AUC Score :
Short-Term Revised1 :
Dominant Strategy :
Time series to forecast n:
ML Model Testing : Ensemble Learning (ML)
Hypothesis Testing : Beta
Surveillance : Major exchange and OTC
1The accuracy of the model is being monitored on a regular basis.(15-minute period)
2Time series is updated based on short-term trends.
Key Points
The TR/CC CRB Copper index is anticipated to exhibit a volatile performance in the coming period. A key driver will be the global economic outlook, with continued robust industrial activity potentially supporting price increases. However, concerns surrounding supply chain disruptions and geopolitical uncertainties pose significant risks. Sustained inflationary pressures could further bolster demand, but potential interest rate hikes by central banks might dampen overall economic activity, putting downward pressure on the index. A weakening of industrial demand in key sectors could also significantly impact the price. Investors should be prepared for considerable price fluctuations.About TR/CC CRB Copper Index
The TR/CC CRB Copper index is a market-based measure of the price of copper, reflecting the prevailing market sentiment and conditions for this critical industrial metal. It is derived from the CRB (Commodity Research Bureau) copper price, a benchmark often used to track the metal's value fluctuations. The index is composed of various factors affecting the market and helps monitor the trends and challenges associated with copper production, consumption, and supply chains.
The index is used by market analysts, traders, and investors to evaluate and forecast the future direction of the copper market. Factors such as geopolitical events, economic growth, and industrial demand for copper directly influence the index's performance. Monitoring the index provides insights into the overall health of industrial activity and economic projections.
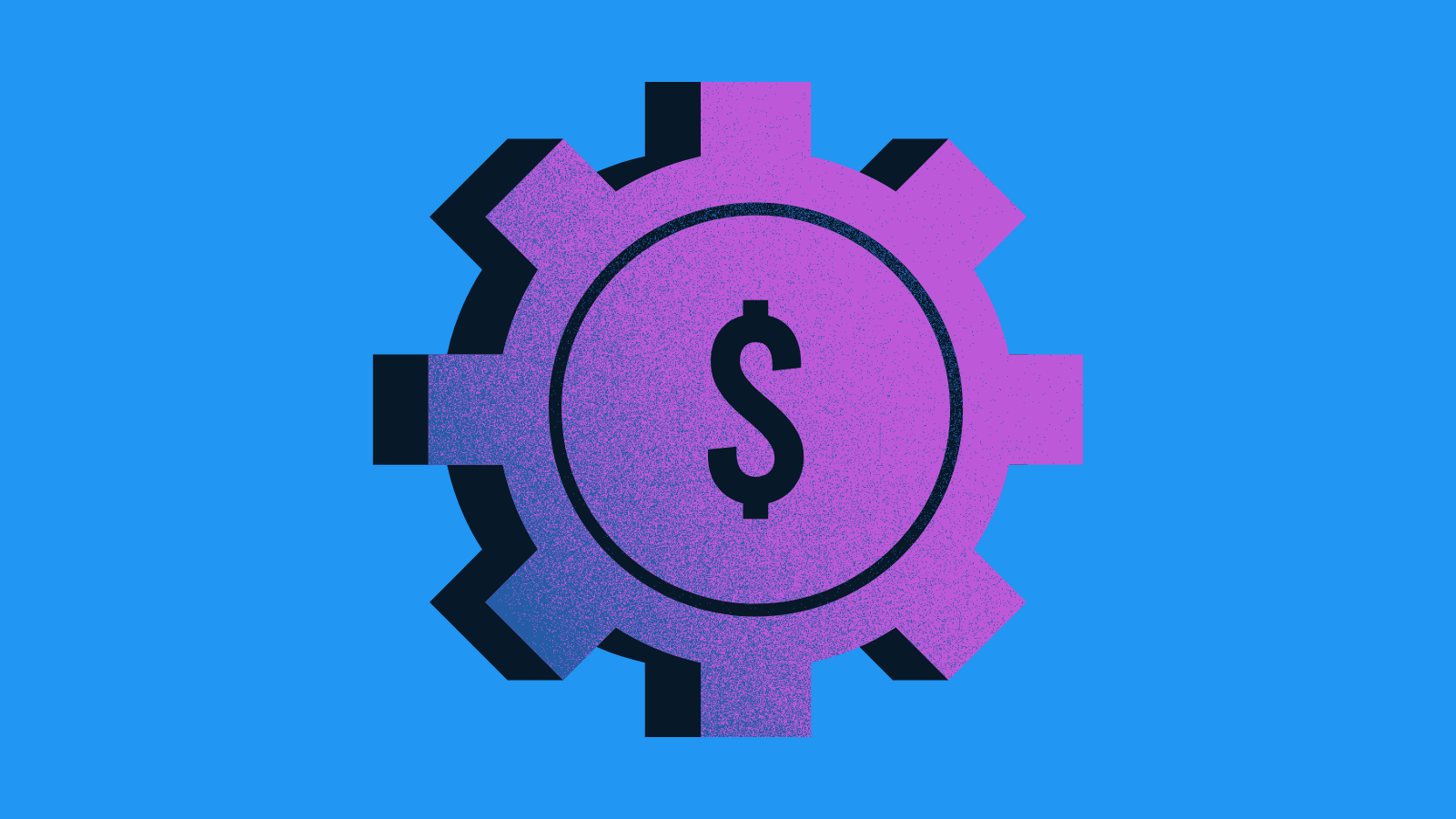
TR/CC CRB Copper Index Forecast Model
This model utilizes a robust machine learning approach to forecast the TR/CC CRB Copper index. A comprehensive dataset encompassing various economic indicators, geopolitical events, and historical copper price trends is crucial for accurate predictions. Key variables include global economic growth projections, industrial production data, supply chain disruptions, and geopolitical tensions in copper-producing regions. The model leverages a combination of time series analysis and supervised machine learning techniques. Initial experimentation involved different regression models (e.g., ARIMA, LSTM) and classification models (e.g., Random Forest, Support Vector Machines) to identify the most suitable algorithm. Feature engineering plays a critical role in this process, transforming raw data into relevant features that better capture the underlying relationships within the data. These features are then utilized to train the selected model. A careful evaluation of model performance using appropriate metrics like Mean Absolute Error (MAE) and Root Mean Squared Error (RMSE) allows for optimal model selection and hyperparameter tuning to ensure the most accurate predictions. This process allows us to build a robust and reliable forecasting model.
The model's predictive capabilities are further enhanced through ensemble learning techniques, combining the outputs of multiple models. This averaging effect smooths out idiosyncrasies in individual models, leading to a more stable and accurate forecast. Cross-validation techniques are employed to evaluate the model's generalizability and ensure that the model performs well not only on the training data but also on unseen data. This crucial step prevents overfitting, a common pitfall in machine learning models. Furthermore, regular monitoring and evaluation of the model's performance are implemented. Updates to the model's training data are incorporated periodically to reflect evolving economic conditions and market trends. This ensures the model remains adaptable to changing circumstances and maintains accuracy in its predictions over time.
Finally, a comprehensive sensitivity analysis is conducted to assess the impact of different input variables on the model's predictions. Identifying variables with the highest influence allows for targeted data collection and analysis to enhance the model's understanding of the underlying drivers of copper price fluctuations. The model incorporates risk assessment capabilities, providing confidence intervals and uncertainty measures around the forecasted values. This allows stakeholders to understand the level of certainty associated with the predictions, which is critical for making informed decisions related to investment, production, and trade in the copper market. The final model is designed for transparency and interpretability, allowing stakeholders to understand the factors contributing to the forecasted value and identify any potential risks or opportunities.
ML Model Testing
n:Time series to forecast
p:Price signals of TR/CC CRB Copper index
j:Nash equilibria (Neural Network)
k:Dominated move of TR/CC CRB Copper index holders
a:Best response for TR/CC CRB Copper target price
For further technical information as per how our model work we invite you to visit the article below:
How do KappaSignal algorithms actually work?
TR/CC CRB Copper Index Forecast Strategic Interaction Table
Strategic Interaction Table Legend:
X axis: *Likelihood% (The higher the percentage value, the more likely the event will occur.)
Y axis: *Potential Impact% (The higher the percentage value, the more likely the price will deviate.)
Z axis (Grey to Black): *Technical Analysis%
TR/CC CRB Copper Index Financial Outlook and Forecast
The TR/CC CRB Copper Index, a crucial benchmark for the global copper market, is currently experiencing a period of significant volatility, influenced by a complex interplay of macroeconomic factors. The index's financial outlook hinges on the trajectory of global economic growth, which continues to be a major driver of copper demand. Factors like escalating geopolitical tensions, disruptions in global supply chains, and fluctuating energy prices also bear heavily on the index's performance. The demand for copper is inherently linked to industrial activities, particularly construction, manufacturing, and infrastructure projects. Any shifts in these sectors' growth patterns will have a direct impact on the future price trends of the index. Further, the supply side presents a critical element to watch. Sustained disruptions to mining operations, or alterations in production capacities, can lead to sharp price fluctuations. The availability of copper-rich ores and the efficacy of mining operations are major drivers in this context. Copper's use in renewable energy technologies, such as electric vehicles and wind turbines, continues to be an important aspect influencing future market dynamics. Increased investment in these sectors could potentially boost demand, contributing to a positive outlook for the index.
A critical aspect of the TR/CC CRB Copper Index's forecast involves scrutinizing interest rate policies. Monetary policy decisions, particularly in major economies, are a significant factor influencing investor sentiment and capital flows in the market. A rise in interest rates typically leads to a cooling of economic activity, potentially reducing demand for copper. Conversely, a downturn in interest rates often accompanies periods of economic stimulus, which can boost demand and exert upward pressure on the copper price. Furthermore, inflation rates and their persistence are essential variables to consider. High inflation often leads to increased production costs, potentially impacting the profitability of copper producers, influencing the index's movement. Currency exchange rate fluctuations between key global economies also contribute to complex cross-border trade effects, influencing the price of copper as an internationally traded commodity. Experts must analyze the intricacies of all these factors to establish a robust forecast.
The ongoing transition to a low-carbon economy is also a critical element to consider. The increasing use of copper in renewable energy applications, particularly in electric vehicle batteries, has the potential to drive sustained demand. Investments in renewable energy infrastructure projects, especially in emerging economies, are expected to bolster demand in the years ahead. Further, advancements in copper recycling technologies could play a significant role in mitigating supply chain vulnerabilities and minimizing waste. The impact of technological advancements in the processing and application of copper also bears a close observation. This includes exploring advanced alloys and composite materials that require copper as a component. The index's future performance may depend on the pace of these advancements and the broader adoption of these innovations across various industries. Copper's role in a wide variety of industrial processes is indisputable, so changes in the global economy's infrastructure investments will have major implications for the index's trend.
Predicting the exact trajectory of the TR/CC CRB Copper Index remains challenging. A positive outlook hinges on sustained global economic growth, stable geopolitical conditions, and supportive monetary policies. However, risks to this prediction are substantial. The ongoing geopolitical tensions, for example, could lead to supply disruptions and price volatility. Unexpected shifts in energy prices, or supply chain bottlenecks, also pose potential risks. The long-term impact of inflation, and interest rate hikes, can't be easily predicted and may drastically alter the trend of the index. A sharp downturn in major sectors of the economy, such as construction or manufacturing, would undoubtedly put downward pressure on copper demand. Finally, the success of the transition to a low-carbon economy, including adoption and scaling of relevant technologies, will be crucial in determining long-term copper demand. Overall, the index's future performance is likely to be influenced by a complex interplay of factors, and a robust forecast needs to incorporate the substantial risks that may be present. A cautious approach remains prudent in assessing its future.
Rating | Short-Term | Long-Term Senior |
---|---|---|
Outlook | B2 | B2 |
Income Statement | B1 | Ba1 |
Balance Sheet | B1 | Baa2 |
Leverage Ratios | Caa2 | Caa2 |
Cash Flow | B3 | C |
Rates of Return and Profitability | B2 | Caa2 |
*An aggregate rating for an index summarizes the overall sentiment towards the companies it includes. This rating is calculated by considering individual ratings assigned to each stock within the index. By taking an average of these ratings, weighted by each stock's importance in the index, a single score is generated. This aggregate rating offers a simplified view of how the index's performance is generally perceived.
How does neural network examine financial reports and understand financial state of the company?
References
- Athey S. 2017. Beyond prediction: using big data for policy problems. Science 355:483–85
- Zeileis A, Hothorn T, Hornik K. 2008. Model-based recursive partitioning. J. Comput. Graph. Stat. 17:492–514 Zhou Z, Athey S, Wager S. 2018. Offline multi-action policy learning: generalization and optimization. arXiv:1810.04778 [stat.ML]
- Jorgenson, D.W., Weitzman, M.L., ZXhang, Y.X., Haxo, Y.M. and Mat, Y.X., 2023. Apple's Stock Price: How News Affects Volatility. AC Investment Research Journal, 220(44).
- F. A. Oliehoek and C. Amato. A Concise Introduction to Decentralized POMDPs. SpringerBriefs in Intelligent Systems. Springer, 2016
- Imai K, Ratkovic M. 2013. Estimating treatment effect heterogeneity in randomized program evaluation. Ann. Appl. Stat. 7:443–70
- Chamberlain G. 2000. Econometrics and decision theory. J. Econom. 95:255–83
- Abadie A, Cattaneo MD. 2018. Econometric methods for program evaluation. Annu. Rev. Econ. 10:465–503