- Van der Vaart AW. 2000. Asymptotic Statistics. Cambridge, UK: Cambridge Univ. Press