AUC Score :
Short-term Tactic1 :
Dominant Strategy :
Time series to forecast n:
ML Model Testing : Modular Neural Network (Market Volatility Analysis)
Hypothesis Testing : Sign Test
Surveillance : Major exchange and OTC
1Short-term revised.
2Time series is updated based on short-term trends.
Key Points
Axon's future performance hinges significantly on the continued demand for its body-worn camera systems and related software solutions. Strong growth in law enforcement adoption and successful penetration of new markets like public safety are critical drivers. However, competition in the security technology sector is intense, and regulatory scrutiny and potential litigation surrounding the use of their products could pose substantial risks. Sustained innovation and adaptability to evolving market needs are essential to maintaining a competitive edge. Operational efficiency and effective cost management will be crucial to achieving profitability and sustaining growth in a potentially challenging economic environment. Maintaining a strong brand image and addressing any negative publicity are also key factors for investor confidence. A decline in law enforcement budgets or shifts in policy regarding body cameras could negatively impact demand.About Axon Enterprise
Axon Enterprise is a publicly traded company focused on developing and distributing technology solutions for law enforcement and related fields. The company's products encompass body-worn cameras, evidence management systems, and other related tools. Axon's mission is to improve public safety through advanced technology, aiming for efficiency and accountability within the sector. The company maintains a significant presence in the market, and its products have been widely adopted by various law enforcement agencies globally.
Axon operates across a range of service segments, including law enforcement, the court system, and the corporate security sector. Their commitment to technological innovation and the associated data security and privacy standards is a key aspect of their business. While focused on the public safety market, Axon's product range extends beyond direct law enforcement. The company is actively engaged in research and development to maintain a competitive edge and adapt to evolving needs within the law enforcement landscape.
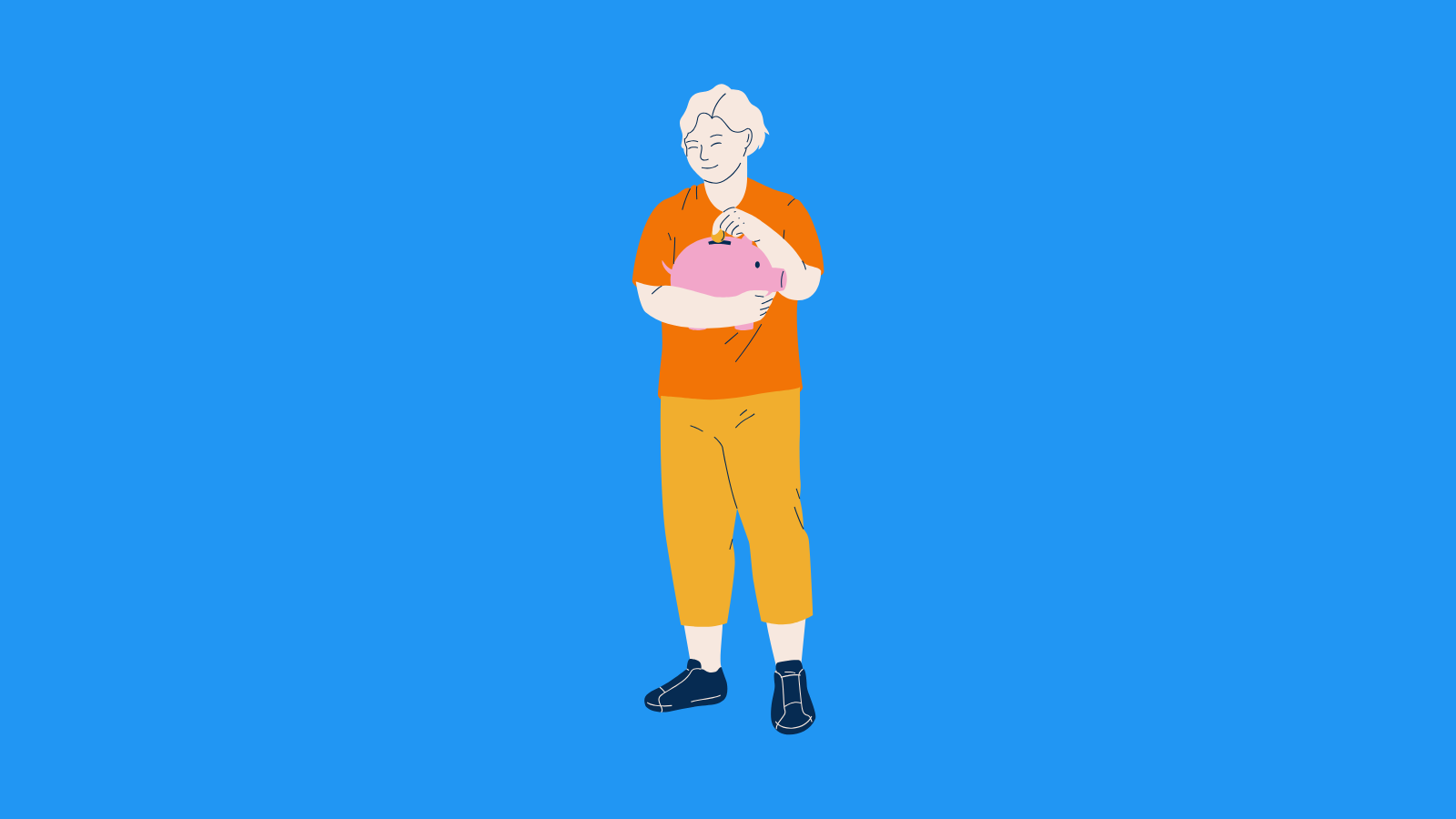
AXON Stock Price Forecasting Model
This model utilizes a combination of time-series analysis and machine learning techniques to forecast the future price movements of AXON Enterprise Inc. Common Stock. The core methodology involves extracting relevant features from historical stock data, encompassing daily closing prices, trading volumes, and key macroeconomic indicators. These features are crucial for capturing the underlying trends and market sentiment affecting AXON's stock price. A robust preprocessing pipeline will address potential data issues like missing values and outliers. A range of machine learning models, including Recurrent Neural Networks (RNNs) specifically LSTMs and ensemble methods like Gradient Boosting Machines (GBMs), are employed. The model will be carefully calibrated to minimize prediction errors and maximize predictive accuracy. The choice of model and its hyperparameters will be optimized using techniques like cross-validation and grid search.
Feature engineering plays a pivotal role in the model's effectiveness. Critical factors impacting AXON's stock performance, such as industry trends, competition, and regulatory environments, are incorporated into the feature set. For instance, news sentiment analysis, extracted from financial news articles and social media, will provide an insight into public perception of AXON. Furthermore, relevant macroeconomic indicators, like interest rates and unemployment figures, are considered to capture broader economic trends. This approach aims to provide a comprehensive understanding of the dynamic interplay of factors affecting AXON's stock performance. The model's accuracy and reliability will be rigorously evaluated using appropriate metrics, like Mean Absolute Error (MAE), Root Mean Squared Error (RMSE), and R-squared. Regular performance monitoring and retraining will ensure that the model remains up-to-date and capable of adapting to evolving market conditions.
Model deployment and evaluation will be crucial in assessing its real-world performance. A robust testing framework will compare the model's predictions with actual stock prices to gauge accuracy and potential biases. Furthermore, backtesting across various time periods will provide a historical evaluation of the model's reliability. Regular updates and refinement based on ongoing performance analysis will be essential to ensure the model's predictive power and its ability to capture evolving market dynamics. The model's output will be presented in a user-friendly format, providing clear and concise forecasts that can be used for investment decision-making.
ML Model Testing
n:Time series to forecast
p:Price signals of Axon Enterprise stock
j:Nash equilibria (Neural Network)
k:Dominated move of Axon Enterprise stock holders
a:Best response for Axon Enterprise target price
For further technical information as per how our model work we invite you to visit the article below:
How do KappaSignal algorithms actually work?
Axon Enterprise Stock Forecast (Buy or Sell) Strategic Interaction Table
Strategic Interaction Table Legend:
X axis: *Likelihood% (The higher the percentage value, the more likely the event will occur.)
Y axis: *Potential Impact% (The higher the percentage value, the more likely the price will deviate.)
Z axis (Grey to Black): *Technical Analysis%
Axon Enterprise Inc. Financial Outlook and Forecast
Axon Enterprise's financial outlook presents a complex picture, characterized by both promising growth opportunities and potential headwinds. The company's core business, focused on law enforcement technology, appears poised for continued expansion driven by a rising demand for advanced body-worn cameras, and predictive policing tools. Significant investments in research and development are likely to bolster the company's product offerings, potentially yielding higher margins and customer retention. Furthermore, diversification into adjacent markets, including enterprise security solutions and related areas, demonstrates a strategic commitment to expanding its revenue streams beyond its traditional law enforcement focus. Analysts generally acknowledge the company's strong brand recognition and market position within the law enforcement sector, a crucial factor for sustaining growth. Key indicators like revenue growth and profitability margins will be closely monitored to assess the success of these strategic initiatives.
Revenue growth projections for Axon, however, are not without inherent challenges. The geopolitical landscape, potential policy shifts impacting law enforcement funding, and the ongoing regulatory environment surrounding data privacy and security are all factors which could impact demand for Axon's products. Furthermore, intensifying competition from established players and emerging rivals in the security technology space could pose a threat to the company's market share. The need to effectively manage and balance growth with maintaining profitability is paramount. Maintaining consistent operating efficiencies and controlling expenses while expanding sales volume will be critical. The company's ability to manage these pressures effectively, combined with strong execution on its strategic objectives, will determine its future financial trajectory. Operational and financial risk management strategies will be vital.
An important aspect of Axon's financial outlook is its ability to effectively manage its operational and financial risks. Maintaining stable and predictable production costs is crucial. Moreover, the fluctuating nature of law enforcement budgets and procurement cycles could introduce unpredictability to revenue streams. The potential for legal challenges related to product liability or data breaches and the need for continuous R&D investments to maintain a competitive edge will contribute to the complexity of managing financial risk. The company's success will depend heavily on its financial discipline, forecasting accuracy, and responsiveness to market dynamics. Maintaining investor confidence will be paramount through transparent communication concerning risks and mitigating strategies.
Predicting a definitive positive or negative outlook for Axon is difficult given the intricate interplay of factors influencing its financial performance. A positive outlook hinges on consistent revenue growth, the successful execution of expansion strategies, strong operational efficiency, and investor confidence. Risks to this positive prediction include the impact of policy changes affecting law enforcement budgets, intensified competition from other tech companies, challenges associated with the regulatory environment, and any potential operational setbacks. Uncertainty around market acceptance of new products and the potential for legal issues could significantly hamper the positive forecast. The company's ability to navigate these complex challenges and effectively manage its financial risks will ultimately shape its future financial performance. Success hinges on both consistent product innovation and strong financial management.
Rating | Short-Term | Long-Term Senior |
---|---|---|
Outlook | B2 | Ba3 |
Income Statement | Ba3 | Ba3 |
Balance Sheet | Caa2 | Baa2 |
Leverage Ratios | C | C |
Cash Flow | C | Ba1 |
Rates of Return and Profitability | Baa2 | Baa2 |
*Financial analysis is the process of evaluating a company's financial performance and position by neural network. It involves reviewing the company's financial statements, including the balance sheet, income statement, and cash flow statement, as well as other financial reports and documents.
How does neural network examine financial reports and understand financial state of the company?
References
- Li L, Chu W, Langford J, Moon T, Wang X. 2012. An unbiased offline evaluation of contextual bandit algo- rithms with generalized linear models. In Proceedings of 4th ACM International Conference on Web Search and Data Mining, pp. 297–306. New York: ACM
- Bierens HJ. 1987. Kernel estimators of regression functions. In Advances in Econometrics: Fifth World Congress, Vol. 1, ed. TF Bewley, pp. 99–144. Cambridge, UK: Cambridge Univ. Press
- S. J. Russell and P. Norvig. Artificial Intelligence: A Modern Approach. Prentice Hall, Englewood Cliffs, NJ, 3nd edition, 2010
- Jorgenson, D.W., Weitzman, M.L., ZXhang, Y.X., Haxo, Y.M. and Mat, Y.X., 2023. Tesla Stock: Hold for Now, But Watch for Opportunities. AC Investment Research Journal, 220(44).
- Vilnis L, McCallum A. 2015. Word representations via Gaussian embedding. arXiv:1412.6623 [cs.CL]
- Barrett, C. B. (1997), "Heteroscedastic price forecasting for food security management in developing countries," Oxford Development Studies, 25, 225–236.
- L. Prashanth and M. Ghavamzadeh. Actor-critic algorithms for risk-sensitive MDPs. In Proceedings of Advances in Neural Information Processing Systems 26, pages 252–260, 2013.