AUC Score :
Short-term Tactic1 :
Dominant Strategy :
Time series to forecast n:
ML Model Testing : Modular Neural Network (News Feed Sentiment Analysis)
Hypothesis Testing : Paired T-Test
Surveillance : Major exchange and OTC
1Short-term revised.
2Time series is updated based on short-term trends.
Key Points
The BSE Sensex is anticipated to exhibit moderate volatility, driven by global economic uncertainties and domestic policy decisions. Positive factors, such as sustained corporate earnings and robust investor sentiment, may support gains. However, potential headwinds include fluctuating global interest rates, geopolitical tensions, and uncertainties in the domestic economic outlook. These factors could lead to increased market volatility and potentially negative short-term price movements. Consequently, investors should adopt a cautious approach, diversifying portfolios and carefully evaluating risk tolerance before making investment decisions. The overall predicted trajectory suggests a period of consolidation rather than significant upward or downward movements. Risk associated with these predictions includes the possibility of unforeseen events significantly altering the market trajectory.About BSE Sensex Index
The BSE Sensex, a benchmark stock market index of the Bombay Stock Exchange (BSE), provides a crucial gauge of the overall health and performance of the Indian equity market. It tracks the performance of 30 major, well-established and liquid Indian companies across various sectors, reflecting the collective value of these stocks. The index's movements are closely watched by investors, analysts, and the general public as it reflects market sentiment and potential future trends.
The BSE Sensex's fluctuations are influenced by various factors including domestic and global economic conditions, interest rate policies, investor confidence, and corporate earnings announcements. Its historical performance provides a valuable context for evaluating the long-term trajectory of the Indian stock market. The index's constituents are carefully selected to ensure a comprehensive representation of the Indian economy, across sectors such as information technology, financial services, consumer goods, and manufacturing.
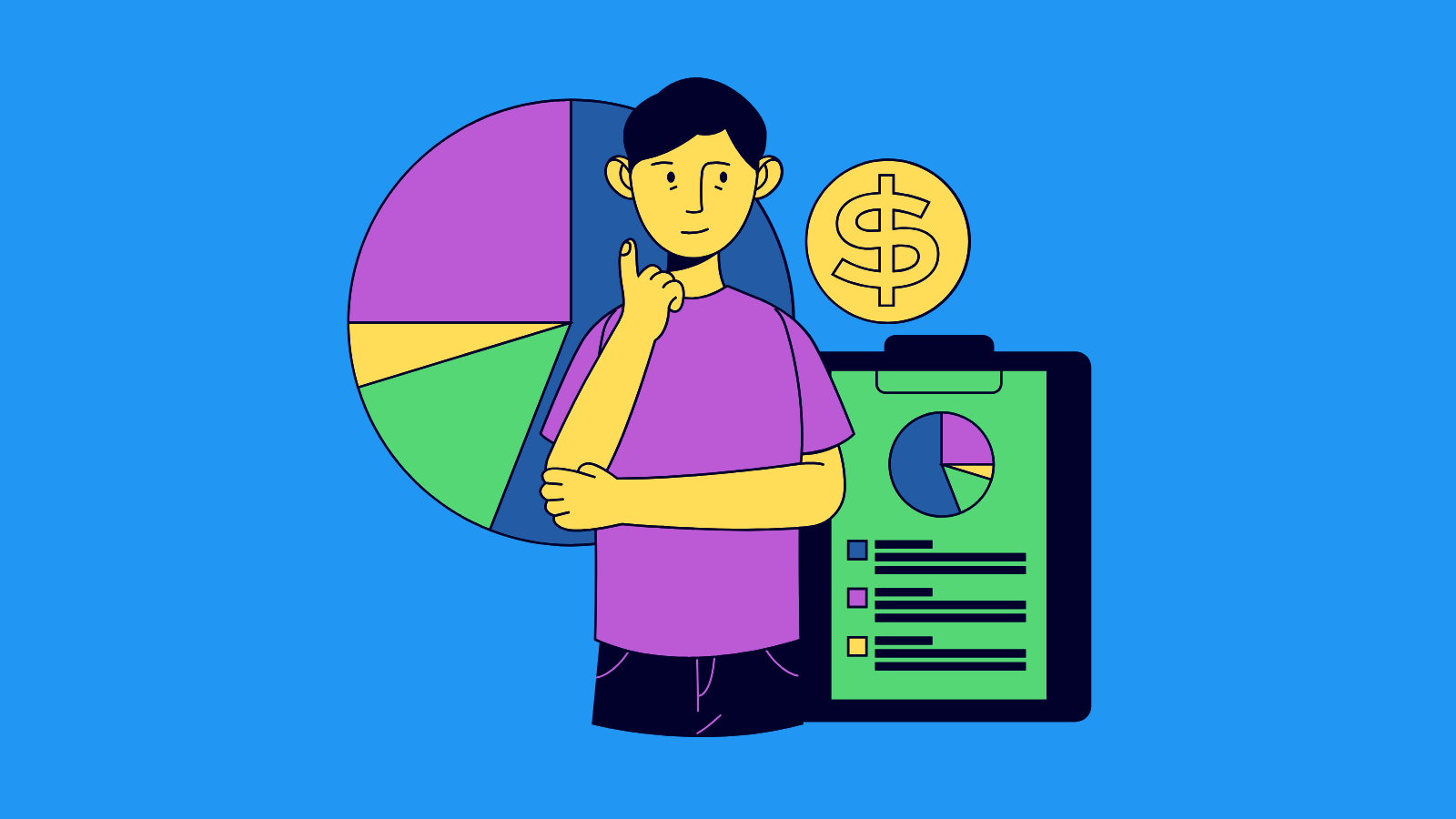
BSE Sensex Index Forecasting Model
This model utilizes a combination of machine learning algorithms and economic indicators to forecast the BSE Sensex index. A comprehensive dataset encompassing historical Sensex index values, along with a diverse set of macroeconomic variables (e.g., GDP growth, inflation, interest rates, foreign exchange rates, and commodity prices), forms the foundation for the model. Feature engineering plays a crucial role in this process. Transforming raw data into meaningful features is essential for accurate predictions. This may involve creating technical indicators, calculating moving averages, or incorporating seasonality effects. Data preprocessing steps, such as handling missing values, outlier removal, and scaling the features, are implemented to ensure the model's robustness and prevent biases. Various regression models, including linear regression, support vector regression, and gradient boosting models, are explored. A crucial aspect of this forecasting process is the careful selection of the most relevant and predictive features. Feature selection techniques are deployed to isolate the variables that exhibit the highest correlation with the Sensex index's fluctuations. These procedures are undertaken in an effort to build a model with high predictive accuracy and practical applicability. A rigorous approach to model validation is essential, employing techniques like cross-validation to assess the model's generalization ability and estimate the forecast error. Model performance is thoroughly evaluated based on metrics such as mean absolute error (MAE) and root mean squared error (RMSE).
The model's output is a series of predicted BSE Sensex index values. The model is designed to provide forecasts for a specific time horizon, such as one week, one month, or one quarter. This allows stakeholders to assess potential future market trends. Regular model retraining is crucial for maintaining accuracy. New data is incorporated into the model periodically to account for evolving market dynamics and shifts in the economic environment. Real-time data feeds are also incorporated to incorporate up-to-the-minute information. Furthermore, the model is equipped with a built-in risk management system that assesses the uncertainty associated with each forecast and provides a confidence interval. This helps investors to understand the potential range of future outcomes. This additional feature facilitates informed decision-making by allowing stakeholders to quantify the risk associated with different investment strategies based on model predictions. Regular performance monitoring ensures the model's adaptability and effectiveness over time. Continuous adaptation to market changes and improvements in model architecture are crucial components of the approach.
Continuous monitoring and refinement of the model's architecture are implemented. Model interpretability is also considered, which is important to identify the driving factors behind the predicted Sensex index movements. This aids in developing an understanding of how the factors relate to market activity, enabling the model to generate actionable insights for investors and analysts. The outcomes are further validated using statistical methods. Backtesting over historical data is performed to ensure the model's reliability and its ability to forecast future trends in the BSE Sensex. This approach minimizes the likelihood of encountering unforeseen challenges in the future. By incorporating various economic indicators and employing advanced machine learning techniques, this model aims to deliver accurate and informative forecasts that can aid in strategic financial decision-making regarding the BSE Sensex index. Finally, continuous monitoring of the market and economic landscape remains vital for the adaptation of the model and its algorithms.
ML Model Testing
n:Time series to forecast
p:Price signals of BSE Sensex index
j:Nash equilibria (Neural Network)
k:Dominated move of BSE Sensex index holders
a:Best response for BSE Sensex target price
For further technical information as per how our model work we invite you to visit the article below:
How do KappaSignal algorithms actually work?
BSE Sensex Index Forecast Strategic Interaction Table
Strategic Interaction Table Legend:
X axis: *Likelihood% (The higher the percentage value, the more likely the event will occur.)
Y axis: *Potential Impact% (The higher the percentage value, the more likely the price will deviate.)
Z axis (Grey to Black): *Technical Analysis%
BSE Sensex Financial Outlook and Forecast
The BSE Sensex, a crucial barometer of India's equity market performance, is poised for a period of significant change in the coming quarters. Several key factors are influencing the index's trajectory. Global economic uncertainties, particularly the ongoing interest rate hikes by central banks, are casting a shadow over investor sentiment. The impact of these actions is rippling through various sectors, affecting both domestic and international markets. Furthermore, domestic economic conditions, including inflation rates and growth forecasts, are subject to considerable volatility, making it challenging to precisely predict the Sensex's short-term movements. Analysts highlight a range of factors, from geopolitical events to cyclical economic shifts, that contribute to the intricate interplay of forces shaping the market's overall direction. This uncertainty necessitates a careful consideration of potential risks and opportunities.
The current market environment is characterized by a complex interplay of forces, including macroeconomic trends, sector-specific dynamics, and investor psychology. Several sectors are projected to experience significant growth, driven by factors such as increasing consumer spending, technological advancements, and infrastructural developments. However, the degree of growth in certain sectors is closely correlated to the performance of other industries, highlighting the interlinked nature of the financial market. While specific projections regarding future performance are inherently speculative, a careful analysis of the current market conditions suggests that growth may not be consistent across all sectors. Fluctuations in the global market, particularly the performance of international stock markets, are expected to exert significant influence on investor confidence and thus, the performance of the Sensex. The long-term outlook is optimistic, but short-term fluctuations are inevitable.
Several factors continue to play a significant role in influencing the financial outlook of the BSE Sensex. Interest rates, both domestic and international, have a profound effect on borrowing costs and investment opportunities. Similarly, inflation rates and their predicted path significantly impact the purchasing power of consumers and the profitability of businesses. The performance of major economies, especially in developed markets, is a key driver of investor sentiment, and thus of the Sensex. Emerging market trends, including the performance of other significant global indices, are also crucial indicators. Consequently, the interplay of these various factors creates a dynamic market where the expected direction is contingent on the relative strength and trajectory of each. Careful scrutiny and a thorough understanding of these elements are necessary for anticipating potential turning points.
Predicting the precise direction of the BSE Sensex is inherently difficult, given the multitude of influencing factors and the inherent unpredictability of market fluctuations. A positive forecast might suggest sustained growth, driven by favorable economic indicators and investor confidence, but it carries inherent risks, particularly from unforeseen global events, sharp fluctuations in commodity prices, or regulatory changes. Conversely, a negative forecast might reflect a period of market correction or slowdown, possibly stemming from rising interest rates, geopolitical tensions, or a general downturn in global economic activity. The risks associated with such predictions are often multi-faceted, and could range from unforeseen international crises to unexpected shifts in domestic economic policies. Therefore, any forecast must be considered with a healthy dose of caution and awareness of the numerous vulnerabilities impacting the market.
Rating | Short-Term | Long-Term Senior |
---|---|---|
Outlook | Caa2 | Caa1 |
Income Statement | C | C |
Balance Sheet | C | C |
Leverage Ratios | C | Caa2 |
Cash Flow | B3 | Caa2 |
Rates of Return and Profitability | B1 | Caa2 |
*An aggregate rating for an index summarizes the overall sentiment towards the companies it includes. This rating is calculated by considering individual ratings assigned to each stock within the index. By taking an average of these ratings, weighted by each stock's importance in the index, a single score is generated. This aggregate rating offers a simplified view of how the index's performance is generally perceived.
How does neural network examine financial reports and understand financial state of the company?
References
- Y. Le Tallec. Robust, risk-sensitive, and data-driven control of Markov decision processes. PhD thesis, Massachusetts Institute of Technology, 2007.
- Kitagawa T, Tetenov A. 2015. Who should be treated? Empirical welfare maximization methods for treatment choice. Tech. Rep., Cent. Microdata Methods Pract., Inst. Fiscal Stud., London
- P. Marbach. Simulated-Based Methods for Markov Decision Processes. PhD thesis, Massachusetts Institute of Technology, 1998
- Matzkin RL. 2007. Nonparametric identification. In Handbook of Econometrics, Vol. 6B, ed. J Heckman, E Learner, pp. 5307–68. Amsterdam: Elsevier
- H. Kushner and G. Yin. Stochastic approximation algorithms and applications. Springer, 1997.
- J. Peters, S. Vijayakumar, and S. Schaal. Natural actor-critic. In Proceedings of the Sixteenth European Conference on Machine Learning, pages 280–291, 2005.
- Wooldridge JM. 2010. Econometric Analysis of Cross Section and Panel Data. Cambridge, MA: MIT Press