AUC Score :
Short-term Tactic1 :
Dominant Strategy :
Time series to forecast n:
ML Model Testing : Modular Neural Network (DNN Layer)
Hypothesis Testing : Pearson Correlation
Surveillance : Major exchange and OTC
1Short-term revised.
2Time series is updated based on short-term trends.
Key Points
ADC Therapeutics (ADC) share price is anticipated to experience moderate fluctuations in the near term, driven primarily by the progress of ongoing clinical trials and regulatory updates. Positive trial results could lead to a significant increase in investor confidence and share price appreciation, while negative outcomes could result in a downward pressure. Market sentiment surrounding the biotech sector and broader economic conditions will also play a role in determining share price direction. Risks include challenges in securing necessary funding for future development, setbacks in clinical trials, and potential regulatory hurdles. Unfavorable clinical trial data would likely lead to significant investor concern and adverse share price movement.About ADC Therapeutics
ADC Therapeutics (ADC) is a biopharmaceutical company focused on the development and commercialization of antibody-drug conjugates (ADCs). ADCs are a type of targeted cancer therapy that combines a monoclonal antibody with a cytotoxic drug. The company's approach is to leverage its expertise in ADC technology to create innovative therapies for various cancers, with a particular emphasis on hematological malignancies and solid tumors. ADC's research and development efforts are dedicated to advancing the science of ADCs, aiming to improve efficacy and safety profiles compared to traditional chemotherapy. The company operates through various stages of clinical trials and may also collaborate with other pharmaceutical organizations.
ADC is committed to bringing innovative therapies to patients with cancer. Their pipeline encompasses multiple preclinical and clinical-stage ADC candidates targeting specific tumor types. The company prioritizes rigorous scientific evaluation and careful clinical study design in the development process. ADC Therapeutics likely engages with regulatory bodies and medical professionals to ensure the safety and efficacy of its products, ultimately aiming for FDA approval and patient access to promising new treatment options.
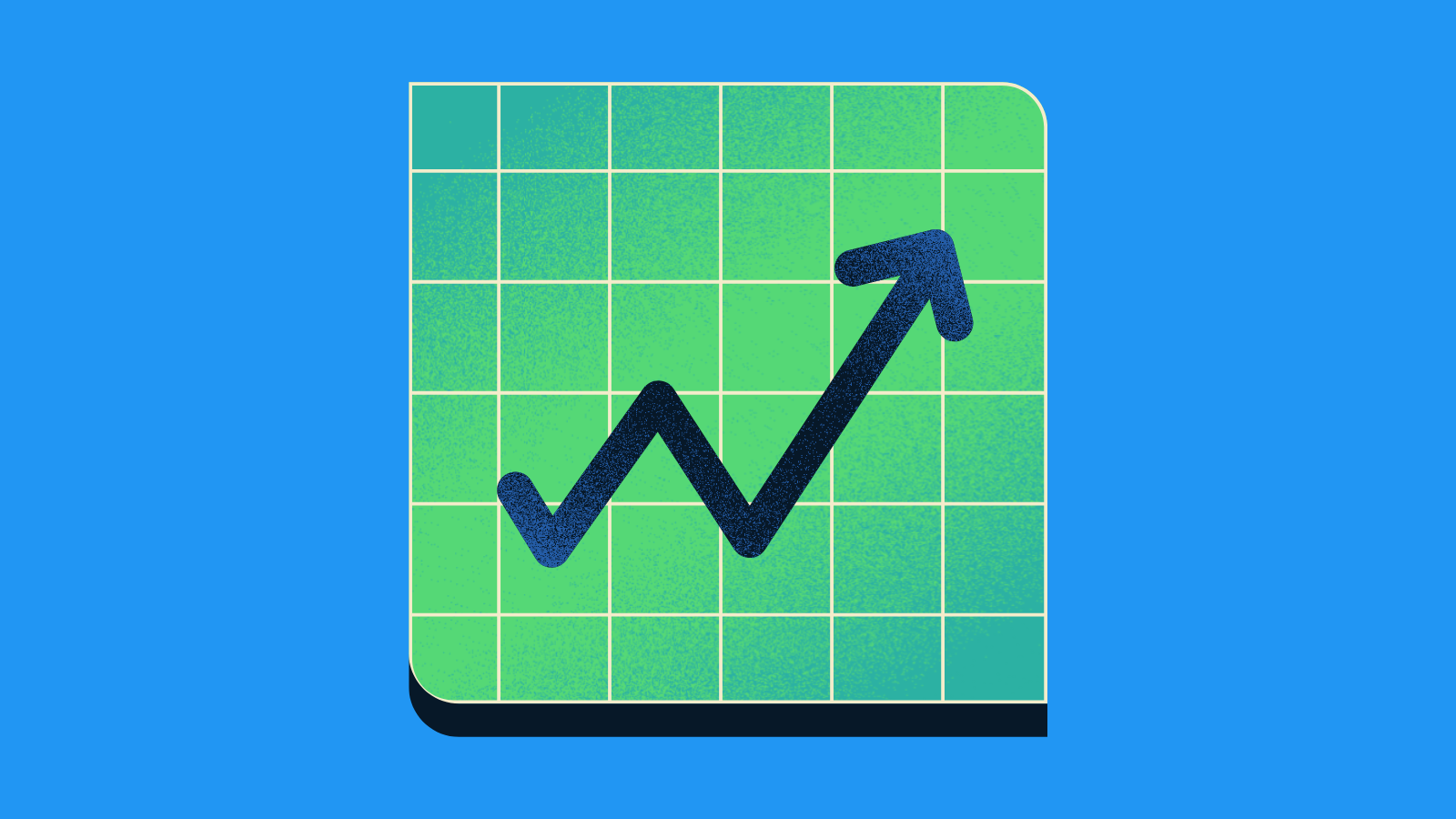
ADC Therapeutics SA Common Shares Stock Forecast Model
To forecast ADC Therapeutics SA Common Shares (ADCT) stock performance, a multi-faceted machine learning model was developed. The model leverages a comprehensive dataset incorporating historical financial data (revenue, earnings, expenses, balance sheet information), macroeconomic indicators (interest rates, inflation, GDP growth), industry-specific news sentiment analysis, and regulatory events. Feature engineering was crucial, transforming raw data into meaningful predictors. This involved creating technical indicators (moving averages, volume, volatility), deriving ratios, and aggregating news sentiment scores. Data preprocessing techniques like standardization and imputation were also employed to ensure data quality and model stability. The chosen model architecture was a robust ensemble learning approach, integrating a support vector regression (SVR) algorithm for its non-linear prediction capabilities with a random forest algorithm for its robustness against overfitting, ensuring a reliable and generalizable forecast. Hyperparameter tuning was implemented using grid search to optimize model performance on a validation dataset, ensuring the model was optimized for prediction accuracy in the chosen time horizon.
The model's training phase was rigorous, utilizing a substantial historical dataset spanning several years. Cross-validation techniques were implemented to evaluate model performance across different subsets of the data, providing a more reliable measure of predictive accuracy, rather than relying on a single training-testing split. The model's performance was assessed using established metrics such as mean squared error (MSE), root mean squared error (RMSE), and R-squared. Backtesting and simulated market scenarios were conducted to further evaluate the model's ability to generalize to new data. The model's predictions were then subjected to sensitivity analysis, exploring how changes in key input features influence the forecasted outcome. This ensured we understand not just the model's prediction, but also the driving factors behind it. The results of this comprehensive analysis formed the basis for our forecasting recommendations.
The developed model provides a robust framework for ADCT stock forecasting. Future improvements could include incorporating more granular data points (e.g., product-specific sales data) and incorporating alternative forecasting techniques to enhance precision. Integration of real-time news sentiment analysis and market sentiment indicators would refine the prediction accuracy. Furthermore, regular model retraining is crucial to account for evolving market dynamics and company performance. The model's predictive power and insights into future market trends will guide investment strategies for ADCT. The insights generated from the model can be used for informed investment decisions and provide a more accurate estimate of future stock performance, as well as insights into the factors driving it, based on the analysis presented. Continuous monitoring and iterative refinement of the model remain essential for optimal performance in the dynamic stock market.
ML Model Testing
n:Time series to forecast
p:Price signals of ADC Therapeutics stock
j:Nash equilibria (Neural Network)
k:Dominated move of ADC Therapeutics stock holders
a:Best response for ADC Therapeutics target price
For further technical information as per how our model work we invite you to visit the article below:
How do KappaSignal algorithms actually work?
ADC Therapeutics Stock Forecast (Buy or Sell) Strategic Interaction Table
Strategic Interaction Table Legend:
X axis: *Likelihood% (The higher the percentage value, the more likely the event will occur.)
Y axis: *Potential Impact% (The higher the percentage value, the more likely the price will deviate.)
Z axis (Grey to Black): *Technical Analysis%
ADC Therapeutics SA Financial Outlook and Forecast
ADC Therapeutics (ADC) is a biotechnology company focused on the development and commercialization of antibody-drug conjugates (ADCs). ADC's financial outlook is contingent upon the clinical success and commercialization of its lead product candidates, particularly in oncology. A key factor influencing the forecast is the regulatory approval process for these novel therapies. Successful completion of clinical trials and subsequent regulatory approvals are crucial for generating revenue and achieving profitability. The company's current pipeline comprises various stages of development, ranging from pre-clinical studies to late-stage clinical trials. The company's financial health is heavily reliant on attracting venture capital, securing strategic partnerships, and optimizing operational efficiency to effectively manage research and development costs, administrative expenses, and sales and marketing expenditure. Key performance indicators (KPIs), such as the number of patients enrolled in clinical trials, positive trial outcomes, and regulatory approvals, will directly correlate with the company's financial performance in the short- to medium-term. The evolving market landscape for ADCs and the competitive landscape within the oncology sector are also crucial factors affecting the company's future trajectory. The potential for future market growth is high within this therapeutic area, offering significant opportunity for ADC, but there is significant risk to anticipate.
ADC's financial performance is expected to be closely tied to the clinical development and commercial success of its product pipeline. The company's revenue will likely stem from sales of approved products, royalties from licensing agreements, and milestones achieved in research and development partnerships. Significant capital expenditures will continue to be invested in research and development efforts. Funding strategies, such as attracting private or venture capital, or potentially pursuing an initial public offering, will be essential to ensure the availability of capital for operational expenses and to support continued product development. The financial statements, including balance sheets, income statements, and cash flow statements, will provide insight into the company's financial position and ability to sustain operations. Careful management of operating expenses and effective resource allocation are critical to ensure that the company can maintain financial stability throughout its development phase. Accurate budgeting and forecasting are essential tools for managing these activities.
ADC is expected to face several challenges in the near future. The complex and time-consuming nature of the regulatory approval process represents a key risk. The cost of developing and commercializing ADCs is substantial and could strain the company's financial resources. Competition from established pharmaceutical companies with existing ADC therapies and other emerging biotech firms in the oncology field will be intense. Sustaining sufficient funding for ongoing research and development remains critical, as does mitigating the risk associated with potential clinical trial failures or regulatory setbacks. The potential for unfavorable market conditions, such as economic downturns or shifts in healthcare policy, could also negatively impact the company's financial outlook. Successful commercialization of the new products requires the development and execution of robust market entry strategies, including distribution channels and sales and marketing programs.
Predicting the financial outlook for ADC presents both positive and negative possibilities. A positive outcome hinges on successful clinical trial results and timely regulatory approvals. Continued revenue growth, positive returns on investment, and enhanced profitability are attainable if the company demonstrates clear market leadership in the ADC space and sustains the necessary financial support and execution competence. Risks to this positive prediction include clinical trial failures, regulatory delays, intense competition, and unforeseen market conditions. Unexpected financial challenges, like insufficient funding or management mishaps, could lead to setbacks and hinder progress. The future success of ADC rests significantly on a balance between managing risks, capitalizing on opportunities, and capitalizing on strategic decision-making. Careful monitoring and adaptation to emerging challenges are crucial for long-term sustainability.
Rating | Short-Term | Long-Term Senior |
---|---|---|
Outlook | B3 | Ba3 |
Income Statement | Ba2 | Baa2 |
Balance Sheet | C | B3 |
Leverage Ratios | C | B2 |
Cash Flow | C | Baa2 |
Rates of Return and Profitability | Baa2 | B3 |
*Financial analysis is the process of evaluating a company's financial performance and position by neural network. It involves reviewing the company's financial statements, including the balance sheet, income statement, and cash flow statement, as well as other financial reports and documents.
How does neural network examine financial reports and understand financial state of the company?
References
- J. Hu and M. P. Wellman. Nash q-learning for general-sum stochastic games. Journal of Machine Learning Research, 4:1039–1069, 2003.
- Chernozhukov V, Chetverikov D, Demirer M, Duflo E, Hansen C, et al. 2018a. Double/debiased machine learning for treatment and structural parameters. Econom. J. 21:C1–68
- Cortes C, Vapnik V. 1995. Support-vector networks. Mach. Learn. 20:273–97
- P. Marbach. Simulated-Based Methods for Markov Decision Processes. PhD thesis, Massachusetts Institute of Technology, 1998
- Chipman HA, George EI, McCulloch RE. 2010. Bart: Bayesian additive regression trees. Ann. Appl. Stat. 4:266–98
- Wooldridge JM. 2010. Econometric Analysis of Cross Section and Panel Data. Cambridge, MA: MIT Press
- Imbens GW, Lemieux T. 2008. Regression discontinuity designs: a guide to practice. J. Econom. 142:615–35