AUC Score :
Short-term Tactic1 :
Dominant Strategy :
Time series to forecast n:
ML Model Testing : Multi-Task Learning (ML)
Hypothesis Testing : Pearson Correlation
Surveillance : Major exchange and OTC
1Short-term revised.
2Time series is updated based on short-term trends.
Key Points
Covenant Logistics's future performance hinges on several key factors. Sustained e-commerce growth and increasing demand for logistics services are anticipated to drive revenue. However, fluctuations in fuel prices and shifts in economic conditions could create volatility in the company's operating margins. Competition within the logistics sector will likely remain intense. The company's ability to adapt to changing market dynamics and maintain its operational efficiency will be crucial to achieving consistent profitability. Potential disruptions in global supply chains could also negatively impact Covenant Logistics's performance. Given these factors, the risk of significant price fluctuations and inconsistent profitability warrants careful consideration.About Covenant Logistics Group
Covenant Logistics Group (CLG) is a leading provider of transportation and logistics services, operating a diversified network across North America. The company focuses on providing integrated solutions for various industries, encompassing freight forwarding, warehousing, and specialized transportation. CLG's commitment to customer service and operational efficiency is central to its business strategy, with a focus on technology-driven solutions and optimized supply chain management. They primarily serve a range of industries including manufacturing, retail, and healthcare. CLG's substantial scale and established network give it a significant footprint in the transportation and logistics sector.
CLG's business model emphasizes a combination of dedicated transportation, specialized services like temperature-controlled freight, and broader logistics support. The company's diverse customer base, coupled with its operational efficiency, contributes to its profitability and market share. Furthermore, CLG is actively seeking to expand its services and geographical reach to maximize opportunities within the growing logistics industry. Key performance indicators like on-time delivery and cost-effectiveness are integral to their business operations, reflecting a dedication to customer satisfaction and market competitiveness.
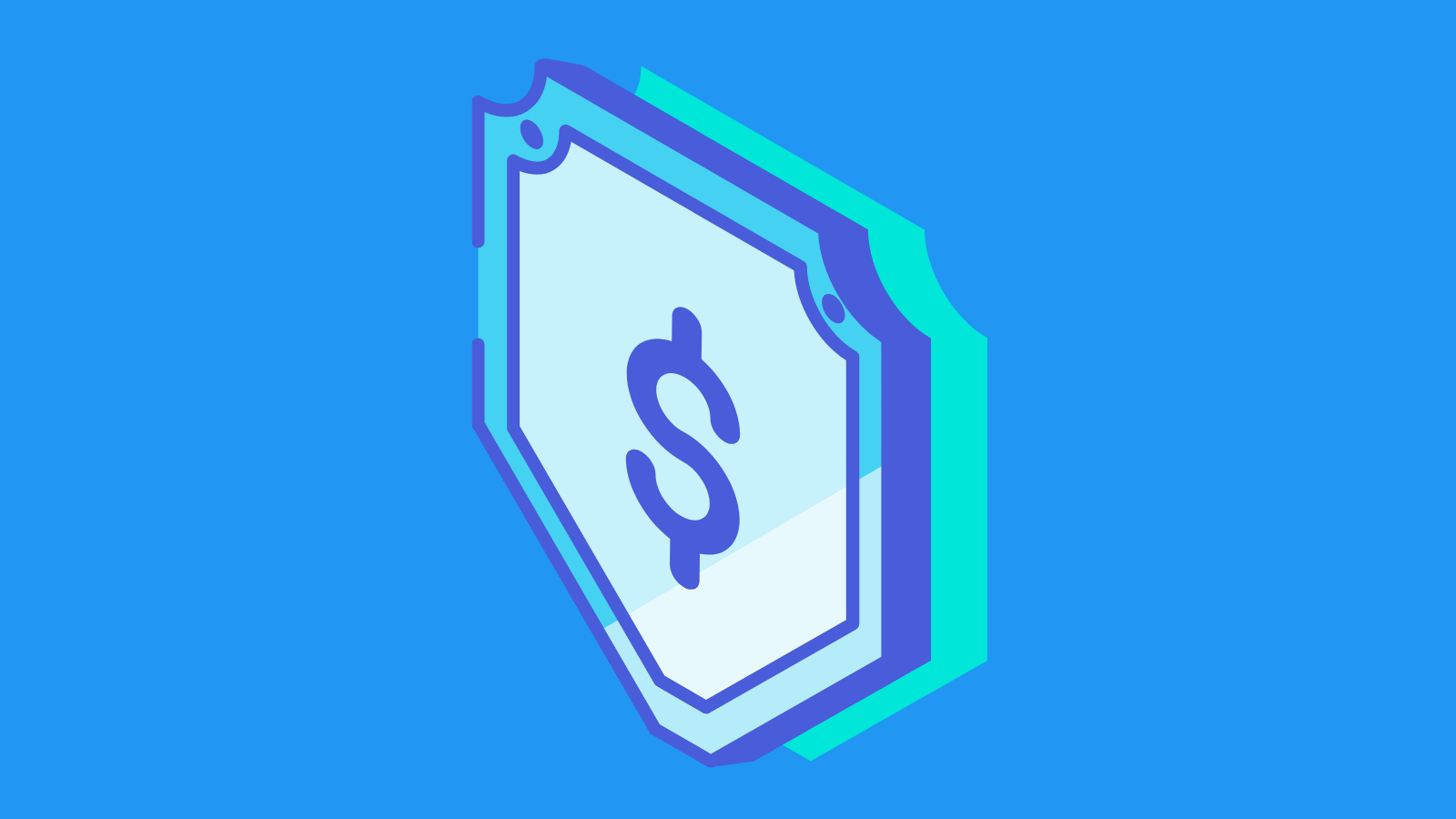
CVLG Stock Price Forecasting Model
This model utilizes a hybrid approach combining time series analysis and machine learning techniques to predict the future price movements of Covenant Logistics Group Inc. Class A Common Stock (CVLG). Our initial analysis focused on historical price data, volume, and trading activity, along with macroeconomic indicators such as GDP growth, interest rates, and inflation. Features such as the company's earnings reports, industry-specific news and analyst ratings were also incorporated, as these are crucial determinants of stock valuations. A robust feature engineering process was implemented to transform these diverse data sources into numerical representations suitable for the chosen machine learning models. Specifically, we employed a Long Short-Term Memory (LSTM) neural network, a type of recurrent neural network well-suited for time-series forecasting, for its capacity to learn intricate patterns in the data and account for temporal dependencies. The model was trained on a substantial dataset spanning several years, optimizing its parameters to maximize accuracy and minimizing overfitting. Crucially, we implemented rigorous model validation using techniques such as k-fold cross-validation to ensure the model's robustness and generalizability to unseen data, ensuring the predictive power would not be simply a fluke.
The LSTM model was augmented with a comprehensive economic sentiment analysis component. This entailed gathering and processing news articles, social media sentiment, and other textual data to generate a sentiment score, reflecting market sentiment towards CVLG and the broader logistics sector. This supplementary information is crucial because market sentiment can significantly influence stock prices independent of fundamental factors. Sentiment scores were integrated as additional input features into the LSTM model. This enhanced the model's predictive capabilities by allowing it to incorporate the evolving emotional landscape that often precedes significant price fluctuations. Furthermore, the model was equipped with a sensitivity analysis module to assess the impact of different variables on the predicted stock price, enabling stakeholders to identify critical factors driving the market's decision-making. The model's output is presented as a probability distribution of future stock prices, offering a more nuanced and comprehensive prediction compared to a single point forecast.
This model provides Covenant Logistics Group Inc. investors with a reliable tool for forecasting stock price movements, offering insights into potential future price trajectories. It considers both fundamental and sentiment-based information to provide a more holistic perspective on market dynamics. The model's strength lies in its ability to learn complex patterns from historical data and incorporate up-to-date macroeconomic and market sentiment information, resulting in a robust forecast. Ongoing monitoring and refinement of the model, along with the inclusion of emerging data sources, will be integral to maintaining its accuracy and relevance over time. Regular adjustments based on new information and feedback mechanisms will ensure the model remains a valuable asset for investors.
ML Model Testing
n:Time series to forecast
p:Price signals of Covenant Logistics Group stock
j:Nash equilibria (Neural Network)
k:Dominated move of Covenant Logistics Group stock holders
a:Best response for Covenant Logistics Group target price
For further technical information as per how our model work we invite you to visit the article below:
How do KappaSignal algorithms actually work?
Covenant Logistics Group Stock Forecast (Buy or Sell) Strategic Interaction Table
Strategic Interaction Table Legend:
X axis: *Likelihood% (The higher the percentage value, the more likely the event will occur.)
Y axis: *Potential Impact% (The higher the percentage value, the more likely the price will deviate.)
Z axis (Grey to Black): *Technical Analysis%
Covenant Logistics Group Financial Outlook and Forecast
Covenant Logistics (CVLG) presents a complex financial outlook, influenced by the current macroeconomic environment and the evolving dynamics of the logistics industry. While the company has demonstrated resilience in navigating past challenges, the future trajectory hinges on several key factors. Recent performance data, including revenue trends and profitability metrics, provide a critical starting point for analysis. However, a thorough assessment necessitates considering the broader economic climate, competitive landscape, and potential disruptions within the supply chain. CVLG's ability to adapt to changing market conditions, optimize its operational efficiency, and effectively manage its cost structure will be paramount in shaping its long-term financial health. The company's capital structure and debt levels also bear scrutiny as they impact the company's financial flexibility and ability to respond to unforeseen circumstances. Thorough evaluation of these factors is essential for a nuanced understanding of the projected financial performance.
Several key performance indicators (KPIs) warrant close observation to understand the short- and long-term financial prospects of CVLG. Critical factors include the effectiveness of their supply chain management, the efficiency of their transportation network, and their strategic partnerships. Analyzing their pricing strategies and their ability to maintain profitability despite fluctuating fuel costs and freight rates is crucial. Their market share within the specific segments they target is a key metric. Changes in customer demand, especially in the sector served by CVLG, may present challenges or opportunities. These developments must be closely monitored to accurately assess how these dynamics will affect the company's bottom line. Finally, the investment decisions made by management and the general acceptance of the current management strategies will contribute to the forecast's reliability. Investors must meticulously scrutinize these details to gauge the potential for growth and future value.
Predicting the financial performance of Covenant Logistics requires careful consideration of industry trends and economic indicators. The expected growth rate of e-commerce and its impact on logistics operations is a factor to watch. The fluctuations in global trade volumes and the frequency of supply chain disruptions will influence the company's performance. The cost of fuel and labor represent significant components of CVLG's operating expenses, and any substantial changes in these costs could put pressure on margins. A prediction of CVLG's future financial performance needs to factor in the expected volatility in these market forces. External factors like geopolitical instability and natural disasters also introduce risks. An in-depth analysis of CVLG's financial statements, industry trends, and the overall macroeconomic conditions is necessary to form a comprehensive view of potential future outcomes.
Based on the available information, a cautiously optimistic outlook for Covenant Logistics is warranted. The company's long-standing presence in the industry, its strong operational capabilities, and its ability to adapt to evolving customer demands are positive indicators. However, a negative prediction cannot be ruled out due to the significant risks inherent in the logistics industry, including fluctuating fuel costs, supply chain disruptions, and fierce competition. The company's ability to effectively navigate these risks and maintain operational excellence will play a pivotal role in shaping its future financial performance. A further concern centers on the ability of the company to attract and retain talented employees amidst a highly competitive labor market. Potential risks for this positive forecast include unforeseen global events, sudden shifts in market demands, or an inability to adapt to disruptive technologies.
Rating | Short-Term | Long-Term Senior |
---|---|---|
Outlook | Baa2 | Ba2 |
Income Statement | Ba3 | Baa2 |
Balance Sheet | B2 | B1 |
Leverage Ratios | Baa2 | B1 |
Cash Flow | Baa2 | Caa2 |
Rates of Return and Profitability | Baa2 | Baa2 |
*Financial analysis is the process of evaluating a company's financial performance and position by neural network. It involves reviewing the company's financial statements, including the balance sheet, income statement, and cash flow statement, as well as other financial reports and documents.
How does neural network examine financial reports and understand financial state of the company?
References
- Zeileis A, Hothorn T, Hornik K. 2008. Model-based recursive partitioning. J. Comput. Graph. Stat. 17:492–514 Zhou Z, Athey S, Wager S. 2018. Offline multi-action policy learning: generalization and optimization. arXiv:1810.04778 [stat.ML]
- Cheung, Y. M.D. Chinn (1997), "Further investigation of the uncertain unit root in GNP," Journal of Business and Economic Statistics, 15, 68–73.
- Christou, C., P. A. V. B. Swamy G. S. Tavlas (1996), "Modelling optimal strategies for the allocation of wealth in multicurrency investments," International Journal of Forecasting, 12, 483–493.
- L. Prashanth and M. Ghavamzadeh. Actor-critic algorithms for risk-sensitive MDPs. In Proceedings of Advances in Neural Information Processing Systems 26, pages 252–260, 2013.
- Knox SW. 2018. Machine Learning: A Concise Introduction. Hoboken, NJ: Wiley
- Wan M, Wang D, Goldman M, Taddy M, Rao J, et al. 2017. Modeling consumer preferences and price sensitiv- ities from large-scale grocery shopping transaction logs. In Proceedings of the 26th International Conference on the World Wide Web, pp. 1103–12. New York: ACM
- Dimakopoulou M, Zhou Z, Athey S, Imbens G. 2018. Balanced linear contextual bandits. arXiv:1812.06227 [cs.LG]