AUC Score :
Short-Term Revised1 :
Dominant Strategy :
Time series to forecast n:
ML Model Testing : Modular Neural Network (Financial Sentiment Analysis)
Hypothesis Testing : Statistical Hypothesis Testing
Surveillance : Major exchange and OTC
1The accuracy of the model is being monitored on a regular basis.(15-minute period)
2Time series is updated based on short-term trends.
Key Points
Transdigm's future performance hinges on several key factors. Continued strong demand for its aerospace and industrial products, particularly in the commercial aviation and defense sectors, is crucial. Sustained profitability within these markets, however, faces potential risks associated with fluctuating global economic conditions, supply chain disruptions, and competition. Successful execution of their strategic initiatives and cost-reduction measures will be important for driving growth. Failure to adapt to changing market dynamics and potential regulatory hurdles could negatively impact earnings and future revenue projections. Investment in new technologies and expansion into emerging markets could drive future growth and enhance shareholder value, but these endeavors often involve considerable risk. Ultimately, Transdigm's stock performance will be closely tied to the overall health of the global economy and the company's ability to execute its strategic plans while mitigating these potential risks.About Transdigm Group
Transdigm (TDG) is a global aerospace and defense company specializing in the design, development, and manufacturing of advanced technologies. The company is a leading provider of engineered solutions for aircraft, including components for engines, landing gear, flight controls, and cabin interiors. Transdigm's products and services also extend to other sectors, such as commercial vehicles and industrial markets. The company emphasizes high-quality engineering and innovative design in its pursuit of product excellence and customer satisfaction.
Transdigm's business model is primarily focused on supplying components and systems to major aerospace manufacturers. It operates through various divisions, each contributing unique technological capabilities. The company's strategy revolves around continuous improvement, innovation, and expanding market share. Significant investments in research and development are a key aspect of maintaining competitiveness and delivering cutting-edge solutions to the industry.
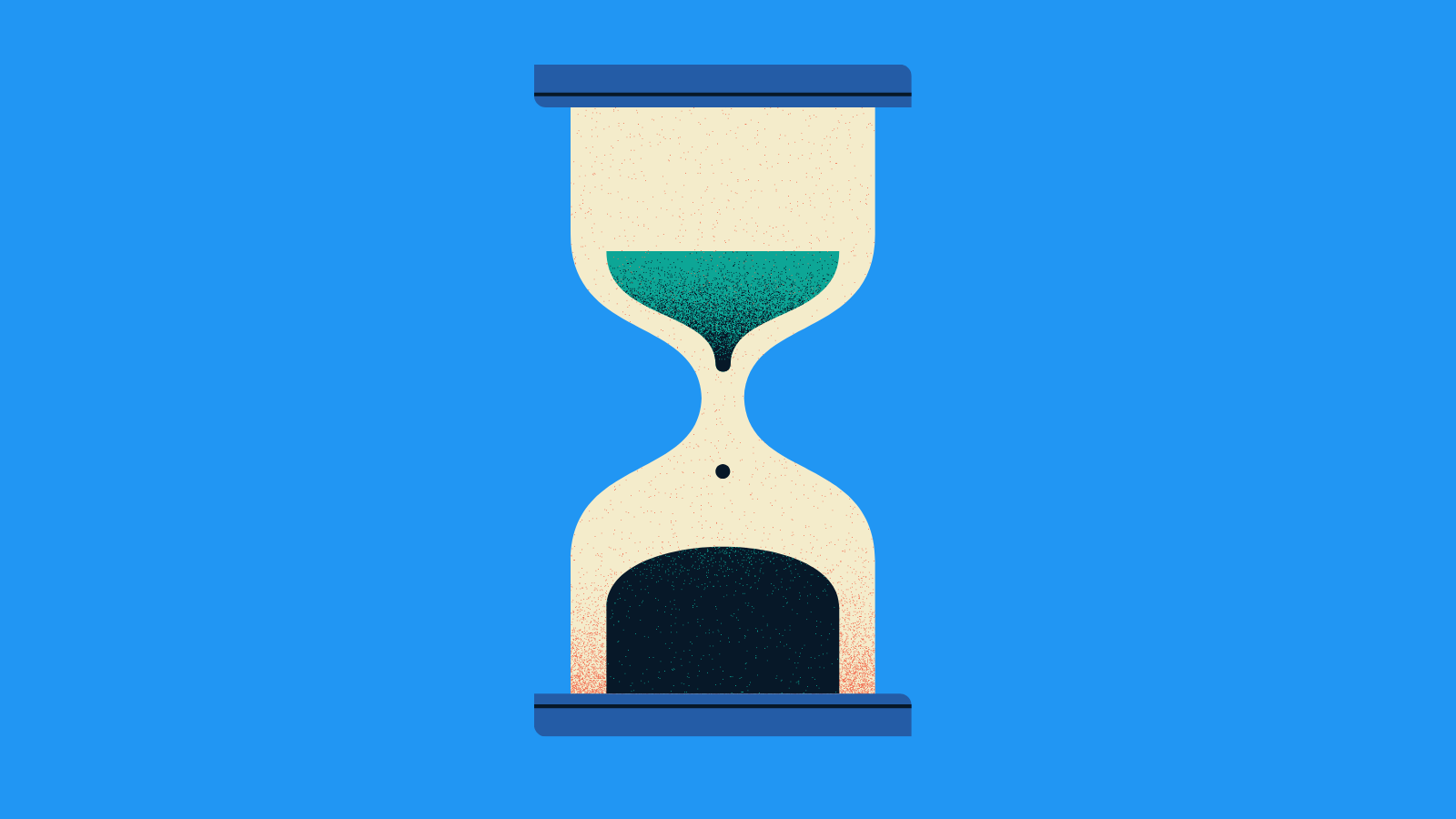
Transdigm Group Incorporated Common Stock (TDG) Stock Forecast Model
To develop a machine learning model for forecasting Transdigm Group Incorporated (TDG) stock, a comprehensive dataset encompassing various financial and macroeconomic indicators is essential. This dataset would include historical stock prices, but also key financial ratios like earnings per share (EPS), revenue growth, debt-to-equity ratios, and cash flow. Furthermore, incorporating macroeconomic variables such as GDP growth, inflation rates, interest rates, and market sentiment indices can significantly enhance predictive accuracy. These factors are crucial to understanding market trends that can influence TDG's performance. We'd employ feature engineering techniques to transform raw data into more informative features, such as indicators of future earnings potential, potentially through the use of moving averages, ratios, and momentum indicators. The selection of appropriate machine learning algorithms, considering the complexity of the stock market, will be carefully evaluated. This could include Recurrent Neural Networks (RNNs), Long Short-Term Memory (LSTMs) networks, or potentially gradient boosting algorithms like XGBoost. The choice would depend on the specific characteristics of the dataset and the desired forecasting horizon. Model training will be rigorously tested with a holdout set to evaluate its generalizability and accuracy on unseen data.
Data preprocessing is paramount to ensure model robustness. This involves handling missing values, outliers, and transforming data to appropriate scales. Normalization and standardization techniques will be employed to prevent features with larger values from dominating the model training process. Properly handling these elements is crucial for preventing model bias and improving predictive accuracy. Critical evaluation of potential biases within the data, such as survivorship bias, and careful consideration of the model's limitations are necessary. Model validation will involve a rigorous process of back-testing and cross-validation to ensure that the model's forecasts align with historical patterns and demonstrate a strong predictive capacity. Regular monitoring of the model's performance will allow for adaptation and adjustments to the model's architecture or input features as needed. Interpretability of the model is equally important for a deeper understanding of the driving factors behind predicted stock movements. This allows for strategic insights for investors and the identification of potential early warning signals from the market.
The model's output will be presented as a probability distribution of possible future stock price movements, reflecting the inherent uncertainty in market predictions. Visualizations and clear reporting of model performance metrics, such as Mean Squared Error (MSE) or Root Mean Squared Error (RMSE) will be provided for transparency. A clear communication strategy, outlining the model's limitations and potential risks will be crucial to ensure appropriate stakeholder interpretation and utilization of the results. Ongoing monitoring and refinement of the model will be essential given the dynamic nature of financial markets. Regular updating of the input data and re-evaluation of model performance will ensure continued accuracy and relevance over time. This iterative process ensures the model's continued effectiveness for predicting future stock trends of Transdigm Group Incorporated.
ML Model Testing
n:Time series to forecast
p:Price signals of Transdigm Group stock
j:Nash equilibria (Neural Network)
k:Dominated move of Transdigm Group stock holders
a:Best response for Transdigm Group target price
For further technical information as per how our model work we invite you to visit the article below:
How do KappaSignal algorithms actually work?
Transdigm Group Stock Forecast (Buy or Sell) Strategic Interaction Table
Strategic Interaction Table Legend:
X axis: *Likelihood% (The higher the percentage value, the more likely the event will occur.)
Y axis: *Potential Impact% (The higher the percentage value, the more likely the price will deviate.)
Z axis (Grey to Black): *Technical Analysis%
Transdigm Financial Outlook and Forecast
Transdigm (TDG) operates in the aerospace and defense sectors, providing critical components and services for aircraft and other systems. The company's financial outlook is intertwined with the performance of the broader aviation industry, specifically commercial air travel. A robust recovery in air travel, driven by economic growth and pent-up demand, would significantly benefit TDG's revenue and earnings. Key performance indicators, such as order intake for their aftermarket services and the demand for their specialized components, are crucial to gauge the company's financial health. Historical data, including trends in global air traffic, will be crucial to forecasting future performance. Several sectors within Transdigm contribute to its overall financial success, including its aerospace component segment, its aftermarket services, and its other key divisions, each with different performance drivers and sensitivities. Sustained innovation and production capacity will play a vital role in maintaining the company's competitiveness in a rapidly evolving industry. The company's exposure to potential geopolitical risks, such as trade disputes or political instability, can create significant uncertainty in its financial outlook. It is expected to be an important factor in future predictions.
A positive outlook for TDG hinges on the continued recovery and expansion of global air travel. Factors like increased air traffic, rising passenger numbers, and the demand for aircraft maintenance, repair, and overhaul (MRO) services are expected to drive revenue growth. The current trends in the aviation industry need to be considered, as well as the potential impact of ongoing or anticipated economic fluctuations. Increased spending on new aircraft and the need for associated components will also influence TDG's revenue. A slowdown in air travel or delays in the recovery could negatively impact the company's revenue and profitability, putting downward pressure on financial results. This is closely tied to overall global economic trends and any potential recessions or economic downturns. External factors, including geopolitical events, supply chain disruptions, and technological advancements in the aerospace industry, will all exert influence on the company's profitability and market position. Analyzing macroeconomic trends is critical to understanding the possible impact on Transdigm's performance.
An important component of Transdigm's future financial performance will be its ability to effectively manage costs and maintain its operational efficiency. The company's product offerings and services will have to continue to meet and exceed the industry's standards for reliability and quality. Efficient supply chain management will be critical to ensuring cost-effectiveness and avoiding potential disruptions. Staying agile and innovative will allow TDG to adapt to shifting market demands and remain competitive. Maintaining strong relationships with key customers and strategic partnerships will be vital for continued success. The overall financial outlook will depend greatly on the company's capability to effectively manage its supply chains, its customer relations, and its pricing policies. The company's approach to these strategic elements will significantly influence its future financial trajectory. Factors like changes in regulatory requirements and environmental sustainability initiatives will also influence the company's strategic decision-making.
Predictive outlook: A moderate, positive outlook is foreseen, given the current trends in aviation demand. However, several risks threaten this prediction. Geopolitical instability and global economic uncertainty may negatively impact air travel and reduce the demand for TDG's products and services, causing a downward revision in earnings projections. Supply chain disruptions, increased raw material costs, and the emergence of new, disruptive technologies in the aerospace industry also pose potential risks to profitability. The accuracy of forecasts is dependent on the continued recovery of air travel, and the ability to navigate through the mentioned risks. In the event that the anticipated demand for TDG's products does not materialize, or the company faces unexpected production challenges, profitability could be significantly affected. The company's management must effectively address these risks to ensure the predicted positive outlook is achieved.
Rating | Short-Term | Long-Term Senior |
---|---|---|
Outlook | B2 | B1 |
Income Statement | B2 | Baa2 |
Balance Sheet | C | Baa2 |
Leverage Ratios | Ba2 | C |
Cash Flow | B3 | B3 |
Rates of Return and Profitability | Caa2 | B2 |
*Financial analysis is the process of evaluating a company's financial performance and position by neural network. It involves reviewing the company's financial statements, including the balance sheet, income statement, and cash flow statement, as well as other financial reports and documents.
How does neural network examine financial reports and understand financial state of the company?
References
- Gentzkow M, Kelly BT, Taddy M. 2017. Text as data. NBER Work. Pap. 23276
- J. G. Schneider, W. Wong, A. W. Moore, and M. A. Riedmiller. Distributed value functions. In Proceedings of the Sixteenth International Conference on Machine Learning (ICML 1999), Bled, Slovenia, June 27 - 30, 1999, pages 371–378, 1999.
- F. A. Oliehoek, M. T. J. Spaan, and N. A. Vlassis. Optimal and approximate q-value functions for decentralized pomdps. J. Artif. Intell. Res. (JAIR), 32:289–353, 2008
- Matzkin RL. 1994. Restrictions of economic theory in nonparametric methods. In Handbook of Econometrics, Vol. 4, ed. R Engle, D McFadden, pp. 2523–58. Amsterdam: Elsevier
- Arora S, Li Y, Liang Y, Ma T. 2016. RAND-WALK: a latent variable model approach to word embeddings. Trans. Assoc. Comput. Linguist. 4:385–99
- Blei DM, Lafferty JD. 2009. Topic models. In Text Mining: Classification, Clustering, and Applications, ed. A Srivastava, M Sahami, pp. 101–24. Boca Raton, FL: CRC Press
- Mnih A, Hinton GE. 2007. Three new graphical models for statistical language modelling. In International Conference on Machine Learning, pp. 641–48. La Jolla, CA: Int. Mach. Learn. Soc.