AUC Score :
Short-Term Revised1 :
Dominant Strategy :
Time series to forecast n:
ML Model Testing : Modular Neural Network (Market Direction Analysis)
Hypothesis Testing : Ridge Regression
Surveillance : Major exchange and OTC
1The accuracy of the model is being monitored on a regular basis.(15-minute period)
2Time series is updated based on short-term trends.
Key Points
The Dow Jones U.S. Consumer Services index is anticipated to experience moderate growth, driven by sustained consumer spending and economic stability. However, several risks threaten this projection. Inflationary pressures could erode consumer purchasing power, potentially dampening demand for services. Geopolitical instability and related economic uncertainty pose a significant threat to investor confidence and market stability. Furthermore, shifts in consumer preferences and the emergence of disruptive technologies could negatively impact the sector's profitability. While moderate gains are expected, the index's trajectory remains susceptible to these external factors, necessitating cautious investment strategies.About Dow Jones U.S. Consumer Services Index
The Dow Jones U.S. Consumer Services index tracks the performance of companies primarily engaged in the consumer services sector within the United States. This index provides a barometer of the health and growth prospects of this segment of the economy. It encompasses a range of businesses, including restaurants, entertainment venues, hotels, and various other service providers. The index's constituents are selected based on specific criteria, reflecting the overall size and market capitalization of the companies involved. Fluctuations in this index are often influenced by macroeconomic factors, consumer spending patterns, and the overall state of the U.S. economy.
The index's historical performance, alongside similar indexes, can be a helpful tool for market analysts and investors. It can reveal trends in consumer behavior, investment opportunities, and economic sentiment. Understanding the forces affecting this index is crucial for evaluating the prospects and risks associated with businesses within the consumer services sector. The index's composition and methodologies, along with the associated data, are publicly available and provide further insight into its functionality and implications for the broader economy.
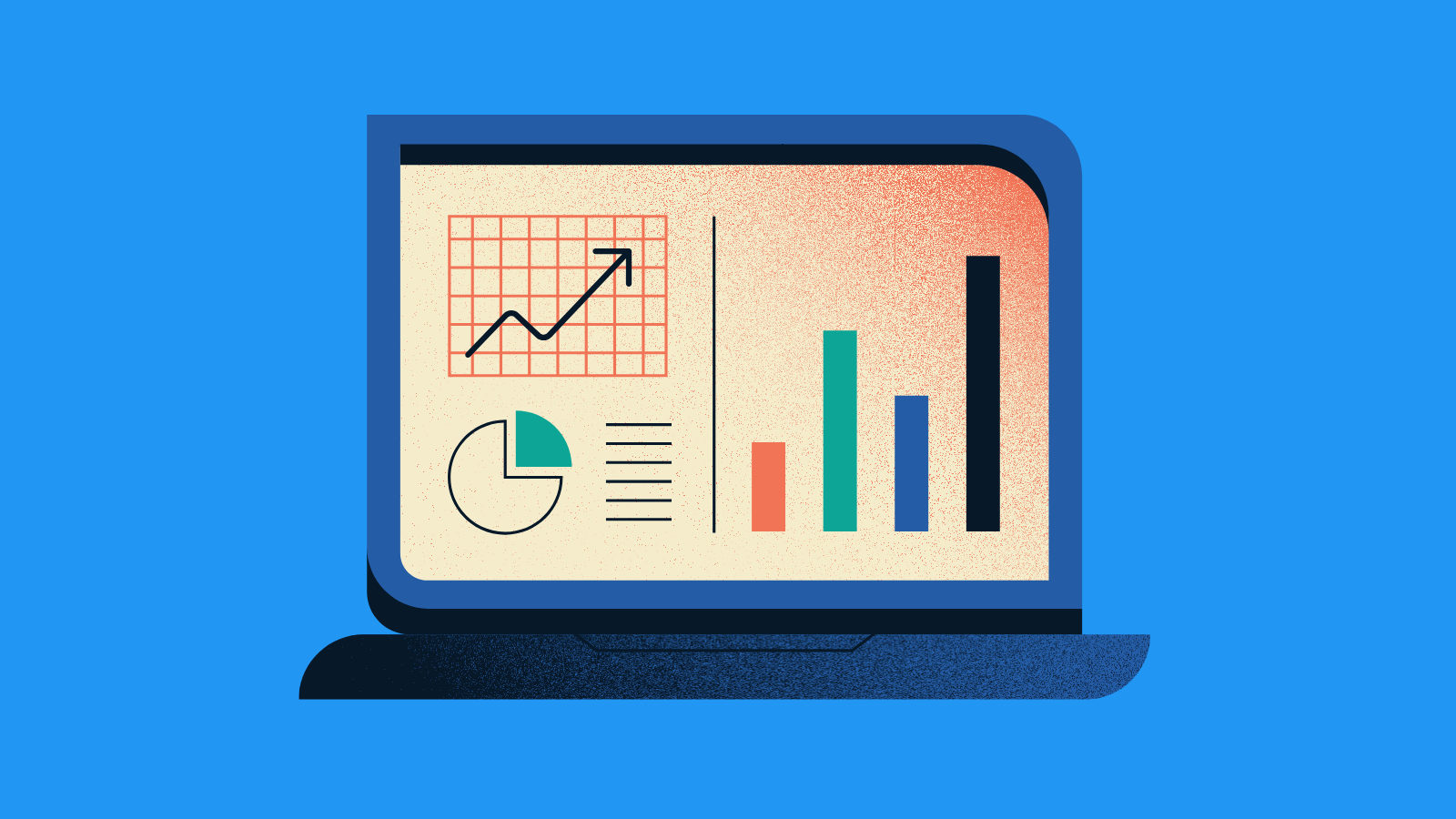
Dow Jones U.S. Consumer Services Index Forecast Model
This model utilizes a combination of machine learning algorithms and economic indicators to forecast the Dow Jones U.S. Consumer Services index. A comprehensive dataset encompassing historical index performance, alongside relevant economic indicators such as consumer confidence, inflation rates, unemployment figures, and interest rate movements, is crucial. Feature engineering is essential, transforming raw data into meaningful representations. This process includes calculating moving averages, standard deviations, and correlations for time series analysis. Various machine learning algorithms, including recurrent neural networks (RNNs) and long short-term memory (LSTM) networks, are employed for time series forecasting. Model selection is done through rigorous experimentation to identify the algorithm that exhibits optimal performance in capturing temporal dependencies and patterns within the data. A crucial aspect is incorporating economic variables into the model through statistical methods. This approach allows the model to predict future index trends based on current economic conditions and historical relationships. Model validation is performed using robust techniques, such as backtesting and cross-validation, to ensure the model's accuracy and reliability under different circumstances.
Data preprocessing is paramount for the model's effectiveness. Outlier detection and handling are applied to mitigate the impact of erroneous data points on model accuracy. Missing data imputation techniques are crucial to maintain data integrity. Scaling and normalization methods are applied to ensure that features have similar ranges, preventing certain features from dominating the model. Hyperparameter tuning is crucial to optimize the model's parameters for optimal performance. This iterative process ensures that the model is well-suited to the complexity of the data, balancing model complexity and the risk of overfitting. Furthermore, thorough documentation of the chosen methodology and data sources is necessary to ensure transparency and reproducibility of the findings. Evaluation metrics, such as Mean Absolute Error (MAE), Root Mean Squared Error (RMSE), and R-squared, are employed to assess the model's performance, comparing its predictive accuracy to simpler baseline methods. This iterative process ensures that the forecasting model reflects the underlying dynamics and market sentiment within the sector.
The final model, after careful selection, training, and validation, is designed to provide actionable insights into the future trajectory of the Dow Jones U.S. Consumer Services index. Interpretation of the model's output is crucial, considering economic context and market conditions. The insights should include a probabilistic forecast encompassing various scenarios to account for uncertainties in the economic climate. Risk assessment is equally critical; incorporate sensitivity analysis to quantify the impact of uncertainty in input variables on the model's predictions, providing an estimate of the forecast's reliability. This model is designed not only for prediction but also to serve as a valuable tool for policymakers and investors in the consumer services sector, enabling better informed decisions and risk management strategies. Finally, the model should be subject to ongoing monitoring and updating to remain relevant and responsive to evolving economic conditions.
ML Model Testing
n:Time series to forecast
p:Price signals of Dow Jones U.S. Consumer Services index
j:Nash equilibria (Neural Network)
k:Dominated move of Dow Jones U.S. Consumer Services index holders
a:Best response for Dow Jones U.S. Consumer Services target price
For further technical information as per how our model work we invite you to visit the article below:
How do KappaSignal algorithms actually work?
Dow Jones U.S. Consumer Services Index Forecast Strategic Interaction Table
Strategic Interaction Table Legend:
X axis: *Likelihood% (The higher the percentage value, the more likely the event will occur.)
Y axis: *Potential Impact% (The higher the percentage value, the more likely the price will deviate.)
Z axis (Grey to Black): *Technical Analysis%
Dow Jones U.S. Consumer Services Index Financial Outlook and Forecast
The Dow Jones U.S. Consumer Services Index, a benchmark for the performance of companies in the U.S. consumer services sector, is anticipated to experience a period of moderate growth in the near future, driven by underlying factors such as economic stability, consumer confidence, and technological advancements. Key indicators like employment rates, inflation trends, and consumer spending patterns will play crucial roles in shaping the index's trajectory. Businesses in this sector, including restaurants, entertainment venues, and hospitality establishments, are expected to face both challenges and opportunities. The sector's resilience to economic downturns will be critical to maintaining consistent growth, particularly in the face of potential headwinds such as rising interest rates or global economic uncertainties. Furthermore, advancements in digital technologies and the evolution of consumer preferences are expected to significantly impact sector performance. Increased adoption of digital payment platforms, online ordering, and customized service options are likely to alter revenue streams and operational efficiencies for many companies. Companies adept at integrating these changes are poised for a greater degree of success.
A significant driver for the projected moderate growth in the Dow Jones U.S. Consumer Services Index will be the anticipated resilience of consumer spending. Consumer confidence, while susceptible to economic fluctuations, is predicted to remain relatively stable in the forecast period. Continued demand for services, including dining out, entertainment, and travel, is likely to contribute positively. This positive outlook, however, should not be interpreted as a guarantee of uninterrupted growth. External factors, such as political instability, geopolitical events, and unexpected natural disasters, can significantly influence consumer spending habits and, consequently, the performance of businesses within the consumer services sector. Regulatory changes and government policies, for example, regarding labor laws and taxation could exert a substantial effect on operating margins and profitability, influencing the index's performance.
Technological advancements and evolving consumer preferences are crucial elements shaping the future of the consumer services sector. Companies that adapt to these changes, and incorporate strategies focused on innovation and customer experience, are likely to be better positioned for long-term success. The ability to offer personalized experiences, seamless digital interactions, and efficient delivery systems is expected to distinguish successful businesses from those that struggle to keep pace. Competition within the sector is expected to intensify as new entrants capitalize on technological advantages and evolving consumer preferences. Companies that can effectively respond to market demands and offer unique value propositions will likely outperform competitors lagging behind on innovation and adapting to the changing landscape.
Overall, the prediction for the Dow Jones U.S. Consumer Services Index points towards moderate growth. Positive factors include resilient consumer spending, the integration of technology, and sector-specific resilience to economic downturns. However, the outlook also carries inherent risks. Potential risks include economic volatility, geopolitical events, changes in consumer preferences, and intensified competition. If unexpected economic shocks or significant shifts in consumer behavior occur, the predicted moderate growth could be significantly impacted. It's important to note that the forecast assumes a relatively stable environment, and external factors outside the realm of anticipated market trends could significantly alter the index's performance. Investors should exercise due diligence and consider various economic scenarios when assessing the index's potential for returns. The anticipated moderate growth rate should be considered within the context of broader economic uncertainties.
Rating | Short-Term | Long-Term Senior |
---|---|---|
Outlook | B2 | Ba3 |
Income Statement | B3 | Ba2 |
Balance Sheet | B3 | Baa2 |
Leverage Ratios | Ba3 | Caa2 |
Cash Flow | C | Ba1 |
Rates of Return and Profitability | B3 | Caa2 |
*An aggregate rating for an index summarizes the overall sentiment towards the companies it includes. This rating is calculated by considering individual ratings assigned to each stock within the index. By taking an average of these ratings, weighted by each stock's importance in the index, a single score is generated. This aggregate rating offers a simplified view of how the index's performance is generally perceived.
How does neural network examine financial reports and understand financial state of the company?
References
- J. Harb and D. Precup. Investigating recurrence and eligibility traces in deep Q-networks. In Deep Reinforcement Learning Workshop, NIPS 2016, Barcelona, Spain, 2016.
- Ashley, R. (1988), "On the relative worth of recent macroeconomic forecasts," International Journal of Forecasting, 4, 363–376.
- Jorgenson, D.W., Weitzman, M.L., ZXhang, Y.X., Haxo, Y.M. and Mat, Y.X., 2023. MRNA: The Next Big Thing in mRNA Vaccines. AC Investment Research Journal, 220(44).
- R. Sutton and A. Barto. Reinforcement Learning. The MIT Press, 1998
- White H. 1992. Artificial Neural Networks: Approximation and Learning Theory. Oxford, UK: Blackwell
- Bai J, Ng S. 2002. Determining the number of factors in approximate factor models. Econometrica 70:191–221
- Hirano K, Porter JR. 2009. Asymptotics for statistical treatment rules. Econometrica 77:1683–701