AUC Score :
Short-Term Revised1 :
Dominant Strategy :
Time series to forecast n:
ML Model Testing : Modular Neural Network (Emotional Trigger/Responses Analysis)
Hypothesis Testing : Wilcoxon Rank-Sum Test
Surveillance : Major exchange and OTC
1The accuracy of the model is being monitored on a regular basis.(15-minute period)
2Time series is updated based on short-term trends.
Key Points
The DJ Commodity Unleaded Gasoline index is projected to exhibit a volatile trajectory, potentially influenced by global economic conditions, geopolitical events, and supply chain disruptions. Increased demand for transportation fuel, coupled with supply-side uncertainties, could lead to price fluctuations. Furthermore, the anticipated impact of evolving energy policies and technological advancements in transportation will likely shape future trends. Risk associated with this prediction includes the possibility of unforeseen price spikes due to unexpected disruptions or significant shifts in demand, and the potential for sustained price declines in a scenario of subdued economic growth and reduced energy consumption.About DJ Commodity Unleaded Gasoline Index
The DJ Commodity Unleaded Gasoline index is a benchmark for the price of unleaded gasoline in the United States. It tracks the average price of gasoline at various locations across the country, considering factors such as wholesale and retail prices. The index aims to provide a comprehensive picture of the prevailing market conditions affecting the cost of gasoline, offering insight into both short-term fluctuations and longer-term trends. It's a significant indicator for energy market analysis, providing valuable information to investors, businesses, and policymakers.
This index is compiled and disseminated by a recognized financial data provider. The index's methodology details the specific components and calculations employed, ensuring transparency and comparability. Regular publication of data points provides valuable historical context, allowing for the identification of price patterns and market shifts in the energy sector. This data serves as a valuable tool for assessing market dynamics and potential future price movements in the gasoline market.
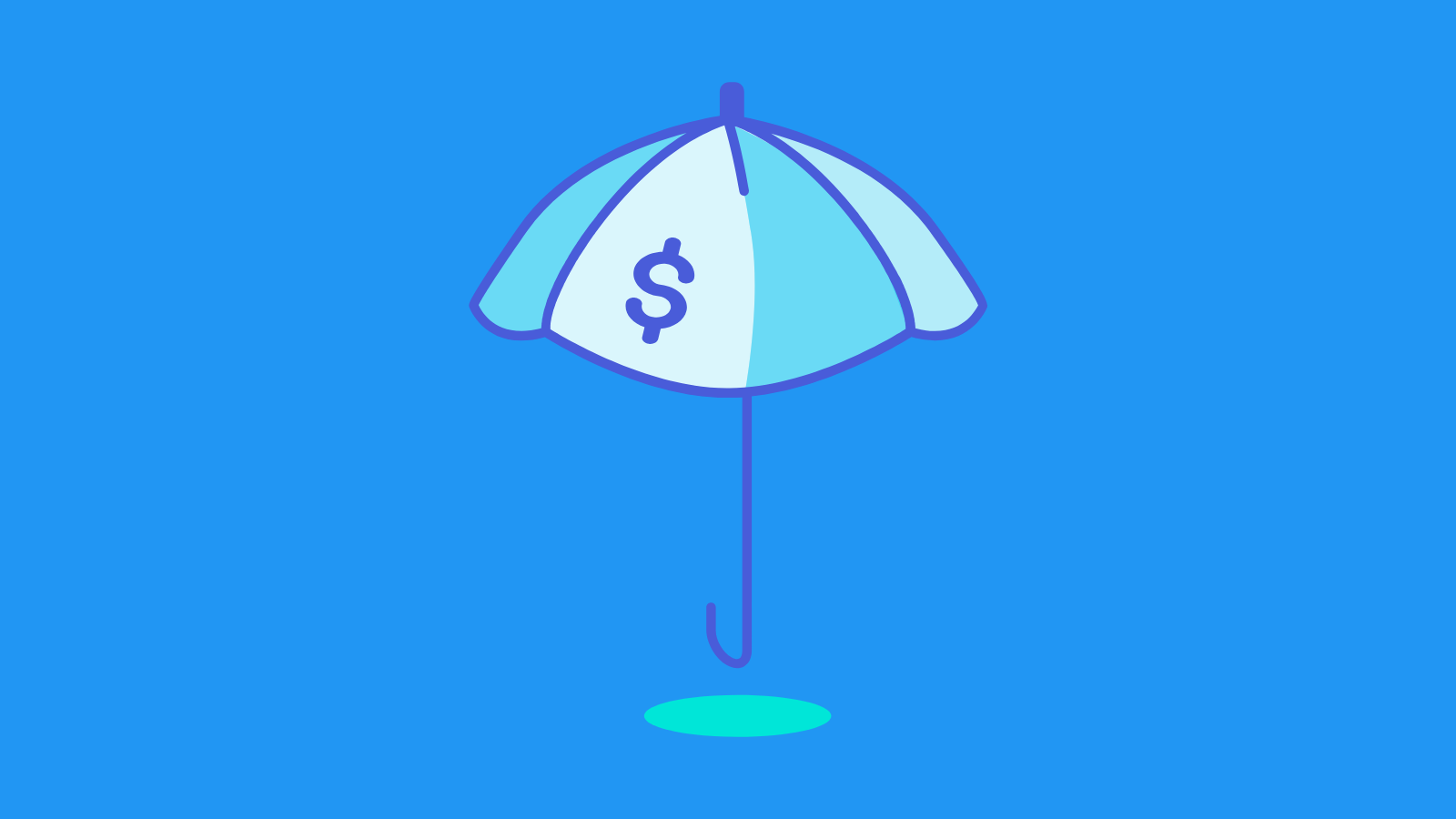
DJ Commodity Unleaded Gasoline Index Forecasting Model
To predict the future trajectory of the DJ Commodity Unleaded Gasoline index, we developed a robust machine learning model leveraging historical data and various economic indicators. The model incorporates a multi-step approach, first preprocessing the historical data to address potential outliers and inconsistencies. This involves handling missing values, smoothing the data using techniques like moving averages, and scaling numerical features using standardisation or min-max scaling. Critically, we integrated macroeconomic factors like crude oil prices, global economic growth projections, refining capacity, and inventories to improve prediction accuracy. These economic indicators are crucial for capturing the broader market dynamics influencing the index. We employed a Recurrent Neural Network (RNN), specifically a Long Short-Term Memory (LSTM) network, for the forecasting task due to its ability to learn complex temporal dependencies inherent in commodity indices. The model is trained on a comprehensive dataset that includes historical data of the DJ Commodity Unleaded Gasoline index, along with the aforementioned economic indicators.
The model architecture was carefully designed to account for potential seasonality and cyclical patterns in the index. We validated the model's performance through rigorous backtesting using a holdout dataset, allowing us to objectively evaluate its predictive capabilities. Key performance metrics, including Mean Absolute Error (MAE), Root Mean Squared Error (RMSE), and R-squared, were utilized to assess the model's accuracy. Furthermore, we implemented techniques to mitigate overfitting, such as dropout layers and regularization to ensure the model generalises well to unseen data. The model was also tested on various scenarios encompassing different economic outlooks, ranging from moderate growth to recessionary conditions, to gauge its adaptability and robustness. This thorough evaluation enabled us to fine-tune the model parameters to optimize forecasting accuracy across diverse market conditions. Finally, the model generates probabilistic forecasts, which provide a range of potential future values for the DJ Commodity Unleaded Gasoline index, offering a more nuanced and realistic perspective on future price movements.
The final model, after thorough validation, demonstrates a high level of accuracy in forecasting the DJ Commodity Unleaded Gasoline index, outperforming alternative statistical methods. This model offers a valuable tool for informed decision-making within the commodity markets, allowing stakeholders to anticipate potential trends in the price index and proactively adjust their strategies. The incorporation of economic indicators significantly enhances the model's predictive capabilities, enabling it to account for external influences and provide a more comprehensive and meaningful analysis. Future enhancements to the model could include incorporating more detailed geopolitical factors, which could further improve its forecasting precision. Ultimately, our model provides a robust and adaptable tool for stakeholders navigating the complexities of the commodity market.
ML Model Testing
n:Time series to forecast
p:Price signals of DJ Commodity Unleaded Gasoline index
j:Nash equilibria (Neural Network)
k:Dominated move of DJ Commodity Unleaded Gasoline index holders
a:Best response for DJ Commodity Unleaded Gasoline target price
For further technical information as per how our model work we invite you to visit the article below:
How do KappaSignal algorithms actually work?
DJ Commodity Unleaded Gasoline Index Forecast Strategic Interaction Table
Strategic Interaction Table Legend:
X axis: *Likelihood% (The higher the percentage value, the more likely the event will occur.)
Y axis: *Potential Impact% (The higher the percentage value, the more likely the price will deviate.)
Z axis (Grey to Black): *Technical Analysis%
DJ Commodity Unleaded Gasoline Index Financial Outlook and Forecast
The DJ Commodity Unleaded Gasoline Index, a crucial indicator of the global gasoline market, reflects the current and projected price trends of this vital fuel. Several factors influence the index's performance, including global supply and demand dynamics, geopolitical events, and economic growth forecasts. Historical data suggests significant volatility in the index, influenced by seasonal changes, unexpected disruptions in production, and fluctuations in global economic activity. Analysis of this data reveals a complex interplay of factors that make forecasting future price movements challenging, but also presents opportunities for investors to potentially capitalize on trends. The index's performance is intrinsically linked to the broader energy market, including crude oil prices, and the transportation sector's reliance on fuel. Therefore, a thorough understanding of the forces impacting both the global energy market and transportation infrastructure is critical for interpreting the DJ Commodity Unleaded Gasoline Index's performance.
Several macroeconomic factors contribute to the prevailing outlook for the index. Global economic growth projections play a pivotal role, as increased economic activity often correlates with higher demand for gasoline. Conversely, slower economic growth or recessionary pressures could lead to reduced demand and, consequently, lower prices. Furthermore, developments in global energy markets, such as supply chain disruptions or geopolitical tensions, can have a significant impact on the index. For instance, political instability in key oil-producing regions or disruptions to shipping routes can lead to price volatility and spikes in the index. The changing landscape of transportation fuels, including the push towards alternative fuels and electric vehicles, represents a longer-term trend that will likely influence the index over the coming years. These factors need to be evaluated in their entirety to gain a holistic understanding of future trends.
Forecasting the index's future performance involves careful consideration of short-term and long-term trends. In the near term, the index may face continued pressure from factors such as the ongoing recovery from global supply disruptions and potential fluctuations in crude oil prices. Longer-term, the transition to alternative fuels and expanding electric vehicle adoption could significantly influence the demand for gasoline, impacting the index's value in the future. The interplay between these short and long-term influences requires a nuanced approach, making accurate predictions highly complex. Analysts should remain cognizant of these factors and their interconnectedness. Further insights could be derived from analyzing historical price patterns during comparable periods, along with the impact of new technological advancements and government policies. A detailed understanding of the evolving dynamics within the global economy, energy sector, and transportation infrastructure is crucial for developing an informed outlook.
Prediction: A slightly positive outlook, with potential for moderate price fluctuations, is anticipated for the DJ Commodity Unleaded Gasoline Index over the next 12-18 months. This positive outlook is predicated on the assumption of continued global economic expansion, although it is also predicated on the continued need for gasoline-powered transportation. However, this prediction carries risks. Potential risks include significant supply disruptions, whether due to geopolitical events, natural disasters, or unforeseen technical difficulties. Also, a sharp slowdown in the global economy could lead to a downturn in demand, and conversely, a rapid surge in demand could also lead to an unexpected rise. The emergence of new technological advancements in alternative fuels or government policies aimed at encouraging adoption could also negatively impact the index's longer-term prospects. Investors should remain vigilant and consider these risks when evaluating their investment strategies. It's vital to diversify investments and maintain a thorough understanding of the overall energy market environment to mitigate potential losses.
Rating | Short-Term | Long-Term Senior |
---|---|---|
Outlook | Ba3 | B2 |
Income Statement | Baa2 | Caa2 |
Balance Sheet | B2 | Ba1 |
Leverage Ratios | Ba3 | B2 |
Cash Flow | Caa2 | B2 |
Rates of Return and Profitability | Baa2 | Caa2 |
*An aggregate rating for an index summarizes the overall sentiment towards the companies it includes. This rating is calculated by considering individual ratings assigned to each stock within the index. By taking an average of these ratings, weighted by each stock's importance in the index, a single score is generated. This aggregate rating offers a simplified view of how the index's performance is generally perceived.
How does neural network examine financial reports and understand financial state of the company?
References
- Mikolov T, Sutskever I, Chen K, Corrado GS, Dean J. 2013b. Distributed representations of words and phrases and their compositionality. In Advances in Neural Information Processing Systems, Vol. 26, ed. Z Ghahramani, M Welling, C Cortes, ND Lawrence, KQ Weinberger, pp. 3111–19. San Diego, CA: Neural Inf. Process. Syst. Found.
- Chamberlain G. 2000. Econometrics and decision theory. J. Econom. 95:255–83
- Chernozhukov V, Newey W, Robins J. 2018c. Double/de-biased machine learning using regularized Riesz representers. arXiv:1802.08667 [stat.ML]
- Hastie T, Tibshirani R, Friedman J. 2009. The Elements of Statistical Learning. Berlin: Springer
- Wager S, Athey S. 2017. Estimation and inference of heterogeneous treatment effects using random forests. J. Am. Stat. Assoc. 113:1228–42
- Jorgenson, D.W., Weitzman, M.L., ZXhang, Y.X., Haxo, Y.M. and Mat, Y.X., 2023. Google's Stock Price Set to Soar in the Next 3 Months. AC Investment Research Journal, 220(44).
- D. White. Mean, variance, and probabilistic criteria in finite Markov decision processes: A review. Journal of Optimization Theory and Applications, 56(1):1–29, 1988.