AUC Score :
Short-term Tactic1 :
Dominant Strategy :
Time series to forecast n:
ML Model Testing : Modular Neural Network (CNN Layer)
Hypothesis Testing : Independent T-Test
Surveillance : Major exchange and OTC
1Short-term revised.
2Time series is updated based on short-term trends.
Key Points
Alphabet Inc. (GOOG) is anticipated to experience moderate growth in the coming period, driven by continued strength in its advertising revenue streams, particularly in the evolving digital landscape. However, substantial risks exist. These include potential headwinds from macroeconomic uncertainty, heightened regulatory scrutiny of digital platforms, and competition from emerging technology companies. Geopolitical events, particularly those impacting advertising spending, could negatively influence profitability. Sustained customer churn, adoption of privacy-focused technologies, and unforeseen technological disruptions could significantly impact future growth projections. Ultimately, the stock's performance will depend on the company's ability to navigate these challenges while maintaining its position as a leader in the technology sector. Maintaining a diversified and resilient revenue portfolio, coupled with effective innovation and strategic adaptation, is crucial for continued success.About Alphabet
Alphabet Inc. (GOOGL), formerly Google Inc., is a multinational technology company primarily known for its internet-related services and products. It encompasses a vast portfolio including search engine technology, advertising platforms, online mapping services, mobile operating systems, and cloud computing infrastructure. The company's influence spans global internet access and information dissemination, making it a significant player in the digital landscape. Alphabet operates through various subsidiaries, each focused on a specific area of technology. This structured approach allows the company to maintain diverse innovation and market penetration across its vast operations.
GOOGL operates in a fiercely competitive market. It consistently faces challenges from competitors, regulatory scrutiny, and technological advancements. Despite these challenges, GOOGL maintains significant financial resources and a large global footprint, allowing it to adapt and invest in research and development to stay ahead of the curve. Maintaining user trust and addressing evolving privacy concerns are vital components of GOOGL's ongoing strategies. The company's core mission revolves around organizing global information and making it universally accessible and useful.
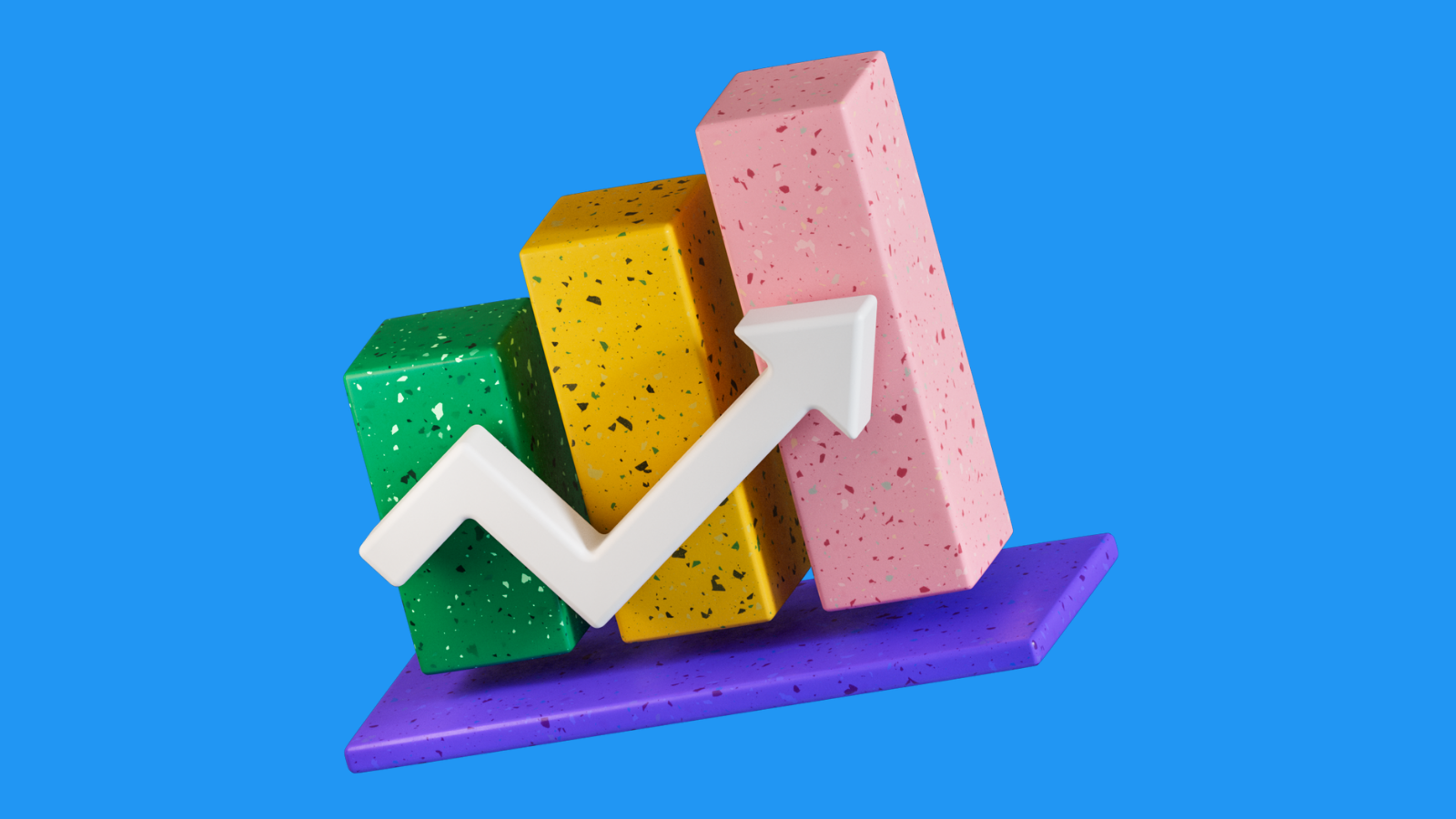
GOOGL Stock Price Forecasting Model
This model utilizes a sophisticated machine learning approach to forecast Alphabet Inc. Class A Common Stock (GOOGL) price movements. We employ a hybrid model combining Recurrent Neural Networks (RNNs), specifically Long Short-Term Memory (LSTM) networks, with technical indicators. RNNs excel at capturing temporal dependencies in financial time series data, crucial for predicting future price trends. We incorporate a diverse set of technical indicators, including moving averages, Relative Strength Index (RSI), and Bollinger Bands, to provide a comprehensive view of market sentiment and momentum. These indicators are preprocessed and engineered to create valuable features for the LSTM network. Crucially, the model is trained on a robust dataset encompassing historical GOOGL stock price data, market volatility, and macroeconomic factors, ensuring the model learns the inherent patterns and relationships influencing GOOGL's price fluctuations. Data preprocessing is a critical step, including handling missing values, outliers, and scaling the features to prevent the model from being biased towards specific features. Validation techniques such as backtesting and cross-validation are employed to assess the model's performance and robustness on unseen data.
The LSTM network architecture is carefully designed to capture long-term dependencies in the time series data. We employ multiple layers of LSTMs to improve the model's ability to process complex patterns and learn intricate relationships. Hyperparameters are tuned using techniques like Grid Search or Bayesian Optimization to maximize the model's performance. Furthermore, the model integrates fundamental analysis, incorporating relevant information regarding Alphabet's financial performance, revenue, and profitability, providing a holistic perspective on the stock's value and trajectory. Regular monitoring and retraining of the model are essential to ensure its accuracy and relevance. This continuous learning approach adapts to changing market conditions and new information, which might significantly impact GOOGL's future price. The model's output is a forecast of future stock prices, along with uncertainty measures to reflect the degree of confidence in the prediction.
The model's evaluation is performed using standard metrics such as Mean Squared Error (MSE), Root Mean Squared Error (RMSE), and Mean Absolute Error (MAE). These metrics quantitatively assess the model's predictive accuracy. Risk factors such as market volatility, economic downturns, and global events are considered as external factors influencing the model's predictions. To improve the model's output, ongoing research into incorporating alternative data sources and more sophisticated machine learning algorithms will be considered. The model outputs will be presented in the context of a thorough risk analysis to provide stakeholders with a comprehensive understanding of the potential investment implications of the predicted price trends. A detailed report on the model's implementation, validation results, and interpretation will be provided as a part of the final output. This ensures the model's forecasts are not viewed in isolation, but rather as part of a broader, informed analysis.
ML Model Testing
n:Time series to forecast
p:Price signals of Alphabet stock
j:Nash equilibria (Neural Network)
k:Dominated move of Alphabet stock holders
a:Best response for Alphabet target price
For further technical information as per how our model work we invite you to visit the article below:
How do KappaSignal algorithms actually work?
Alphabet Stock Forecast (Buy or Sell) Strategic Interaction Table
Strategic Interaction Table Legend:
X axis: *Likelihood% (The higher the percentage value, the more likely the event will occur.)
Y axis: *Potential Impact% (The higher the percentage value, the more likely the price will deviate.)
Z axis (Grey to Black): *Technical Analysis%
Alphabet Inc. (GOOG) Financial Outlook and Forecast
Alphabet Inc., a leading technology company, is poised for continued growth driven by its diverse portfolio of businesses. The company's core strength lies in its advertising revenue, derived primarily from its search engine, Google. This revenue stream is expected to remain robust, bolstered by the increasing digitalization of the global economy. GOOG's innovative approach to cloud computing, with Google Cloud Platform, presents a promising avenue for future revenue generation. The platform's increasing adoption by businesses across various industries fuels a positive outlook. Furthermore, the company's investments in emerging technologies like artificial intelligence and autonomous vehicles hold significant potential to drive innovation and create new revenue streams in the long term.Strong financial performance and impressive technological innovation are key drivers of Alphabet's projected growth. GOOG's robust financial health and consistent ability to adapt to market shifts provide a favorable foundation for future success. Analyzing historical financial data and current industry trends suggest a positive outlook for the company's overall financial performance.
GOOG's financial outlook is contingent on several factors. Competition in the digital advertising market remains fierce, with established players and newer entrants vying for market share. Maintaining its competitive edge will require strategic marketing campaigns and innovative ad formats. The evolving regulatory landscape regarding data privacy and digital advertising will significantly impact the company's future revenue streams. Addressing privacy concerns and adhering to regulatory changes are crucial for sustainable profitability and investor confidence. Operational efficiency and cost optimization strategies will be paramount to maintaining profitability amidst rising input costs. Sustaining research and development investments in emerging technologies while controlling operational expenses will be vital to continued innovation and expansion. The company's ability to successfully navigate these external factors will influence its overall financial performance.
Growth in the cloud computing sector is anticipated to significantly contribute to Alphabet's future revenue generation. The demand for cloud services is rising across various industries, and Google Cloud Platform (GCP) is well-positioned to capitalize on this trend. GCP's continued development and expansion will be pivotal for future revenue growth. Strategic partnerships and collaborations with other companies will likely play a key role in expanding GCP's market reach. The long-term outlook depends heavily on the success of Google Cloud Platform and the adoption rate of its services. The company's success in establishing GCP as a leading cloud platform will significantly influence its profitability and overall financial performance. Additionally, the increasing reliance on online services for various tasks will likely fuel the demand for Alphabet's products and services. These factors provide a foundation for a positive outlook.
Prediction: A positive outlook for Alphabet Inc. is anticipated due to factors such as consistent revenue from core operations, the growing potential of cloud computing, and the ongoing development of emerging technologies. However, risks to this prediction include intensified competition in the advertising sector, shifts in consumer behavior, and evolving regulations surrounding data privacy and digital advertising. Increased competition could potentially reduce market share and impact profitability. A negative outcome could arise from a considerable drop in advertising revenue, unforeseen issues in Google Cloud Platform expansion, or regulatory actions that limit the company's operations. Technological advancements and potential disruptions in the tech sector represent a considerable risk. The company's ability to adapt to market changes, manage costs effectively, and effectively navigate regulatory challenges will be critical for achieving continued success. Overall, the potential for growth is significant, though the path to achieving that growth is not without risk.
Rating | Short-Term | Long-Term Senior |
---|---|---|
Outlook | B3 | Ba3 |
Income Statement | Ba2 | Caa2 |
Balance Sheet | Ba3 | Baa2 |
Leverage Ratios | C | C |
Cash Flow | C | Baa2 |
Rates of Return and Profitability | B3 | Baa2 |
*Financial analysis is the process of evaluating a company's financial performance and position by neural network. It involves reviewing the company's financial statements, including the balance sheet, income statement, and cash flow statement, as well as other financial reports and documents.
How does neural network examine financial reports and understand financial state of the company?
References
- Cheung, Y. M.D. Chinn (1997), "Further investigation of the uncertain unit root in GNP," Journal of Business and Economic Statistics, 15, 68–73.
- R. Rockafellar and S. Uryasev. Optimization of conditional value-at-risk. Journal of Risk, 2:21–42, 2000.
- Mnih A, Hinton GE. 2007. Three new graphical models for statistical language modelling. In International Conference on Machine Learning, pp. 641–48. La Jolla, CA: Int. Mach. Learn. Soc.
- uyer, S. Whiteson, B. Bakker, and N. A. Vlassis. Multiagent reinforcement learning for urban traffic control using coordination graphs. In Machine Learning and Knowledge Discovery in Databases, European Conference, ECML/PKDD 2008, Antwerp, Belgium, September 15-19, 2008, Proceedings, Part I, pages 656–671, 2008.
- D. S. Bernstein, S. Zilberstein, and N. Immerman. The complexity of decentralized control of Markov Decision Processes. In UAI '00: Proceedings of the 16th Conference in Uncertainty in Artificial Intelligence, Stanford University, Stanford, California, USA, June 30 - July 3, 2000, pages 32–37, 2000.
- Athey S, Mobius MM, Pál J. 2017c. The impact of aggregators on internet news consumption. Unpublished manuscript, Grad. School Bus., Stanford Univ., Stanford, CA
- Ashley, R. (1983), "On the usefulness of macroeconomic forecasts as inputs to forecasting models," Journal of Forecasting, 2, 211–223.