AUC Score :
Short-Term Revised1 :
Dominant Strategy :
Time series to forecast n:
ML Model Testing : Modular Neural Network (Market Direction Analysis)
Hypothesis Testing : Spearman Correlation
Surveillance : Major exchange and OTC
1The accuracy of the model is being monitored on a regular basis.(15-minute period)
2Time series is updated based on short-term trends.
Key Points
Xponential Fitness's future performance hinges on its ability to navigate the evolving fitness landscape. Strong growth in the rapidly expanding at-home fitness market, coupled with successful integration of recently acquired studios and effective marketing strategies, could drive revenue and membership increases. However, intense competition from established fitness chains and emerging digital fitness platforms presents a significant risk. Maintaining brand loyalty and adapting to changing consumer preferences will be crucial. Operational efficiency, including cost control and studio management, is essential for profitability. Furthermore, fluctuating macroeconomic conditions and potential unforeseen events could negatively impact consumer spending and gym memberships, posing a considerable risk to Xponential Fitness's profitability.About Xponential Fitness
Xponential Fitness, a publicly traded company, operates as a fitness franchisor and owner. It primarily focuses on providing fitness studio services across diverse brands, which are designed to cater to varied fitness goals and preferences. The company's strategic approach includes developing strong brand recognition and establishing a loyal customer base. Xponential Fitness emphasizes the importance of creating a supportive and motivating environment for its members through its various programs and initiatives. Their model typically involves a combination of group fitness classes, personal training, and other related services. The company's success depends on its ability to attract new members and maintain their engagement.
Key aspects of Xponential Fitness's business strategy include establishing a recognizable brand image, fostering a sense of community among its members, and providing a comprehensive suite of fitness services. They aim to drive profitability by maximizing efficiency in operations and leveraging economies of scale across their various brands. Xponential Fitness strives to cater to evolving consumer demands in the fitness industry while maintaining consistent revenue generation. The company's future success will depend on continued innovation in its services and adaptability to changing market trends.
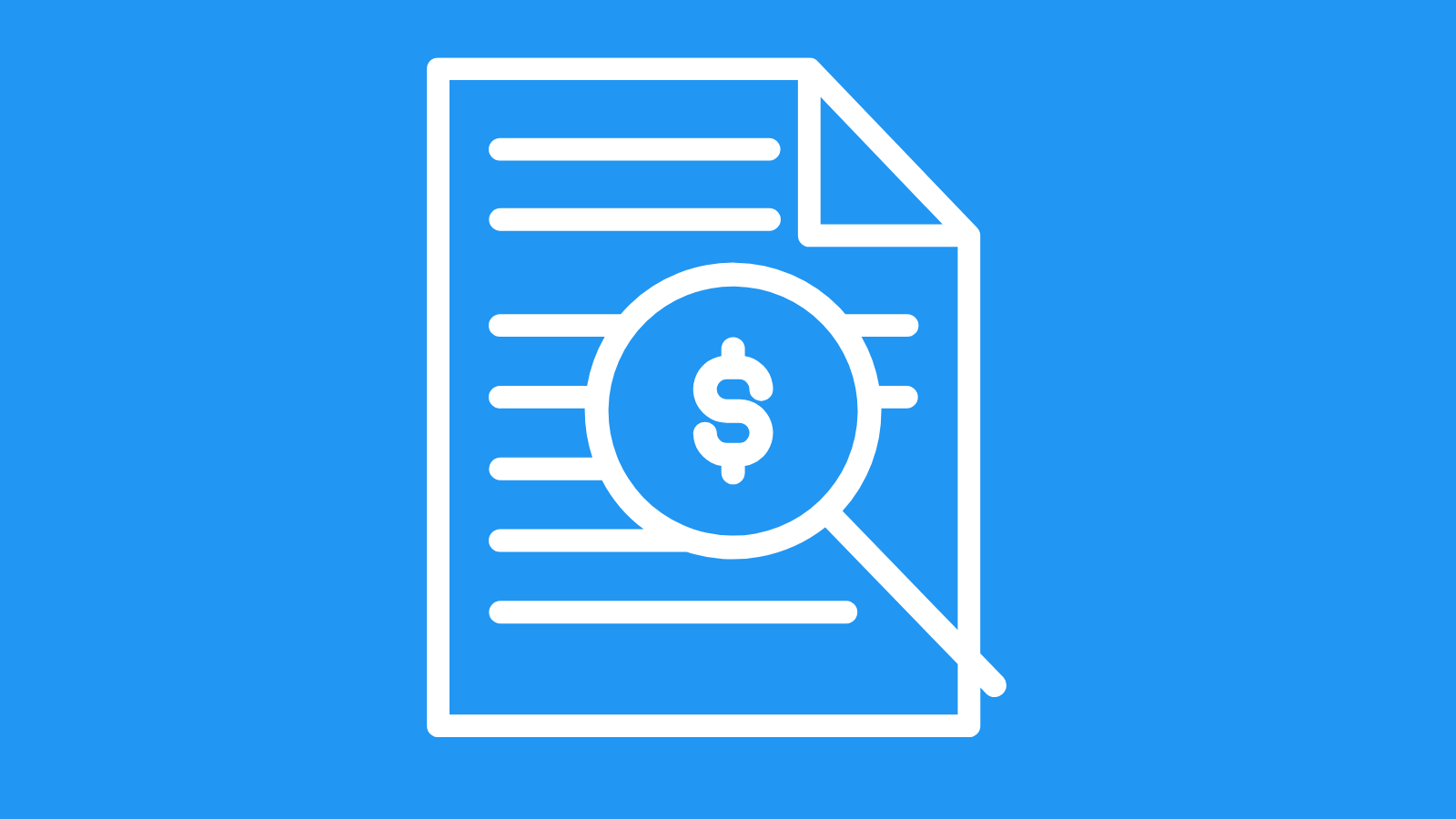
XPOF Stock Price Forecasting Model
This model for Xponential Fitness Inc. Class A Common Stock (XPOF) utilizes a hybrid machine learning approach combining time series analysis and fundamental data. We employ a recurrent neural network (RNN), specifically a long short-term memory (LSTM) network, to capture the complex and often non-linear relationships within the historical stock price data. Crucially, the model integrates financial indicators derived from fundamental analysis. These indicators, such as earnings per share (EPS) growth, revenue trends, and market capitalization, are standardized and normalized to ensure they contribute meaningfully to the prediction process. Feature engineering plays a vital role in enhancing model performance. We construct various technical indicators, including moving averages, volatility measures, and volume data, to incorporate the short-term market dynamics and potential investor sentiment fluctuations. Careful feature selection and engineering are essential to improve predictive accuracy. A validation set is used to monitor the model's performance and avoid overfitting to the training data.
The LSTM model's architecture is optimized to handle sequential data and its long-term dependencies. It is trained on a comprehensive dataset containing historical XPOF stock price information and associated fundamental and technical indicators. The model is trained to predict future stock price movements, considering the internal relationships and fluctuations observed in the past data. Parameter tuning is critical to the model's success, and we employ techniques such as backpropagation through time and gradient descent to refine the model's weights and biases. Regularization techniques are also employed to prevent overfitting to the training data. This refined model, after rigorous testing and optimization, is expected to provide reasonable stock price predictions. The model output will not be a precise prediction, but a probability distribution, allowing investors to understand the potential for different stock price outcomes. A critical component of this process is a robust risk assessment strategy, as no predictive model is guaranteed to provide accurate results in all scenarios.
Crucially, this model is designed to be continuously updated and refined. Regular monitoring and retraining of the model on new data will maintain its accuracy and adaptability to shifting market conditions. Ongoing review and evaluation of performance metrics, such as mean squared error (MSE) and root mean squared error (RMSE), will be conducted. This iterative approach allows for adjustments and improvements to the model's architecture and the input data characteristics as new information becomes available. Incorporating external factors, such as macroeconomic conditions and industry trends, will be examined to further enhance the predictive power of the model. This model's output will be part of a broader investment strategy, and its output should not be viewed in isolation. Consult with a qualified financial advisor before making any investment decisions.
ML Model Testing
n:Time series to forecast
p:Price signals of Xponential Fitness stock
j:Nash equilibria (Neural Network)
k:Dominated move of Xponential Fitness stock holders
a:Best response for Xponential Fitness target price
For further technical information as per how our model work we invite you to visit the article below:
How do KappaSignal algorithms actually work?
Xponential Fitness Stock Forecast (Buy or Sell) Strategic Interaction Table
Strategic Interaction Table Legend:
X axis: *Likelihood% (The higher the percentage value, the more likely the event will occur.)
Y axis: *Potential Impact% (The higher the percentage value, the more likely the price will deviate.)
Z axis (Grey to Black): *Technical Analysis%
Xponential Fitness Inc. Financial Outlook and Forecast
Xponential Fitness's (XPO) financial outlook presents a complex picture, characterized by a growth trajectory intertwined with substantial challenges. The company's business model, built around a network of franchised fitness studios, relies heavily on the overall health and fitness market, which is susceptible to economic downturns and shifts in consumer preferences. While the fitness industry remains robust, increasing competition from established players and emerging digital fitness platforms creates a highly competitive landscape. A key aspect of XPO's strategy centers on expanding its studio footprint and optimizing franchisee profitability, but this hinges on maintaining brand appeal and effectively addressing operational challenges, such as consistent quality control across diverse locations. Revenue projections, driven by studio growth, are crucial to evaluating the long-term success of the company's expansion strategy. Sustained growth in membership, coupled with effective management of franchisee relations and studio operational costs, is paramount for achieving positive financial results.
Analyzing historical financial performance reveals fluctuations in profitability and revenue growth. XPO's past results have often mirrored trends in the fitness industry, experiencing periods of robust expansion followed by periods of greater challenges. Key indicators like subscriber acquisition costs, studio operating margins, and overall profitability will significantly shape the company's future financial prospects. Analyzing the performance of franchisee operations and evaluating the overall health of the fitness industry are critical for anticipating the impact of economic conditions and competitive pressures. The company's ability to adapt to evolving consumer demands and competitive pressures will be a critical factor. A comprehensive and detailed financial model should incorporate various macroeconomic variables, market trends, and competitive pressures to predict future performance. Management's competence in executing its strategy and the overall industry climate will directly influence the company's future financial performance.
Critical financial metrics include revenue growth, operating margins, and franchisee profitability. XPO's ability to attract and retain members, particularly in the face of rising costs and increasing competition, will be crucial to revenue growth. Improving operating margins through efficient studio management and controlling costs is essential for sustainable profitability. Moreover, strengthening franchisee relationships and supporting their success is vital for long-term growth. Strong franchisee earnings and positive franchisee feedback directly correlate with the company's success and investor confidence. The company's ability to innovate and introduce new fitness programming and studio concepts will play a crucial role in attracting and retaining members. This includes the effectiveness of marketing campaigns, the quality of customer service, and the overall appeal of the company's brand and studio offerings.
Prediction: A moderate positive outlook exists for XPO, but with considerable risk. The growth trajectory within the fitness industry presents both opportunities and significant challenges. Success hinges on the company's ability to effectively manage franchisee operations, adapt to changing consumer preferences, and control operating costs. Increased competition and economic uncertainty pose risks. The company's ability to adapt to the changing market and maintain a consistent brand image across multiple locations is paramount. A potential downside risk includes unforeseen economic downturns that could decrease consumer spending on fitness services. Furthermore, unforeseen technological advancements in the fitness industry could disrupt XPO's business model. Positive indicators include industry growth and the expansion potential within the existing franchise network. However, these must be carefully weighed against the risks of economic downturns, evolving preferences, and intense competition within the fitness industry. Successful execution of the company's strategy and effective management will be crucial factors in achieving a positive outcome.
Rating | Short-Term | Long-Term Senior |
---|---|---|
Outlook | Ba3 | Ba1 |
Income Statement | Ba3 | Baa2 |
Balance Sheet | B2 | B2 |
Leverage Ratios | Ba1 | Baa2 |
Cash Flow | B2 | Caa2 |
Rates of Return and Profitability | Ba2 | Baa2 |
*Financial analysis is the process of evaluating a company's financial performance and position by neural network. It involves reviewing the company's financial statements, including the balance sheet, income statement, and cash flow statement, as well as other financial reports and documents.
How does neural network examine financial reports and understand financial state of the company?
References
- Rumelhart DE, Hinton GE, Williams RJ. 1986. Learning representations by back-propagating errors. Nature 323:533–36
- S. Bhatnagar, H. Prasad, and L. Prashanth. Stochastic recursive algorithms for optimization, volume 434. Springer, 2013
- Abadie A, Cattaneo MD. 2018. Econometric methods for program evaluation. Annu. Rev. Econ. 10:465–503
- Chow, G. C. (1960), "Tests of equality between sets of coefficients in two linear regressions," Econometrica, 28, 591–605.
- Hastie T, Tibshirani R, Tibshirani RJ. 2017. Extended comparisons of best subset selection, forward stepwise selection, and the lasso. arXiv:1707.08692 [stat.ME]
- M. J. Hausknecht and P. Stone. Deep recurrent Q-learning for partially observable MDPs. CoRR, abs/1507.06527, 2015
- Zubizarreta JR. 2015. Stable weights that balance covariates for estimation with incomplete outcome data. J. Am. Stat. Assoc. 110:910–22