AUC Score :
Short-Term Revised1 :
Dominant Strategy :
Time series to forecast n:
ML Model Testing : Supervised Machine Learning (ML)
Hypothesis Testing : Paired T-Test
Surveillance : Major exchange and OTC
1The accuracy of the model is being monitored on a regular basis.(15-minute period)
2Time series is updated based on short-term trends.
Key Points
The S&P Ethereum index is anticipated to experience significant volatility in the coming period. Potential upward momentum is likely contingent upon sustained positive macroeconomic indicators and a resolution of ongoing regulatory uncertainties surrounding the cryptocurrency market. However, downturns could be triggered by unfavorable economic developments, regulatory crackdowns, or negative market sentiment. Further, the index's performance is highly susceptible to shifts in investor confidence and speculative trading activity within the digital asset market. This volatility presents substantial risks for investors. Major risks include substantial losses if market sentiment shifts negatively, and heightened risk of mispricing due to speculative pressures within the decentralized finance sector.About S&P Ethereum Index
The S&P Ethereum Index is a benchmark that tracks the performance of publicly traded companies significantly involved in the Ethereum ecosystem. It aims to capture the overall market capitalization of this segment. While not directly tracking Ethereum's price per coin, the index reflects the collective market value of businesses that operate within and around the Ethereum blockchain, including those in decentralized finance (DeFi), non-fungible tokens (NFTs), and other related industries. Its composition and methodology are designed to provide an objective measure of this specific market segment's overall performance, offering investors a means to assess and potentially invest in this evolving area of the cryptocurrency market.
The index is typically comprised of a basket of publicly listed companies, reflecting the current market landscape. Its design is crucial to offer a more nuanced understanding of the Ethereum-adjacent market beyond the price of the native Ethereum cryptocurrency. This allows for a more comprehensive evaluation, going beyond just cryptocurrencies themselves and acknowledging the broad range of companies involved in this blockchain technology's applications. However, it's vital to acknowledge that the index reflects only a selected portion of the broader market and does not represent the full breadth of the Ethereum ecosystem.
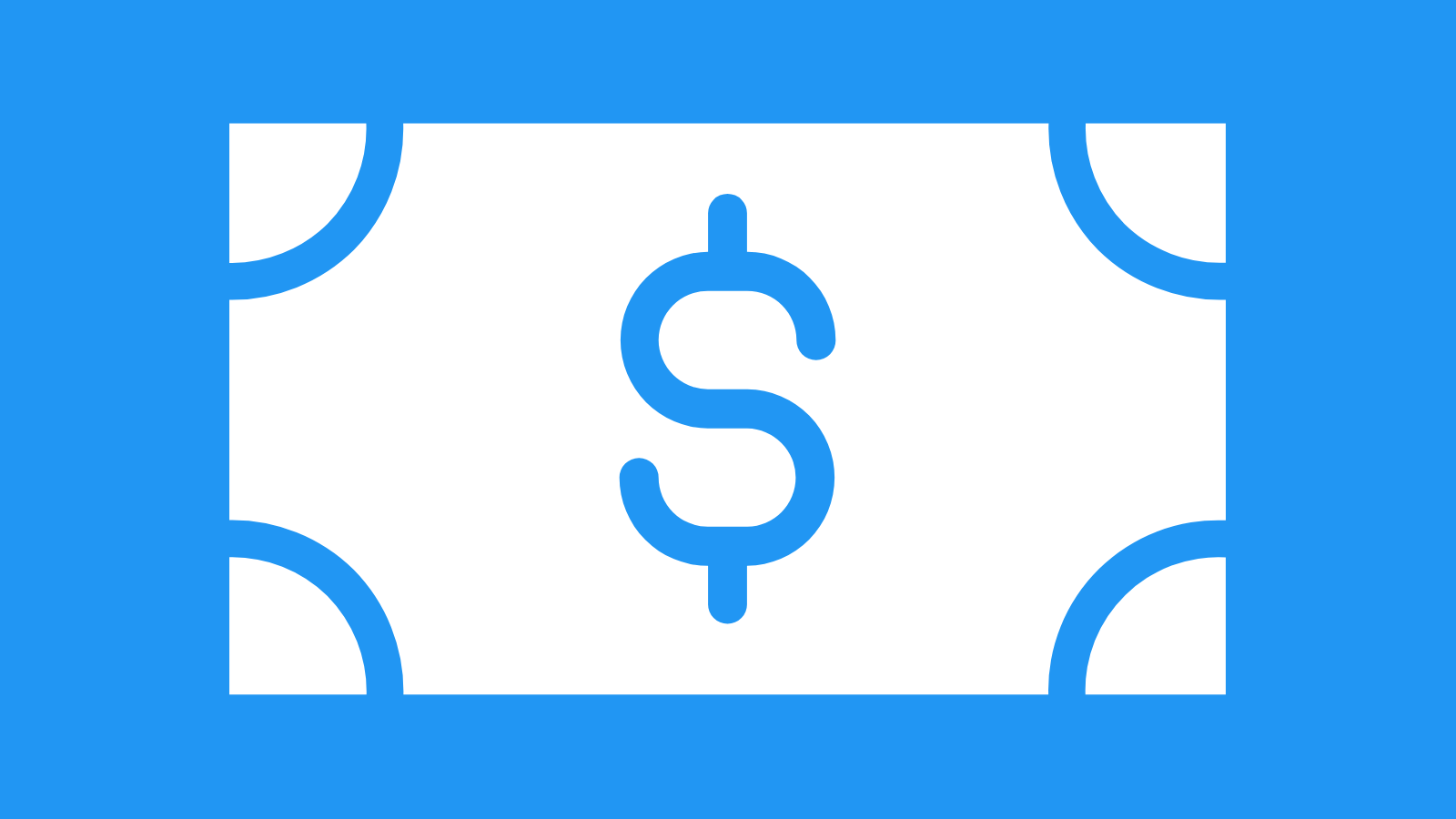
S&P Ethereum Index Future Price Forecasting Model
To predict the future trajectory of the S&P Ethereum index, we propose a machine learning model leveraging a combination of historical market data and macroeconomic indicators. The model architecture will comprise several key components. Firstly, a robust dataset is crucial. This dataset will encompass historical price fluctuations of the S&P Ethereum index, alongside relevant macroeconomic indicators such as inflation rates, interest rates, and geopolitical events. These indicators will be meticulously preprocessed to account for seasonality, trends, and outliers. Feature engineering will be paramount, aiming to capture complex relationships between variables. This will involve generating new features from existing ones, such as moving averages, volatility measures, and correlations. Crucially, the model must incorporate data specific to the cryptocurrency market, including trading volume and market sentiment.
The model itself will employ a combination of supervised learning algorithms. Regression models, such as support vector regression (SVR) or gradient boosting, are well-suited for forecasting continuous values like the S&P Ethereum index. These algorithms will be trained on the preprocessed dataset, learning the complex relationships between input features and the index's future values. To enhance predictive accuracy, we will employ techniques like cross-validation to assess model performance on unseen data and avoid overfitting. Hyperparameter tuning will be implemented to optimize the chosen algorithm for the specific dataset. Furthermore, a careful consideration of potential model biases and their impact on forecasting accuracy is crucial.
Evaluation of the model's performance will involve rigorous testing. We will utilize metrics like root mean squared error (RMSE) and mean absolute error (MAE) to assess the accuracy of the model's predictions. Furthermore, we will conduct backtesting using historical data to evaluate the model's predictive power over time. Regular retraining and updating of the model are necessary to adapt to evolving market conditions and new data. Model deployment will involve establishing a robust infrastructure to ensure real-time predictions, providing timely insights for decision-making in the investment domain. The model should be carefully monitored and refined based on feedback and real-world performance to ensure ongoing efficacy.
ML Model Testing
n:Time series to forecast
p:Price signals of S&P Ethereum index
j:Nash equilibria (Neural Network)
k:Dominated move of S&P Ethereum index holders
a:Best response for S&P Ethereum target price
For further technical information as per how our model work we invite you to visit the article below:
How do KappaSignal algorithms actually work?
S&P Ethereum Index Forecast Strategic Interaction Table
Strategic Interaction Table Legend:
X axis: *Likelihood% (The higher the percentage value, the more likely the event will occur.)
Y axis: *Potential Impact% (The higher the percentage value, the more likely the price will deviate.)
Z axis (Grey to Black): *Technical Analysis%
S&P Ethereum Index Financial Outlook and Forecast
The financial outlook for the S&P Ethereum index hinges on several interconnected factors, primarily the trajectory of the underlying Ethereum cryptocurrency market. Ethereum's position as a leading blockchain platform, and its evolving ecosystem of decentralized applications (dApps), plays a critical role. The index's performance is intricately linked to the value proposition of Ethereum as a technological platform, and its ability to attract developers and users. This includes the ongoing development and deployment of crucial upgrades within the Ethereum network, as well as regulatory developments related to cryptocurrencies and blockchain technologies. Factors influencing developer activity, market adoption of Ethereum-based projects, and the overall health of the cryptocurrency market all directly impact the S&P Ethereum index's valuation.
The long-term outlook for the S&P Ethereum index incorporates a nuanced perspective on technological progress within the blockchain domain. Innovation within the Ethereum ecosystem, such as the introduction of new smart contracts or the development of more sophisticated decentralized finance (DeFi) protocols, could potentially drive substantial growth in the index's value. Equally significant is the evolving regulatory environment impacting cryptocurrencies. Governmental policies and regulatory bodies' approaches to blockchain and cryptocurrencies will directly influence market confidence and investment opportunities. This uncertainty adds a degree of volatility to the index's performance and requires a thorough examination of both technological developments and regulatory pressures.
Forecasting the precise trajectory of the S&P Ethereum index presents considerable challenges due to the inherent volatility of the cryptocurrency market. Market sentiment, speculative trading, and unexpected events, such as security breaches or regulatory crackdowns, can significantly affect the index's value. The future of the index also relies heavily on broader market trends. Economic conditions, geopolitical events, and investor confidence all have an impact on the overall performance of the financial markets. The ability of the Ethereum network to adapt to future demands, overcome technological hurdles, and garner continued widespread adoption will also significantly shape the S&P Ethereum index's prospects.
Predicting a positive or negative outlook for the S&P Ethereum index involves acknowledging the significant uncertainties embedded in the crypto market. While the potential for substantial growth within the Ethereum ecosystem remains strong, driven by innovative applications and technological advancements, the inherent risks of the crypto market are significant and should not be discounted. Factors such as regulatory uncertainty, market corrections, and security vulnerabilities could all negatively impact the index. Furthermore, the index's performance is vulnerable to broader economic downturns and changes in investor sentiment. The presence of a multitude of competing blockchain projects and developments in alternative technologies could also serve as a mitigating factor to growth. Therefore, any positive prediction must be tempered by the inherent risks. A thorough understanding of both the opportunities and the potential pitfalls is critical for investors considering this index.
Rating | Short-Term | Long-Term Senior |
---|---|---|
Outlook | Ba2 | B2 |
Income Statement | Ba3 | C |
Balance Sheet | B2 | Baa2 |
Leverage Ratios | Baa2 | Caa2 |
Cash Flow | B2 | B1 |
Rates of Return and Profitability | Baa2 | Caa2 |
*An aggregate rating for an index summarizes the overall sentiment towards the companies it includes. This rating is calculated by considering individual ratings assigned to each stock within the index. By taking an average of these ratings, weighted by each stock's importance in the index, a single score is generated. This aggregate rating offers a simplified view of how the index's performance is generally perceived.
How does neural network examine financial reports and understand financial state of the company?
References
- Athey S, Wager S. 2017. Efficient policy learning. arXiv:1702.02896 [math.ST]
- Ashley, R. (1988), "On the relative worth of recent macroeconomic forecasts," International Journal of Forecasting, 4, 363–376.
- Imbens GW, Rubin DB. 2015. Causal Inference in Statistics, Social, and Biomedical Sciences. Cambridge, UK: Cambridge Univ. Press
- K. Tuyls and G. Weiss. Multiagent learning: Basics, challenges, and prospects. AI Magazine, 33(3): 41–52, 2012
- V. Mnih, K. Kavukcuoglu, D. Silver, A. Rusu, J. Veness, M. Bellemare, A. Graves, M. Riedmiller, A. Fidjeland, G. Ostrovski, S. Petersen, C. Beattie, A. Sadik, I. Antonoglou, H. King, D. Kumaran, D. Wierstra, S. Legg, and D. Hassabis. Human-level control through deep reinforcement learning. Nature, 518(7540):529–533, 02 2015.
- Abadie A, Imbens GW. 2011. Bias-corrected matching estimators for average treatment effects. J. Bus. Econ. Stat. 29:1–11
- Chernozhukov V, Chetverikov D, Demirer M, Duflo E, Hansen C, et al. 2016a. Double machine learning for treatment and causal parameters. Tech. Rep., Cent. Microdata Methods Pract., Inst. Fiscal Stud., London