AUC Score :
Short-Term Revised1 :
Dominant Strategy :
Time series to forecast n:
ML Model Testing : Modular Neural Network (DNN Layer)
Hypothesis Testing : Paired T-Test
Surveillance : Major exchange and OTC
1The accuracy of the model is being monitored on a regular basis.(15-minute period)
2Time series is updated based on short-term trends.
Key Points
The Dow Jones U.S. Select Investment Services index is anticipated to experience moderate growth, driven by ongoing positive trends in the investment sector. This projection, however, carries inherent risks. Economic instability, such as fluctuating interest rates or unexpected market corrections, could significantly impact the index's performance. Furthermore, regulatory changes affecting investment services firms could create uncertainty and potentially lower returns. Competition within the investment industry also poses a risk, as new entrants or evolving strategies could alter market share dynamics. Finally, global events, including geopolitical tensions and natural disasters, can influence investor sentiment and affect the index's trajectory.About Dow Jones U.S. Select Investment Services Index
The Dow Jones U.S. Select Investment Services index is a market capitalization-weighted index that tracks the performance of companies in the investment services sector. It provides a benchmark for investors seeking exposure to this specific segment of the U.S. equity market. The index selection process is designed to capture the diverse range of businesses within the investment services industry, encompassing brokerage firms, financial advisors, and investment management companies. The index aims to reflect the overall health and performance of this sector within the broader economy. Changes to index constituents and methodologies, along with the index's performance, are usually detailed in announcements by the index provider.
The index, like other market indexes, is designed to offer a consistent and comparable measure of sector performance. It allows for analysis of the sector's trends and facilitates comparative assessments of individual company performance within the investment services industry. The performance of the index can be a useful tool for investors to assess exposure to specific sectors of the investment world. Furthermore, the index and its performance are often used in conjunction with other metrics to paint a more complete picture of financial market behavior.
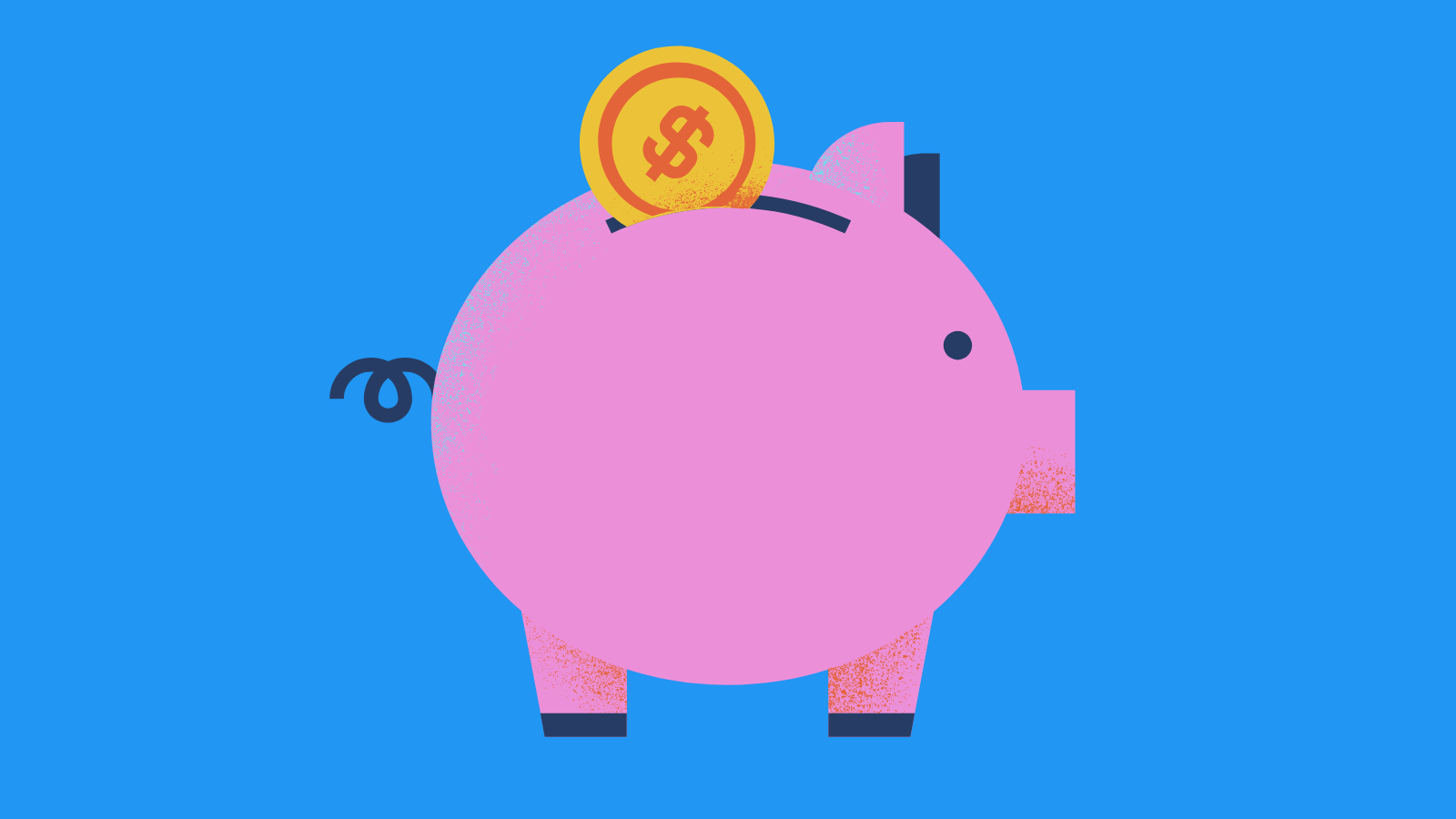
Dow Jones U.S. Select Investment Services Index Forecast Model
To forecast the Dow Jones U.S. Select Investment Services index, we leverage a time series model incorporating macroeconomic indicators and company-specific data. The model architecture comprises a sequential LSTM (Long Short-Term Memory) network, augmented by a feature engineering process that extracts relevant insights from financial news sentiment, interest rate fluctuations, and industry-specific trends. The LSTM architecture is adept at capturing temporal dependencies within the financial market, crucial for anticipating index movements. We meticulously curate a dataset spanning several years, including historical index values, key economic indicators (GDP growth, inflation rates, unemployment), and pertinent company financial statements. Feature selection and scaling are paramount to ensure that the model effectively processes the diverse range of input variables. Careful consideration is given to the potential impact of market volatility and the inherent limitations of predictive models in the financial arena. The model is trained and validated on a robust dataset, employing techniques like cross-validation to mitigate overfitting and optimize its generalization capacity. Further enhancements are considered through regularized methods to maintain model stability and interpretability.
To assess the model's predictive capabilities, a comprehensive evaluation strategy is deployed, encompassing metrics like mean squared error (MSE), root mean squared error (RMSE), and mean absolute error (MAE). These metrics quantify the model's accuracy in approximating future index values. A critical step involves backtesting the model on historical data to evaluate its performance under various market conditions. The accuracy of the forecasts is monitored continuously and recalibrated to adapt to changing market trends and evolving economic conditions. Regular retraining of the model is necessary to ensure its continued relevance in light of new information. A risk assessment framework is integrated, considering potential outliers and extreme market events, to provide a more nuanced outlook on forecast reliability. Robust error handling and mitigation strategies are integrated into the model pipeline to account for data anomalies and minimize the impact of unexpected events.
The model's output is presented as a probability distribution of potential future index values, acknowledging the inherent uncertainty in financial forecasting. This distribution-based approach offers a more comprehensive understanding of the potential range of outcomes, which is particularly valuable for investors and financial analysts. The model is continuously monitored for performance degradation and adaptability, and its underlying assumptions and limitations are documented in a transparent manner. Ongoing data collection and validation are pivotal to maintain the model's accuracy and relevance. Ongoing updates to the model architecture and training procedures will be made based on the evaluation of its performance. The incorporation of real-time data feeds is planned for future iterations of the model to improve forecasting responsiveness to immediate market shifts. Model transparency and interpretability are essential features that are explicitly considered in the design of the model, enabling meaningful insights into the factors driving the forecasts and facilitating informed decision-making.
ML Model Testing
n:Time series to forecast
p:Price signals of Dow Jones U.S. Select Investment Services index
j:Nash equilibria (Neural Network)
k:Dominated move of Dow Jones U.S. Select Investment Services index holders
a:Best response for Dow Jones U.S. Select Investment Services target price
For further technical information as per how our model work we invite you to visit the article below:
How do KappaSignal algorithms actually work?
Dow Jones U.S. Select Investment Services Index Forecast Strategic Interaction Table
Strategic Interaction Table Legend:
X axis: *Likelihood% (The higher the percentage value, the more likely the event will occur.)
Y axis: *Potential Impact% (The higher the percentage value, the more likely the price will deviate.)
Z axis (Grey to Black): *Technical Analysis%
Dow Jones U.S. Select Investment Services Index Financial Outlook and Forecast
The Dow Jones U.S. Select Investment Services Index, a benchmark for the performance of companies within the investment services sector, is anticipated to experience a period of moderate growth in the coming fiscal year. Several factors suggest this trajectory. Strong consumer confidence and increased investor activity, particularly in the stock market, are expected to drive demand for financial advisory services. This increased demand should translate into higher revenue for investment banks and brokerage firms, which are major constituents of the index. Furthermore, improved regulatory environments, while sometimes complex, can create a more stable and favorable operating landscape, potentially leading to positive performance. The index may also benefit from ongoing technological advancements in the financial sector, creating new opportunities for growth and potentially attracting investors.
However, the outlook is not without its challenges. Economic uncertainty, including fluctuating interest rates, potential inflation, and global geopolitical events, could negatively impact investor sentiment and consequently, demand for investment services. Competition from fintech companies is intensifying, and well-established firms will need to adapt to maintain market share. Regulatory scrutiny and potential compliance costs could place a burden on the sector's profitability. Changes in investment strategies, client preferences, and evolving market conditions may also create unforeseen challenges. Therefore, while a moderate growth forecast exists, the precise magnitude of this growth remains contingent on various external factors.
Key metrics that will influence the index's trajectory include macroeconomic indicators such as GDP growth, inflation rates, and unemployment figures. Performance of the broader stock market, interest rates, and investor sentiment are crucial for assessing the index's future direction. The rate of innovation and adoption of technology within the financial services industry will significantly impact the competitive landscape and, consequently, profitability. Changes in client demographics, preferences, and behaviors will influence the demand for specific investment services. Therefore, analysts and investors need to closely monitor these indicators to assess the overall health of the investment services sector and, in turn, the future trajectory of the index.
Predicting the future performance of the Dow Jones U.S. Select Investment Services Index with complete certainty is impossible. The positive outlook rests on sustained consumer confidence, moderate economic growth, and ongoing adaptability within the sector. However, potential risks include significant market volatility, heightened regulatory pressures, and intense competition from fintech upstarts. Investor sentiment shifts, economic downturns, and geopolitical instability could all contribute to a more negative outlook. A scenario involving a significant economic downturn or prolonged period of high inflation could lead to a substantial reduction in investor activity and consequently, reduced profitability for companies in the index. Ultimately, the index's forecast hinges on the management of these variables over the coming year. A more nuanced understanding is gained by considering potential scenarios: sustained growth versus substantial headwinds, for example.
Rating | Short-Term | Long-Term Senior |
---|---|---|
Outlook | B2 | Ba1 |
Income Statement | B3 | B3 |
Balance Sheet | Caa2 | Baa2 |
Leverage Ratios | Ba3 | Baa2 |
Cash Flow | B1 | Baa2 |
Rates of Return and Profitability | B2 | B2 |
*An aggregate rating for an index summarizes the overall sentiment towards the companies it includes. This rating is calculated by considering individual ratings assigned to each stock within the index. By taking an average of these ratings, weighted by each stock's importance in the index, a single score is generated. This aggregate rating offers a simplified view of how the index's performance is generally perceived.
How does neural network examine financial reports and understand financial state of the company?
References
- Abadie A, Diamond A, Hainmueller J. 2010. Synthetic control methods for comparative case studies: estimat- ing the effect of California's tobacco control program. J. Am. Stat. Assoc. 105:493–505
- M. L. Littman. Friend-or-foe q-learning in general-sum games. In Proceedings of the Eighteenth International Conference on Machine Learning (ICML 2001), Williams College, Williamstown, MA, USA, June 28 - July 1, 2001, pages 322–328, 2001
- Arjovsky M, Bottou L. 2017. Towards principled methods for training generative adversarial networks. arXiv:1701.04862 [stat.ML]
- S. Devlin, L. Yliniemi, D. Kudenko, and K. Tumer. Potential-based difference rewards for multiagent reinforcement learning. In Proceedings of the Thirteenth International Joint Conference on Autonomous Agents and Multiagent Systems, May 2014
- Athey S, Blei D, Donnelly R, Ruiz F. 2017b. Counterfactual inference for consumer choice across many prod- uct categories. AEA Pap. Proc. 108:64–67
- Künzel S, Sekhon J, Bickel P, Yu B. 2017. Meta-learners for estimating heterogeneous treatment effects using machine learning. arXiv:1706.03461 [math.ST]
- Armstrong, J. S. M. C. Grohman (1972), "A comparative study of methods for long-range market forecasting," Management Science, 19, 211–221.