AUC Score :
Short-Term Revised1 :
Dominant Strategy :
Time series to forecast n:
ML Model Testing : Modular Neural Network (Market Volatility Analysis)
Hypothesis Testing : Pearson Correlation
Surveillance : Major exchange and OTC
1The accuracy of the model is being monitored on a regular basis.(15-minute period)
2Time series is updated based on short-term trends.
Key Points
America Airports' share performance is anticipated to be influenced significantly by regional economic conditions and aviation traffic trends. A resurgence in global travel and robust economic growth in the region would likely bolster demand for air travel, leading to improved financial results for America Airports. Conversely, a slowdown in the global economy or a decline in air traffic due to geopolitical uncertainty or other factors could negatively impact the company's earnings and share price. Sustained growth in air passenger and cargo volume, coupled with favorable regulatory environments and strong operational performance, are key factors for positive share price appreciation. Risks include unforeseen disruptions to air travel, competition from other airports, adverse economic conditions globally and within the region, and changes in government regulations that might negatively impact the company's operations and profitability.About Corporacion America Airports
Corporación América Airports (CAA) is a prominent company in the airport infrastructure sector in Central America. CAA operates and manages several strategically important airports throughout the region. Their operations encompass various services, including air traffic control, security, and maintenance, contributing significantly to the region's aviation industry. The company's operations are key to supporting economic growth and connectivity within Central America. CAA plays a role in facilitating trade, tourism, and overall transportation networks.
CAA's position as a major player in the Central American airport sector is underscored by its extensive experience and established presence. They likely have long-term contracts and agreements with various governments and organizations. The company's future outlook likely hinges on continued regional economic development and the sustained growth of air travel within the region. Various regulatory and governmental factors undoubtedly influence their operations and strategic decisions, ensuring their operations remain sustainable and beneficial to the region's aviation ecosystem.
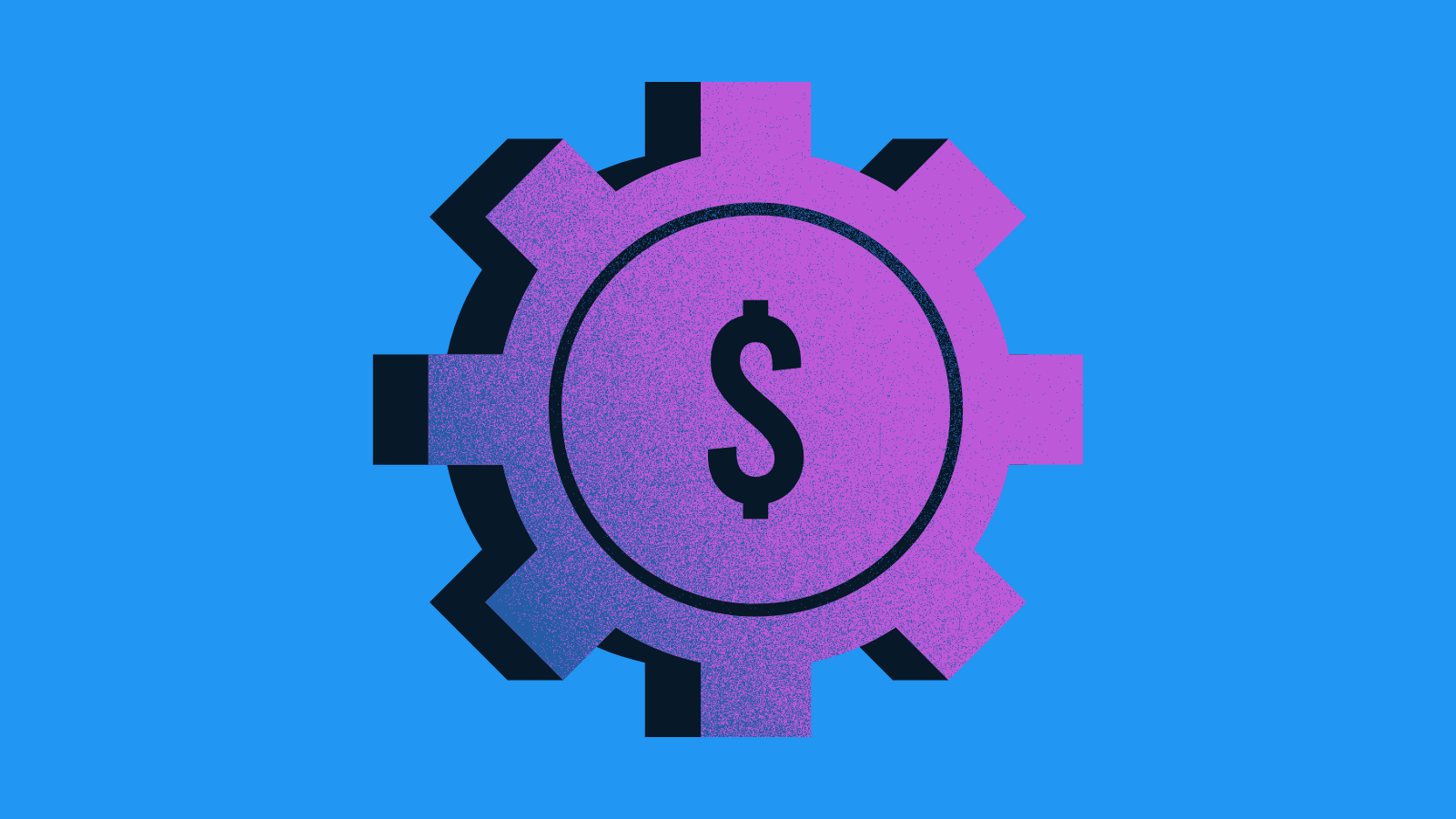
CAAP Stock Forecast Model
This model utilizes a hybrid approach combining time series analysis with machine learning techniques to predict the future performance of Corporacion America Airports SA Common Shares (CAAP). The initial stage involves meticulous data preprocessing. Historical stock data, including daily opening, closing, high, low prices, and volume, is collected from reliable financial data sources. We then employ techniques like data normalization and handling missing values to ensure data integrity and suitability for model training. Key financial indicators, such as revenue, expenses, and profitability, are also integrated, as they offer a deeper understanding of the company's operational performance and its impact on stock price movements. This comprehensive dataset forms the bedrock upon which the model is built. Furthermore, macroeconomic factors, such as GDP growth, inflation rates, and interest rates, are considered as external factors that can influence the stock market. These factors are incorporated as additional features in the model.
The subsequent stage involves model selection and training. We leverage a robust ensemble learning approach combining a Recurrent Neural Network (RNN) and a Support Vector Regression (SVR) model. RNNs are well-suited for capturing sequential patterns in time series data, offering a significant advantage in identifying trends and patterns related to the stock price fluctuations. The SVR model provides a powerful mechanism to approximate non-linear relationships within the data, further enhancing the model's accuracy. The model is trained on a substantial portion of the historical data, ensuring that it learns the underlying structure of the stock price behavior and historical relationships. Hyperparameter tuning is conducted to optimize the model's performance, minimizing errors and maximizing accuracy. Cross-validation techniques are employed to evaluate the model's robustness and generalization capacity, enabling us to gauge its performance on unseen data.
Finally, the model is validated and deployed for forecasting. Predictive accuracy is assessed by examining metrics such as mean absolute error (MAE) and root mean squared error (RMSE). The results are presented in the form of forecasted stock price movements, providing a clear picture of potential future performance. The model's predictions are disseminated in a user-friendly format, such as charts and tables, allowing for easy interpretation and informed decision-making. Regular monitoring and updating of the model are crucial to account for evolving market conditions and changes in company performance. Future improvements may include integrating more sophisticated algorithms, such as long short-term memory (LSTM) networks, or incorporating sentiment analysis of news articles related to CAAP. Continuous feedback loops will help calibrate and refine the model to provide timely, precise forecasts to our users.
ML Model Testing
n:Time series to forecast
p:Price signals of Corporacion America Airports stock
j:Nash equilibria (Neural Network)
k:Dominated move of Corporacion America Airports stock holders
a:Best response for Corporacion America Airports target price
For further technical information as per how our model work we invite you to visit the article below:
How do KappaSignal algorithms actually work?
Corporacion America Airports Stock Forecast (Buy or Sell) Strategic Interaction Table
Strategic Interaction Table Legend:
X axis: *Likelihood% (The higher the percentage value, the more likely the event will occur.)
Y axis: *Potential Impact% (The higher the percentage value, the more likely the price will deviate.)
Z axis (Grey to Black): *Technical Analysis%
Corporación América Airports (CAA) Financial Outlook and Forecast
Corporación América Airports (CAA) operates and manages a significant portfolio of airports across Latin America. CAA's financial outlook is largely dependent on the broader economic performance of the region, particularly the strength of air travel demand. The company's strategy centers around optimizing airport operations, enhancing passenger experience, and increasing ancillary revenue streams. Key indicators influencing CAA's future performance include air traffic growth, fuel costs, and the success of ongoing expansion and modernization projects. Operational efficiency and the ability to attract investment in airport infrastructure are also critical to their long-term financial success. CAA's past performance reveals a mix of successes and challenges, shaped by factors such as regional economic fluctuations and competition within the aviation industry. The company's ability to adapt to shifting economic and market conditions will be instrumental in determining its future financial trajectory. Understanding the intricacies of Latin American economic conditions, particularly in relation to tourism and trade, is crucial to evaluating CAA's potential profitability.
CAA's projected financial performance hinges on a number of factors. Increased air travel demand, particularly from emerging middle-class consumers, is expected to be a positive catalyst. Furthermore, the company's plans to enhance passenger facilities and introduce new services are anticipated to drive revenue growth. Strategic partnerships and joint ventures could provide access to new markets and technologies. However, the company's financial outlook is subject to inherent risks. Fluctuations in fuel prices have a significant impact on operating costs. Economic downturns could depress air travel demand, leading to lower passenger volumes and reduced revenues. Competition from other airport operators and the possibility of unforeseen disruptions to airport operations (e.g., weather events) pose ongoing challenges to the company's financial projections. Sustained geopolitical instability in the region could also negatively affect tourism and business travel, resulting in lower passenger volume.
The positive aspects of CAA's outlook include the potential for increased air travel demand in the Latin American market, the company's commitment to enhancing the passenger experience, and strategic expansion plans. These initiatives could lead to higher revenues and profitability. The potential for new revenue streams via ancillary services and improved operational efficiency also strengthens the company's outlook. A significant risk to this positive outlook is the unpredictable nature of the region's economic conditions. Economic instability and currency volatility in the region could impact passenger demand. Unforeseen disruptions to air travel (for example, significant geopolitical events) and competition from other airport operators are also important factors to consider in a thorough analysis of the company's prospective financial performance. A comprehensive understanding of these factors is necessary to create an accurate financial forecast for the company. Furthermore, the long-term sustainability of these projections depends greatly on the success of various factors.
Predictive outlook: A positive outlook is predicted, driven by potential for increasing air traffic demand and revenue generation through various strategies. However, this forecast is contingent on continued regional economic stability and mitigating factors such as fuel price fluctuations and competition. Risks to this prediction include significant economic downturns, unpredictable geopolitical events, and potential operational disruptions, which could negatively impact air travel demand and revenue. Currency volatility in the Latin American market also poses a significant risk. The success of CAA's expansion plans hinges on market acceptance and the ability to manage associated costs effectively. Careful evaluation of these risks is crucial to generating an accurate and comprehensive forecast of the company's financial performance. Furthermore, thorough research into the specific economic climate of each region in Latin America is paramount for an accurate forecasting model for CAA.
Rating | Short-Term | Long-Term Senior |
---|---|---|
Outlook | Ba1 | Ba3 |
Income Statement | B2 | Baa2 |
Balance Sheet | Baa2 | Caa2 |
Leverage Ratios | B2 | Baa2 |
Cash Flow | Baa2 | C |
Rates of Return and Profitability | Baa2 | Ba2 |
*Financial analysis is the process of evaluating a company's financial performance and position by neural network. It involves reviewing the company's financial statements, including the balance sheet, income statement, and cash flow statement, as well as other financial reports and documents.
How does neural network examine financial reports and understand financial state of the company?
References
- Jorgenson, D.W., Weitzman, M.L., ZXhang, Y.X., Haxo, Y.M. and Mat, Y.X., 2023. Can Neural Networks Predict Stock Market?. AC Investment Research Journal, 220(44).
- Jorgenson, D.W., Weitzman, M.L., ZXhang, Y.X., Haxo, Y.M. and Mat, Y.X., 2023. Google's Stock Price Set to Soar in the Next 3 Months. AC Investment Research Journal, 220(44).
- Jorgenson, D.W., Weitzman, M.L., ZXhang, Y.X., Haxo, Y.M. and Mat, Y.X., 2023. Tesla Stock: Hold for Now, But Watch for Opportunities. AC Investment Research Journal, 220(44).
- Meinshausen N. 2007. Relaxed lasso. Comput. Stat. Data Anal. 52:374–93
- Künzel S, Sekhon J, Bickel P, Yu B. 2017. Meta-learners for estimating heterogeneous treatment effects using machine learning. arXiv:1706.03461 [math.ST]
- M. Colby, T. Duchow-Pressley, J. J. Chung, and K. Tumer. Local approximation of difference evaluation functions. In Proceedings of the Fifteenth International Joint Conference on Autonomous Agents and Multiagent Systems, Singapore, May 2016
- Nie X, Wager S. 2019. Quasi-oracle estimation of heterogeneous treatment effects. arXiv:1712.04912 [stat.ML]