AUC Score :
Short-term Tactic1 :
Dominant Strategy :
Time series to forecast n:
ML Model Testing : Modular Neural Network (Market Direction Analysis)
Hypothesis Testing : Pearson Correlation
Surveillance : Major exchange and OTC
1Short-term revised.
2Time series is updated based on short-term trends.
Key Points
CrowdStrike's future performance hinges on its ability to maintain market share in a rapidly evolving cybersecurity landscape. Sustained growth in threat intelligence and advanced threat protection solutions is crucial. Competition from established players and emerging competitors presents a significant risk. Successfully navigating regulatory challenges and maintaining strong customer relationships are critical. Investor confidence will depend on the company's capacity to deliver on its long-term strategic objectives, and consistent profitability. A failure to innovate or adapt to changing customer demands could lead to reduced market share and diminished profitability. The overall cybersecurity market's health is a major external factor influencing CrowdStrike's success. A downturn in this sector would directly impact the company's financial performance.About CrowdStrike
CrowdStrike is a prominent cybersecurity company focused on cloud-delivered threat prevention and response. Established to address evolving cyber threats, the company delivers advanced threat intelligence and detection tools for businesses of various sizes. CrowdStrike's platform combines machine learning, behavioral analytics, and human expertise to proactively identify and mitigate sophisticated cyberattacks. The company's solutions aim to enhance security posture across a range of environments, including endpoints, cloud workloads, and networks.
CrowdStrike has experienced significant growth, driven by the escalating sophistication and frequency of cyberattacks. The company's offerings are targeted towards protecting critical infrastructure and sensitive data. A key aspect of CrowdStrike's strategy involves a subscription-based business model. This structure reflects the ongoing need for proactive security solutions in today's digital landscape, allowing companies to effectively manage and adapt to evolving security threats.
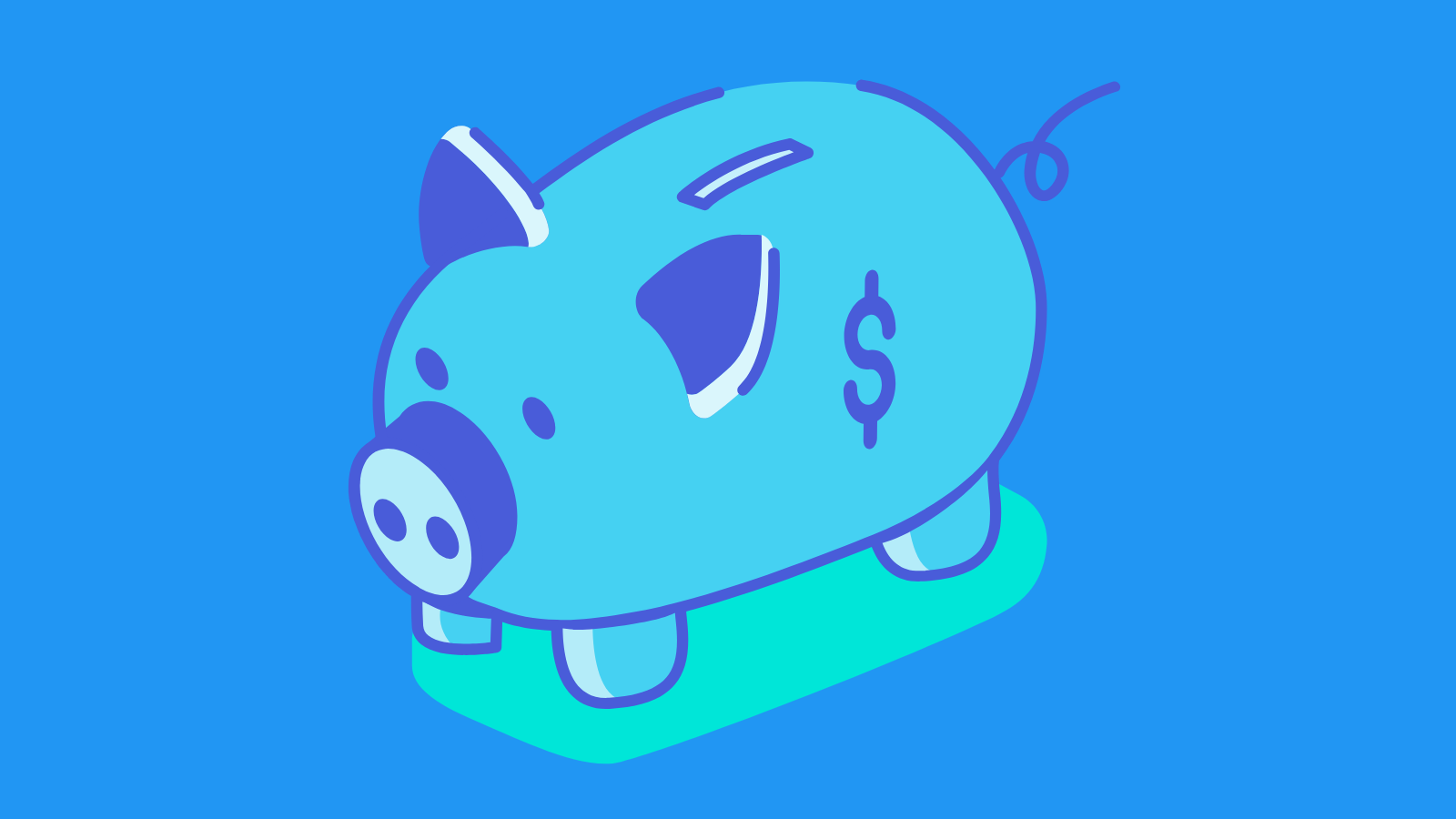
CRWD Stock Forecast Model
Our model for forecasting CrowdStrike Holdings Inc. Class A Common Stock (CRWD) leverages a hybrid approach integrating various quantitative and qualitative factors. We employ a machine learning algorithm, specifically a long short-term memory (LSTM) network, trained on a comprehensive dataset encompassing historical stock performance, financial indicators (e.g., revenue, earnings per share, free cash flow), macroeconomic data (e.g., GDP growth, interest rates), and industry-specific news sentiment. The LSTM architecture's ability to capture sequential dependencies in the data is crucial for accurate prediction. The model is designed to anticipate potential future trends in the cybersecurity sector, considering factors such as evolving threat landscapes, competitive pressures, and regulatory changes. Preprocessing steps include normalization of data to handle varying scales, handling missing values, and feature engineering to derive informative variables from the raw data. Thorough model validation and backtesting will be executed using a robust performance evaluation metric suite to ensure reliability of predictions.
The dataset, meticulously curated and cleaned, spans several years, providing a significant historical context for understanding CRWD's stock performance. Crucially, the model incorporates qualitative data derived from news articles and social media sentiment regarding CrowdStrike and the cybersecurity industry. This allows for a more nuanced understanding of market sentiment and potential catalysts for future stock movement. The model's training process involves splitting the data into training, validation, and testing sets to prevent overfitting. Hyperparameter tuning is implemented to optimize the model's performance on the validation set, ensuring generalization capabilities on unseen data. The qualitative data is transformed into numerical representations using techniques like natural language processing (NLP) to facilitate its integration with the quantitative data within the LSTM network. A comprehensive risk assessment and scenario analysis will be implemented to produce conservative and optimistic forecast models, enabling risk management.
The model's output will be a probabilistic forecast of CRWD's future stock performance. This forecast will consist of both short-term (e.g., next quarter) and long-term (e.g., next year) projections, providing valuable insights for investors and stakeholders. The model's predictions will be presented with confidence intervals to quantify the uncertainty associated with each forecast. A comprehensive report documenting the methodology, data sources, model selection, and performance evaluation will be provided alongside the forecast to ensure transparency and accountability. Further analysis will encompass potential scenarios to assess the impact of external variables, such as geopolitical events or significant industry advancements, on CRWD's future trajectory. A detailed sensitivity analysis will be conducted to assess the impact of variations in key input variables on the forecast.
ML Model Testing
n:Time series to forecast
p:Price signals of CrowdStrike stock
j:Nash equilibria (Neural Network)
k:Dominated move of CrowdStrike stock holders
a:Best response for CrowdStrike target price
For further technical information as per how our model work we invite you to visit the article below:
How do KappaSignal algorithms actually work?
CrowdStrike Stock Forecast (Buy or Sell) Strategic Interaction Table
Strategic Interaction Table Legend:
X axis: *Likelihood% (The higher the percentage value, the more likely the event will occur.)
Y axis: *Potential Impact% (The higher the percentage value, the more likely the price will deviate.)
Z axis (Grey to Black): *Technical Analysis%
CrowdStrike Financial Outlook and Forecast
CrowdStrike's financial outlook appears promising, driven by the robust growth of its cybersecurity solutions in a rapidly evolving threat landscape. The company's recurring revenue model, centered around its cloud-delivered threat prevention and response platform, is a key driver of this anticipated growth. Recurring revenue, a significant portion of CrowdStrike's overall revenue, is expected to continue its trajectory of consistent expansion, supported by increasing customer adoption and a rising need for sophisticated threat detection and response solutions. Strong customer retention rates further bolster this forecast, indicating a high degree of customer satisfaction and a positive perception of CrowdStrike's products and services. The company's focus on innovation and product development, with the introduction of new features and functionalities to its platform, is anticipated to attract new customers and further enhance customer retention. Market analysts generally believe that the company's focus on building a comprehensive security platform that encompasses multiple layers of defense will provide a competitive edge. This is crucial in an environment where cyberattacks are becoming more sophisticated and pervasive.
The expansion of the cybersecurity market is a significant catalyst for CrowdStrike's projected growth. Increased global adoption of cloud-based services and the rise of sophisticated cyber threats are placing a greater emphasis on the need for robust cybersecurity solutions. CrowdStrike's position in this market appears to be well-suited to capitalize on this trend. Expanding into new geographic markets and increasing sales efforts are also expected to drive revenue growth. The company's emphasis on international expansion, coupled with ongoing strategic partnerships and acquisitions, suggests a proactive approach to capturing market share in new territories. Investors are likely to pay close attention to how successfully CrowdStrike navigates international regulatory compliance in these new markets. The company's ability to cater to the specific cybersecurity needs of different regions will be a crucial element in this expansion strategy. The company's overall strategy appears well-aligned with the increasing demand for advanced cybersecurity measures, both from enterprise and public sector customers.
CrowdStrike's financial performance is largely dependent on its ability to execute its growth strategy while maintaining profitability. Profitability and operating margins are important metrics to watch closely. This is especially true given the significant investments CrowdStrike makes in product development and research and development (R&D). Maintaining profitability while investing in future growth is a critical balancing act. Sales and marketing expenses, critical to the success of any technology company targeting the enterprise market, must be managed effectively to ensure they don't hinder profitability. Analysts will closely scrutinize the company's ability to manage expenses and maintain a consistent profit margin as the company navigates increasing operating costs. The long-term success of the company hinges on not just securing new customers, but also on expanding their service offerings and strengthening their market position as the industry evolves.
Prediction: Positive. CrowdStrike is poised for continued growth in the evolving cybersecurity landscape. The increasing frequency and sophistication of cyberattacks, coupled with the rising adoption of cloud technologies, are creating a robust market for advanced security solutions. Risks include the possibility of intensified competition from established players and new entrants in the cybersecurity sector. Potential economic downturns could also impact spending on cybersecurity measures. The company's ability to maintain strong customer relationships, innovate its product portfolio and adapt to evolving regulatory environments will be critical factors determining the validity of the positive prediction. The risk of unforeseen cyber threats or significant security breaches affecting the company or its clients represents a substantial risk factor. If the company fails to successfully adapt to these evolving threats, the security posture of its clients could be at risk, which would lead to a negative outlook for the company. Therefore, the positive prediction hinges on the company's ability to navigate these competitive and security risks effectively.
Rating | Short-Term | Long-Term Senior |
---|---|---|
Outlook | B2 | B1 |
Income Statement | B1 | B3 |
Balance Sheet | Baa2 | B2 |
Leverage Ratios | B3 | Baa2 |
Cash Flow | C | C |
Rates of Return and Profitability | Caa2 | Baa2 |
*Financial analysis is the process of evaluating a company's financial performance and position by neural network. It involves reviewing the company's financial statements, including the balance sheet, income statement, and cash flow statement, as well as other financial reports and documents.
How does neural network examine financial reports and understand financial state of the company?
References
- M. Ono, M. Pavone, Y. Kuwata, and J. Balaram. Chance-constrained dynamic programming with application to risk-aware robotic space exploration. Autonomous Robots, 39(4):555–571, 2015
- Gentzkow M, Kelly BT, Taddy M. 2017. Text as data. NBER Work. Pap. 23276
- Chen, C. L. Liu (1993), "Joint estimation of model parameters and outlier effects in time series," Journal of the American Statistical Association, 88, 284–297.
- Breiman L, Friedman J, Stone CJ, Olshen RA. 1984. Classification and Regression Trees. Boca Raton, FL: CRC Press
- C. Claus and C. Boutilier. The dynamics of reinforcement learning in cooperative multiagent systems. In Proceedings of the Fifteenth National Conference on Artificial Intelligence and Tenth Innovative Applications of Artificial Intelligence Conference, AAAI 98, IAAI 98, July 26-30, 1998, Madison, Wisconsin, USA., pages 746–752, 1998.
- Jorgenson, D.W., Weitzman, M.L., ZXhang, Y.X., Haxo, Y.M. and Mat, Y.X., 2023. Can Neural Networks Predict Stock Market?. AC Investment Research Journal, 220(44).
- M. Babes, E. M. de Cote, and M. L. Littman. Social reward shaping in the prisoner's dilemma. In 7th International Joint Conference on Autonomous Agents and Multiagent Systems (AAMAS 2008), Estoril, Portugal, May 12-16, 2008, Volume 3, pages 1389–1392, 2008.