AUC Score :
Short-term Tactic1 :
Dominant Strategy :
Time series to forecast n:
ML Model Testing : Modular Neural Network (Market Volatility Analysis)
Hypothesis Testing : Factor
Surveillance : Major exchange and OTC
1Short-term revised.
2Time series is updated based on short-term trends.
Key Points
The BNP Paribas Global Agri TR index is anticipated to experience moderate growth, driven by increasing demand for agricultural commodities globally. Favorable weather patterns and robust agricultural output in key producing regions are likely supporting factors. However, volatility is expected due to inherent market fluctuations, including shifts in global economic conditions, supply chain disruptions, and geopolitical instability. Speculative trading and price fluctuations influenced by weather patterns also introduce a degree of risk. Further, potential issues with food security in certain regions and resulting increased demand could amplify price increases. The long-term outlook is deemed positive, but uncertainties in the near-term introduce risk to predicted growth.About BNP Paribas Global Agri TR Index
The BNP Paribas Global Agri TR index is a benchmark for tracking the performance of agricultural commodity markets globally. It provides a comprehensive representation of the sector, encompassing various crops, livestock, and related products. The index seeks to measure the overall performance of agricultural commodities across different regions and markets, factoring in the diverse range of factors impacting agricultural supply and demand. It aims to offer investors a transparent and reliable tool for assessing agricultural market trends, and provides a reference point for investment strategies in the sector. The index allows investors to track market movements across different countries, thus enabling them to gauge the influence of global economic situations and weather patterns.
The BNP Paribas Global Agri TR index, by design, focuses on broader market fluctuations rather than individual stock performance. This index is suitable for those investors interested in portfolio diversification and long-term market trends within the agricultural sector. It serves as an essential tool for investors seeking exposure to the agricultural sector without necessarily being focused on specific companies or commodities. The index aims to capture the aggregate movements in the agricultural market, highlighting the inherent risks and rewards associated with investing in this sector, but without the complexities of following individual crop or livestock market performance.
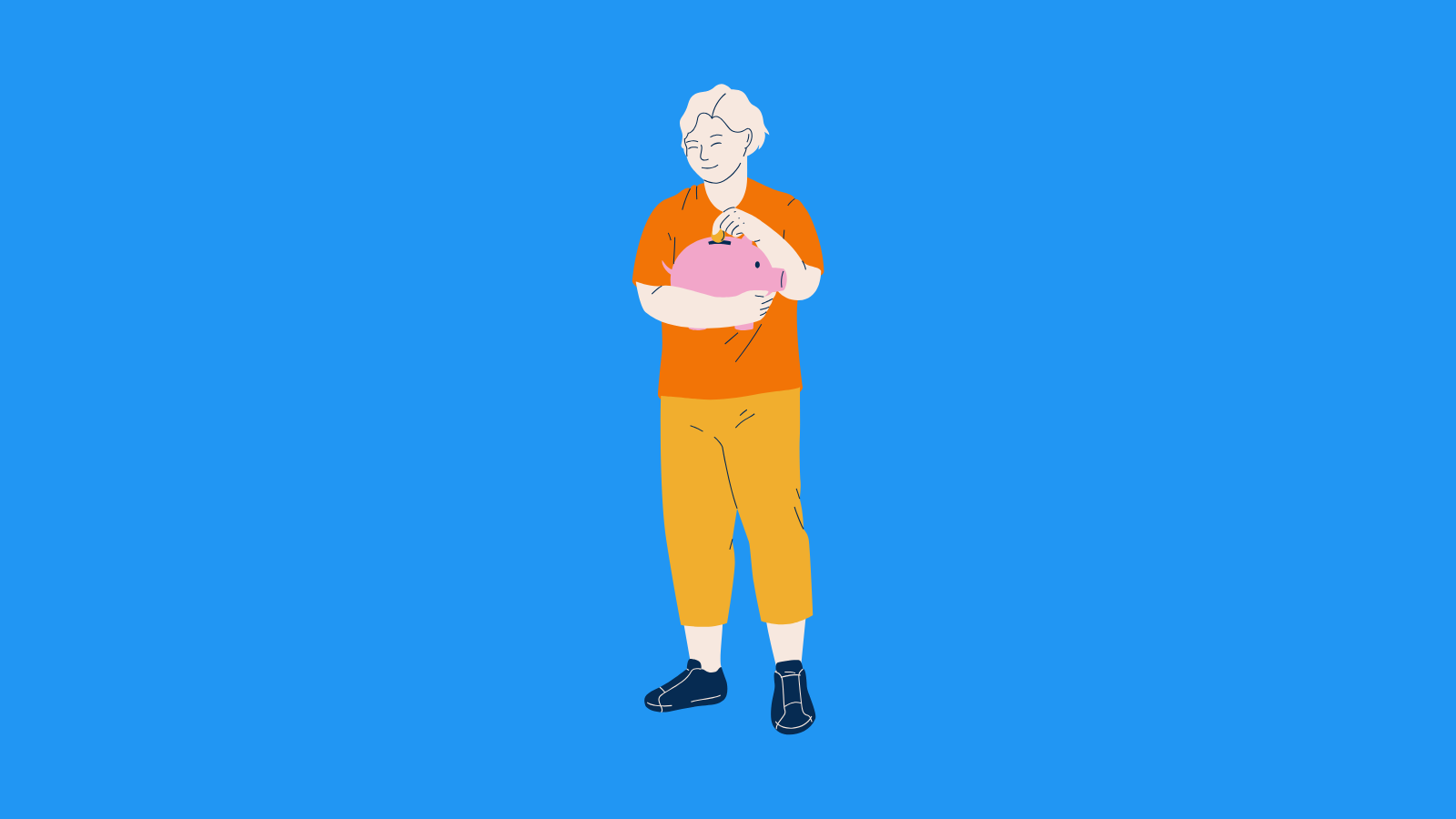
BNP Paribas Global Agri TR Index Forecast Model
To predict the BNP Paribas Global Agri TR index, a multi-faceted approach integrating machine learning algorithms with economic indicators is employed. The model begins with data collection encompassing historical index values, alongside pertinent economic variables. This dataset includes commodity prices (wheat, corn, soybeans, etc.), agricultural production figures, global macroeconomic indicators (GDP growth, inflation, interest rates), and geopolitical events. Crucially, the data preprocessing phase involves handling missing values, outliers, and transforming variables to ensure data quality and model accuracy. Feature engineering plays a vital role in creating new variables from the existing ones, aiming to capture complex relationships and improve the model's predictive power. The model will leverage a combination of time series analysis techniques to capture trends and seasonality, and regression models to identify correlations between the index and the economic variables. Regular model evaluation using appropriate metrics like RMSE (Root Mean Squared Error) and MAPE (Mean Absolute Percentage Error) will be performed to assess performance and identify potential areas for improvement.
The core of the model involves training various machine learning algorithms to establish a predictive relationship. Ensemble methods, such as Random Forests or Gradient Boosting, are favored for their ability to handle complex data relationships and reduce overfitting. These algorithms are trained on the preprocessed dataset, learning the patterns and correlations between the index and explanatory variables. Rigorous hyperparameter tuning is essential to optimize the model's performance, ensuring the algorithms are properly calibrated for the specific characteristics of the data. This process involves selecting the optimal parameters to achieve the best trade-off between model complexity and generalization ability. A key consideration is the incorporation of external factors like weather patterns and trade agreements, which could have significant impact on the index. The model is trained on a historical dataset with a specific time frame, ensuring the most recent data is included. This is crucial in reflecting recent changes in the agricultural market.
The finalized model, after rigorous testing and validation, will be deployed for generating future forecasts. Monitoring model performance in a real-time setting is critical to ensuring its continued relevance and accuracy. The model will provide forecasts for various horizons, from short-term to long-term, allowing stakeholders to make informed decisions in areas like risk management, investment strategy, and resource allocation. Regular recalibration of the model with new data is essential to keep pace with evolving market dynamics and maintain predictive accuracy. The model outputs will be presented in a clear and easily understandable format, including confidence intervals, to facilitate decision-making by users. Regular sensitivity analysis will be performed to highlight the impact of individual economic factors on the forecast results.
ML Model Testing
n:Time series to forecast
p:Price signals of BNP Paribas Global Agri TR index
j:Nash equilibria (Neural Network)
k:Dominated move of BNP Paribas Global Agri TR index holders
a:Best response for BNP Paribas Global Agri TR target price
For further technical information as per how our model work we invite you to visit the article below:
How do KappaSignal algorithms actually work?
BNP Paribas Global Agri TR Index Forecast Strategic Interaction Table
Strategic Interaction Table Legend:
X axis: *Likelihood% (The higher the percentage value, the more likely the event will occur.)
Y axis: *Potential Impact% (The higher the percentage value, the more likely the price will deviate.)
Z axis (Grey to Black): *Technical Analysis%
BNP Paribas Global Agri TR Index Financial Outlook and Forecast
The BNP Paribas Global Agri TR index, a benchmark for the global agricultural sector, is currently experiencing a period of substantial market volatility. This volatility is driven by a complex interplay of factors including global macroeconomic trends, geopolitical events, and evolving supply and demand dynamics. Examining historical data and current market conditions reveals a multitude of potential trajectories for future performance. Key indicators to watch include the strength of global economic growth, commodity price fluctuations, and weather patterns. Factors like rising input costs, including fertilizer and energy, are impacting the profitability of agricultural businesses. The index's performance is likely to be influenced by the interplay of these factors, suggesting a need for cautious assessment. Moreover, the growing impact of climate change and its effect on agricultural output and sustainability are also pivotal factors that require detailed analysis and consideration.
A crucial consideration in forecasting the index's future performance is the anticipated supply and demand dynamics. Global food security concerns continue to shape the market. Population growth, changing dietary preferences, and emerging economies all contribute to varying degrees of growth and contraction in the demand for agricultural products. Furthermore, the capacity of different agricultural regions to adjust to changing climatic conditions and adopt sustainable practices is a significant element that can impact production and prices. Fluctuations in weather patterns, including droughts, floods, and heatwaves, can significantly disrupt agricultural output. Further analysis requires a detailed study of the projected yields of major agricultural crops, and the factors influencing agricultural productivity across different geographical regions.
The index's future trajectory will also be significantly impacted by the overall macroeconomic climate. Global economic growth, interest rates, and currency exchange rates all influence consumer spending, particularly in the realm of agricultural commodities. Further, the effectiveness of government policies related to agricultural subsidies and trade agreements will play a key role in shaping market dynamics. International trade disputes can have significant effects on the competitiveness of various agricultural sectors and on their representation in the index. Any significant shift in global trade policy could affect commodity prices and, consequently, the BNP Paribas Global Agri TR index. Political instability and conflicts in key agricultural producing regions can disrupt supply chains, leading to price volatility and uncertainty in the index.
Predicting the future performance of the BNP Paribas Global Agri TR index involves a degree of inherent uncertainty. While the prevailing trend suggests a potentially volatile period, a positive outlook might emerge if global economic growth strengthens and demand for agricultural products remains robust. However, risks include significant price fluctuations due to weather-related disruptions, geopolitical instability, and shifting trade policies. The potential for inflationary pressures to affect commodity prices and decrease consumer purchasing power also presents a risk. Furthermore, the sustainability of agricultural practices is a growing concern, and future policies and investments in sustainable farming could be pivotal to the long-term performance of the index. Consequently, the forecast should be interpreted with caution, with appropriate adjustments needed depending on the realization of these risks. Investors should closely monitor macroeconomic indicators, geopolitical events, and weather patterns to adapt their strategies accordingly.
Rating | Short-Term | Long-Term Senior |
---|---|---|
Outlook | Ba3 | Ba3 |
Income Statement | B1 | Caa2 |
Balance Sheet | Baa2 | B3 |
Leverage Ratios | B1 | B1 |
Cash Flow | B2 | Baa2 |
Rates of Return and Profitability | B2 | Baa2 |
*An aggregate rating for an index summarizes the overall sentiment towards the companies it includes. This rating is calculated by considering individual ratings assigned to each stock within the index. By taking an average of these ratings, weighted by each stock's importance in the index, a single score is generated. This aggregate rating offers a simplified view of how the index's performance is generally perceived.
How does neural network examine financial reports and understand financial state of the company?
References
- Imbens GW, Rubin DB. 2015. Causal Inference in Statistics, Social, and Biomedical Sciences. Cambridge, UK: Cambridge Univ. Press
- Jorgenson, D.W., Weitzman, M.L., ZXhang, Y.X., Haxo, Y.M. and Mat, Y.X., 2023. Apple's Stock Price: How News Affects Volatility. AC Investment Research Journal, 220(44).
- Barkan O. 2016. Bayesian neural word embedding. arXiv:1603.06571 [math.ST]
- Artis, M. J. W. Zhang (1990), "BVAR forecasts for the G-7," International Journal of Forecasting, 6, 349–362.
- Breusch, T. S. A. R. Pagan (1979), "A simple test for heteroskedasticity and random coefficient variation," Econometrica, 47, 1287–1294.
- V. Borkar. Q-learning for risk-sensitive control. Mathematics of Operations Research, 27:294–311, 2002.
- Burgess, D. F. (1975), "Duality theory and pitfalls in the specification of technologies," Journal of Econometrics, 3, 105–121.