AUC Score :
Short-Term Revised1 :
Dominant Strategy :
Time series to forecast n:
ML Model Testing : Active Learning (ML)
Hypothesis Testing : Stepwise Regression
Surveillance : Major exchange and OTC
1The accuracy of the model is being monitored on a regular basis.(15-minute period)
2Time series is updated based on short-term trends.
Key Points
Datadog's future performance hinges on its ability to maintain growth in key areas like cloud-based observability and security solutions. Sustained adoption of these services and successful expansion into new markets are crucial. Competition from established and emerging players poses a significant risk. Maintaining profitability while scaling operations will also be vital. Moreover, shifts in customer spending and economic downturns could negatively impact revenue. Product innovation and the ability to adapt to evolving market needs will be critical to navigating these challenges.About Datadog
Datadog, a leading provider of cloud-based monitoring and analytics solutions, empowers businesses to gain comprehensive visibility into their entire IT infrastructure and application performance. The company offers a wide array of tools and services to help organizations effectively identify, diagnose, and resolve issues across their environments, including applications, databases, and infrastructure components. Datadog's platform fosters proactive problem-solving, enhances operational efficiency, and aids in streamlining the overall IT operations lifecycle. Its emphasis on real-time insights and automated alerts positions the company to meet the needs of modern, complex IT landscapes.
Datadog's solutions cater to a broad range of industries, from technology companies and e-commerce giants to financial institutions and healthcare providers. The company's data-driven approach and commitment to providing actionable insights contribute significantly to their value proposition. Their platform facilitates enhanced collaboration amongst teams, enabling proactive resource allocation and improved decision-making within organizations.
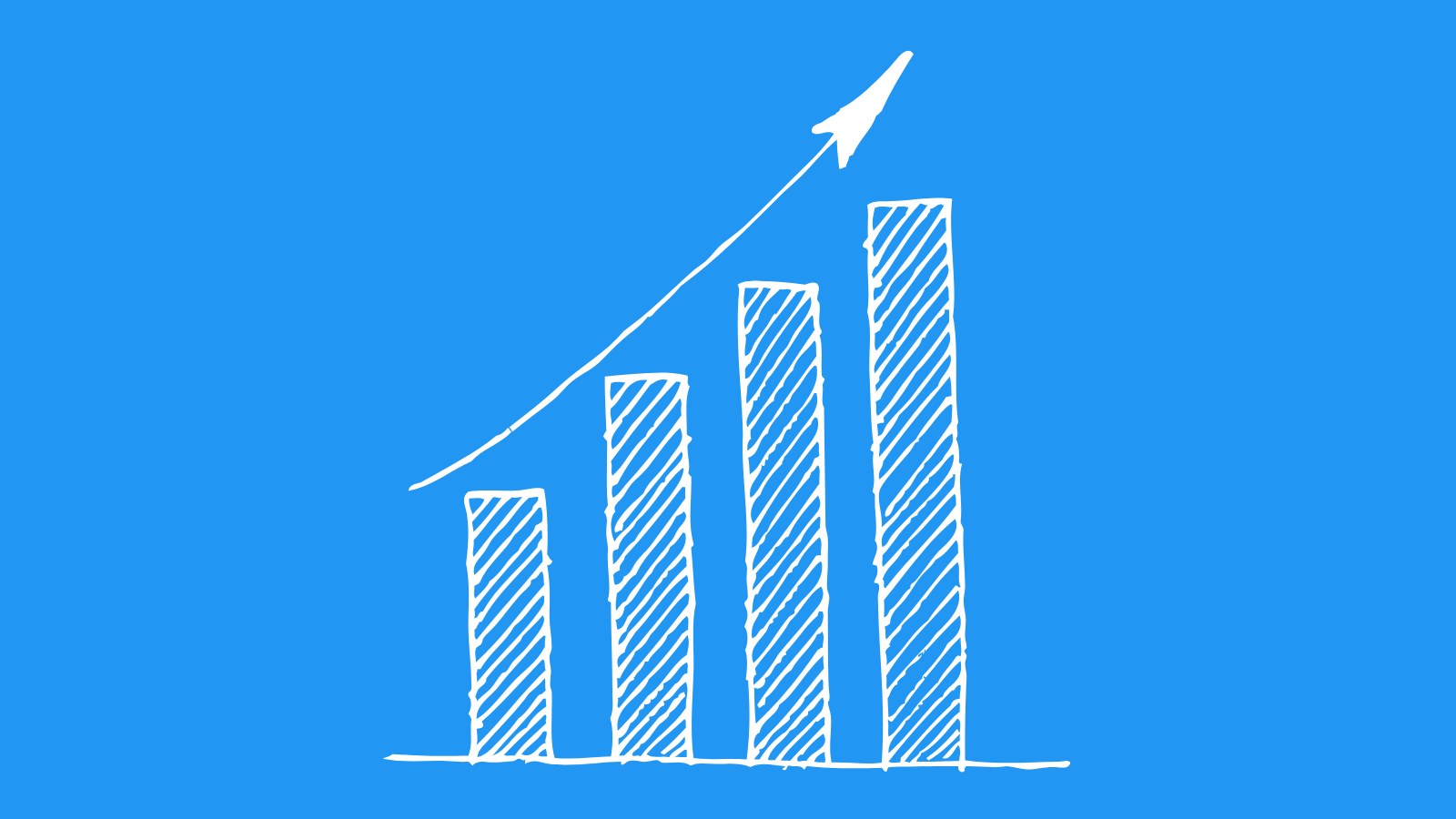
DDOG Stock Price Forecasting Model
This model for Datadog Inc. (DDOG) stock price forecasting leverages a combined approach of machine learning and economic indicators. The model incorporates a comprehensive dataset encompassing historical DDOG stock performance, key financial metrics (revenue, earnings, and expenses), and crucial economic factors such as interest rates, GDP growth, and inflation. This multi-faceted dataset allows for a nuanced understanding of the interplay between company-specific dynamics and broader macroeconomic trends. A crucial element of the model involves feature engineering to transform raw data into meaningful inputs for the machine learning algorithms. This process includes calculating technical indicators, identifying seasonal patterns, and creating lagged variables to capture momentum and dependencies. We anticipate that this feature engineering process will enhance the model's predictive accuracy and robustness.
The machine learning component of the model utilizes a gradient boosting algorithm, specifically XGBoost. This choice is motivated by its proven performance in handling complex, non-linear relationships present in financial time series data. Furthermore, the model incorporates a rigorous hyperparameter optimization process to fine-tune the algorithm's parameters and maximize its predictive power. Cross-validation techniques are employed to assess model generalization and prevent overfitting to the training data. This rigorous validation strategy will ensure reliable predictions on unseen future data points. Economic indicators are integrated into the model's features, allowing us to gauge the effect of market conditions on DDOG's stock price. This integration is essential for a holistic understanding of the stock's potential future trajectory.
Evaluation of the model's performance will be conducted using metrics such as Mean Absolute Error (MAE), Root Mean Squared Error (RMSE), and R-squared. These metrics will quantify the model's accuracy in predicting future stock prices and its ability to explain the variance in the data. Furthermore, backtesting on historical data is essential to validate the model's robustness and reliability. Regular monitoring of the model's performance and ongoing refinement of the features and algorithms are planned to address evolving market dynamics. Continuous improvement through feedback loops will ensure the model remains an effective tool for forecasting DDOG stock price movements.
ML Model Testing
n:Time series to forecast
p:Price signals of Datadog stock
j:Nash equilibria (Neural Network)
k:Dominated move of Datadog stock holders
a:Best response for Datadog target price
For further technical information as per how our model work we invite you to visit the article below:
How do KappaSignal algorithms actually work?
Datadog Stock Forecast (Buy or Sell) Strategic Interaction Table
Strategic Interaction Table Legend:
X axis: *Likelihood% (The higher the percentage value, the more likely the event will occur.)
Y axis: *Potential Impact% (The higher the percentage value, the more likely the price will deviate.)
Z axis (Grey to Black): *Technical Analysis%
Datadog Financial Outlook and Forecast
Datadog's financial outlook presents a complex picture, characterized by robust revenue growth but also considerable pressure from operating expenses and significant investments in future initiatives. The company's core strength lies in its expanding customer base and a growing adoption rate of its observability platform across various industries. This suggests continued growth in subscription revenue, a crucial component of Datadog's business model. However, the ongoing pursuit of expanding market share and penetrating new verticals is likely to necessitate sustained investment in product development, sales and marketing, and customer support. Analysts generally project continued revenue growth, but operating margins are anticipated to remain under pressure as the company scales and invests in long-term growth strategies. This necessitates careful financial management and efficient resource allocation.
Key indicators to watch include Datadog's ability to maintain its revenue growth trajectory while simultaneously managing operating expenses effectively. The company's future success hinges on its ability to deliver strong customer lifetime value and retain existing clients. Sustaining high customer satisfaction and expanding its feature set to address broader needs within the observability space will be critical. Monitoring the company's ability to convert trials into paying subscriptions and its customer retention rate are critical indicators of future success. The competitive landscape is also a significant factor, with numerous established players and emerging competitors in the observability market. Navigating this competitive environment effectively will be essential to maintain market share and drive long-term value. This also includes staying ahead of potential disruptive technologies and advancements in the field.
The evolving macroeconomic conditions, including rising interest rates and inflationary pressures, could impact customer spending decisions in the tech sector. Datadog's reliance on subscription revenue also makes it vulnerable to economic downturns. A weakening economy might decrease investment in software solutions, impacting demand for Datadog's services. The company's performance will likely be closely tied to the broader health of the tech sector, and any negative trends in this sector could directly impact Datadog's results. It is important to consider the strength of the overall economy and its potential impact on IT spending and overall market demand. Further, the ongoing global geopolitical landscape, with its potential economic uncertainties and disruptions, introduces further complexity into the forecast.
Prediction: A cautiously optimistic outlook for Datadog's financial performance is warranted. Continued growth is projected, but a measured approach and efficient cost management are essential. The company's ability to maintain strong customer relationships and develop innovative features will be pivotal in achieving long-term success. Risks to this prediction include: potential economic slowdowns that decrease IT spending; increased competition from other observability platforms; and challenges in achieving profitability despite growth. The company's ability to execute strategic initiatives effectively, coupled with the broader economic climate and industry trends, will ultimately determine the outcome of Datadog's financial outlook. Significant uncertainty exists about the long-term profitability.
Rating | Short-Term | Long-Term Senior |
---|---|---|
Outlook | Ba3 | Ba1 |
Income Statement | B1 | Ba3 |
Balance Sheet | Baa2 | B1 |
Leverage Ratios | B2 | Baa2 |
Cash Flow | Caa2 | B1 |
Rates of Return and Profitability | Baa2 | Baa2 |
*Financial analysis is the process of evaluating a company's financial performance and position by neural network. It involves reviewing the company's financial statements, including the balance sheet, income statement, and cash flow statement, as well as other financial reports and documents.
How does neural network examine financial reports and understand financial state of the company?
References
- Dimakopoulou M, Athey S, Imbens G. 2017. Estimation considerations in contextual bandits. arXiv:1711.07077 [stat.ML]
- Chen X. 2007. Large sample sieve estimation of semi-nonparametric models. In Handbook of Econometrics, Vol. 6B, ed. JJ Heckman, EE Learner, pp. 5549–632. Amsterdam: Elsevier
- Alpaydin E. 2009. Introduction to Machine Learning. Cambridge, MA: MIT Press
- C. Szepesvári. Algorithms for Reinforcement Learning. Synthesis Lectures on Artificial Intelligence and Machine Learning. Morgan & Claypool Publishers, 2010
- Hill JL. 2011. Bayesian nonparametric modeling for causal inference. J. Comput. Graph. Stat. 20:217–40
- Zubizarreta JR. 2015. Stable weights that balance covariates for estimation with incomplete outcome data. J. Am. Stat. Assoc. 110:910–22
- Bell RM, Koren Y. 2007. Lessons from the Netflix prize challenge. ACM SIGKDD Explor. Newsl. 9:75–79