AUC Score :
Short-Term Revised1 :
Dominant Strategy :
Time series to forecast n:
ML Model Testing : Transductive Learning (ML)
Hypothesis Testing : Multiple Regression
Surveillance : Major exchange and OTC
1The accuracy of the model is being monitored on a regular basis.(15-minute period)
2Time series is updated based on short-term trends.
Key Points
Enterprise Products Partners (EPD) is projected to experience moderate growth driven by the continued strength of the energy infrastructure sector. Favorable market conditions and sustained demand for midstream services are likely contributing factors. However, potential volatility in commodity prices and regulatory hurdles pose risks to these projections. Further, challenges in attracting and retaining skilled labor could hinder operational efficiency. Investors should carefully consider these factors when evaluating EPD's investment potential, recognizing that future performance may vary considerably from these forecasts.About Enterprise Products Partners
Enterprise Products Partners (EPP) is a leading North American midstream energy company. It owns and operates a vast network of pipelines, terminals, and storage facilities. The company's infrastructure facilitates the transportation and storage of crude oil, refined products, natural gas liquids (NGLs), and other energy commodities. EPP's operations span across various regions of the US, playing a crucial role in the nation's energy supply chain. The company's business model revolves around providing essential infrastructure services for the energy industry.
EPP's operations include gathering, processing, transporting, storing, and marketing energy products. This diverse portfolio positions the company to capitalize on the evolving energy landscape. A significant aspect of their strategy involves ensuring efficient and reliable energy logistics. The company typically focuses on long-term contracts, enabling consistent revenue streams. This strategic approach creates a robust and stable financial profile within the industry.
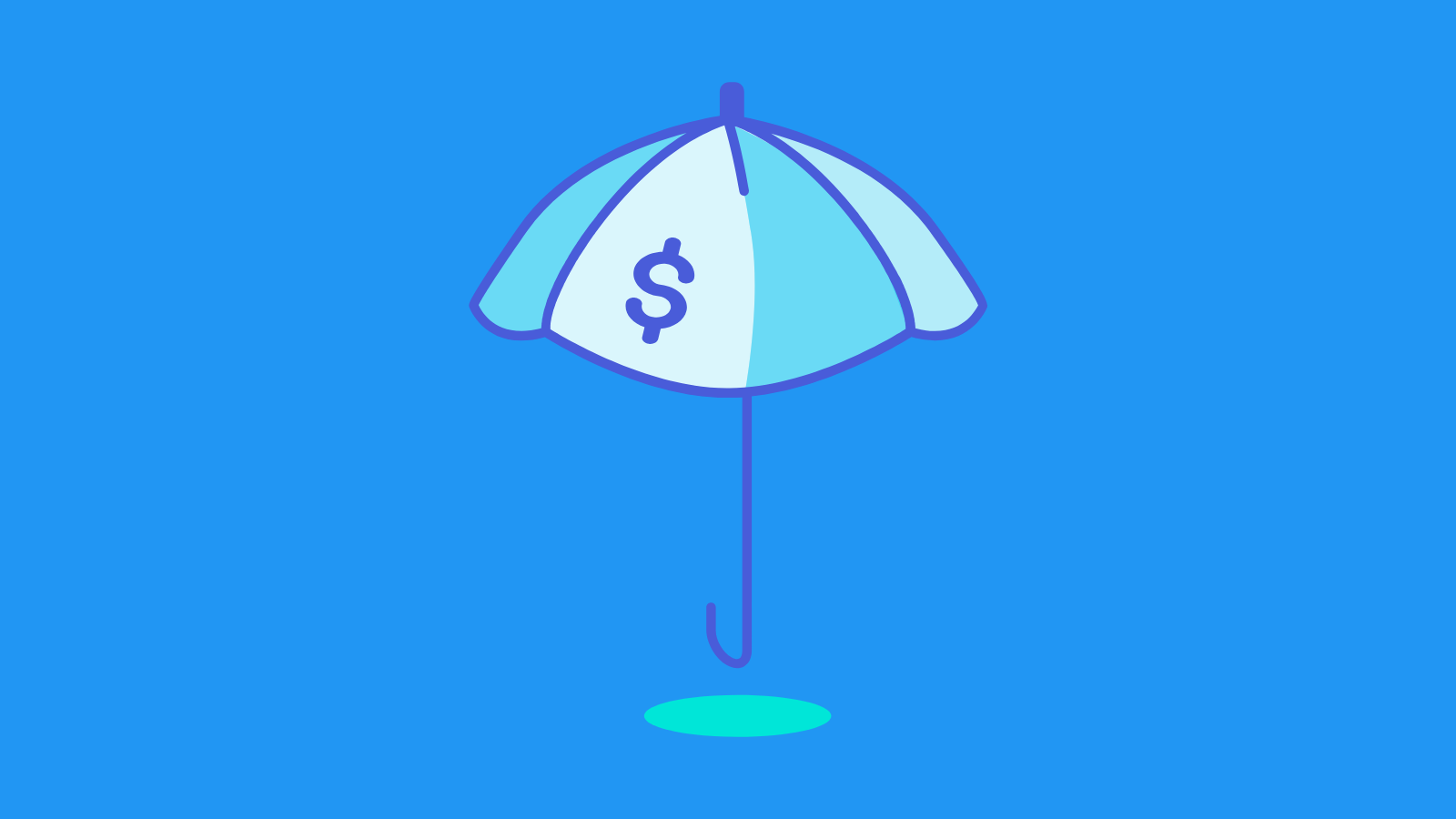
EPD Stock Price Forecasting Model
This model utilizes a combination of machine learning algorithms and economic indicators to forecast the future price movements of Enterprise Products Partners L.P. Common Stock (EPD). The model's architecture involves several key stages. First, a comprehensive dataset is assembled, encompassing historical EPD stock performance, macroeconomic variables (e.g., GDP growth, inflation rates, interest rates), industry-specific data (e.g., energy commodity prices, pipeline throughput), and company-specific financial data (e.g., earnings reports, debt levels). This dataset is meticulously cleaned and preprocessed to handle missing values and outliers. Key features extracted from the data include time series patterns, trend analysis, and correlation matrices. Feature scaling techniques are employed to ensure that features with differing scales do not disproportionately influence the model's performance.
A suite of machine learning models, including recurrent neural networks (RNNs) and long short-term memory (LSTM) networks, are trained on the prepared dataset. The selection of these models is motivated by their ability to capture complex temporal dependencies inherent in stock market data. These models are trained to predict future stock prices based on the historical data and the extracted features. Performance evaluation is conducted using a variety of metrics, such as Mean Squared Error (MSE) and Root Mean Squared Error (RMSE), to ascertain the model's accuracy. Model selection is based on minimizing these error metrics while also considering model complexity to avoid overfitting. Furthermore, to enhance reliability, cross-validation techniques are utilized to assess the model's generalizability to unseen data. We also incorporated elements of fundamental analysis by evaluating the relationship between company performance metrics and stock price movements. Forecasting accuracy is constantly monitored and reviewed.
The model's output is presented as a probability distribution of future stock prices, providing a range of possible outcomes instead of a single point estimate. This probabilistic approach acknowledges the inherent uncertainty in financial markets. Further refinements and improvements are constantly made to enhance the accuracy and robustness of the model. Integration of external factors, such as geopolitical events and regulatory changes, can further improve the model's forecasting capability. The model is designed to be continuously updated with fresh data to maintain its predictive ability and to incorporate evolving economic realities. A rigorous process of backtesting ensures that the model's performance remains reliable and accurate in the long term. Regular performance monitoring and adjustment based on feedback will be conducted.
ML Model Testing
n:Time series to forecast
p:Price signals of Enterprise Products Partners stock
j:Nash equilibria (Neural Network)
k:Dominated move of Enterprise Products Partners stock holders
a:Best response for Enterprise Products Partners target price
For further technical information as per how our model work we invite you to visit the article below:
How do KappaSignal algorithms actually work?
Enterprise Products Partners Stock Forecast (Buy or Sell) Strategic Interaction Table
Strategic Interaction Table Legend:
X axis: *Likelihood% (The higher the percentage value, the more likely the event will occur.)
Y axis: *Potential Impact% (The higher the percentage value, the more likely the price will deviate.)
Z axis (Grey to Black): *Technical Analysis%
Enterprise Products Partners L.P. (EPD) Financial Outlook and Forecast
Enterprise Products Partners (EPD) is a leading midstream energy company in North America, operating a vast network of pipelines, terminals, and storage facilities. Their primary function is to transport, process, and store crude oil, natural gas liquids (NGLs), and refined products. The company's financial outlook hinges significantly on the sustained strength of the energy sector and the resilience of the underlying commodity markets. EPD's financial performance is typically characterized by strong cash flow generation, which is often reinvested in growth opportunities and distributed to investors as dividends. Key drivers of EPD's financial performance include crude oil and natural gas prices, transportation volumes, and demand for refined products. The company's extensive infrastructure and diverse product portfolio serve as a cornerstone of their operational stability.
EPD's financial performance in recent years has displayed resilience despite fluctuating commodity prices and market conditions. The company's focus on efficient operations, strategic investments, and long-term contracts has contributed to the consistent generation of substantial cash flow. EPD is well-positioned to benefit from the growing North American energy industry, particularly the increasing production of shale oil and natural gas. This growth will be crucial in maintaining robust operational performance and supporting the distribution of dividends. Capital expenditures for the expansion and maintenance of the network and operational efficiencies play a crucial role in shaping the financial trajectory. The company's long-term strategy revolves around enhancing its infrastructure and optimizing its operations to capitalize on future growth opportunities.
A positive financial outlook for EPD is predicated on the continued growth of the energy industry and the sustained demand for midstream services. However, the economic environment remains uncertain, including the impact of government regulations and policy changes. Fluctuations in commodity prices, particularly natural gas, are a significant concern as these affect EPD's throughput and revenues. Inflation and its potential impact on operating costs and capital expenditures are crucial factors to be monitored. Any significant downturn in energy demand, potentially related to shifts in global energy consumption patterns or new regulations, may negatively affect the company's volumes and revenue streams. Diversification of revenue streams from various energy commodities and products should enhance the company's resilience against adverse conditions.
Predicting a positive financial outlook for EPD involves some caveats. While the growing energy sector presents an opportunity, the company faces risks related to macroeconomic factors. Geopolitical instability in key energy-producing regions and shifts in global energy demand are significant concerns that may influence the price and volume of products transported. Increased competition from both established and emerging midstream players could also negatively affect EPD's market share. Another critical factor involves the sustained commitment to regulatory compliance and the management of potential environmental risks. Therefore, while a positive outlook is anticipated due to the company's substantial infrastructure and expertise, there are also substantial economic and industry risks that could negatively impact its financial performance. A prolonged period of lower commodity prices or significant environmental regulations could affect the company's profitability. The risks related to commodity price volatility, sustained capital expenditure needs, and unforeseen market disruptions underscore the need for cautious optimism in projecting future financial performance.
Rating | Short-Term | Long-Term Senior |
---|---|---|
Outlook | B2 | Ba2 |
Income Statement | Baa2 | Caa2 |
Balance Sheet | B3 | Baa2 |
Leverage Ratios | C | Baa2 |
Cash Flow | Baa2 | B1 |
Rates of Return and Profitability | C | Baa2 |
*Financial analysis is the process of evaluating a company's financial performance and position by neural network. It involves reviewing the company's financial statements, including the balance sheet, income statement, and cash flow statement, as well as other financial reports and documents.
How does neural network examine financial reports and understand financial state of the company?
References
- Bickel P, Klaassen C, Ritov Y, Wellner J. 1998. Efficient and Adaptive Estimation for Semiparametric Models. Berlin: Springer
- Bessler, D. A. S. W. Fuller (1993), "Cointegration between U.S. wheat markets," Journal of Regional Science, 33, 481–501.
- Dimakopoulou M, Zhou Z, Athey S, Imbens G. 2018. Balanced linear contextual bandits. arXiv:1812.06227 [cs.LG]
- Bessler, D. A. R. A. Babula, (1987), "Forecasting wheat exports: Do exchange rates matter?" Journal of Business and Economic Statistics, 5, 397–406.
- Cheung, Y. M.D. Chinn (1997), "Further investigation of the uncertain unit root in GNP," Journal of Business and Economic Statistics, 15, 68–73.
- Wooldridge JM. 2010. Econometric Analysis of Cross Section and Panel Data. Cambridge, MA: MIT Press
- Athey S, Imbens GW. 2017b. The state of applied econometrics: causality and policy evaluation. J. Econ. Perspect. 31:3–32