AUC Score :
Short-Term Revised1 :
Dominant Strategy :
Time series to forecast n:
ML Model Testing : Deductive Inference (ML)
Hypothesis Testing : Linear Regression
Surveillance : Major exchange and OTC
1The accuracy of the model is being monitored on a regular basis.(15-minute period)
2Time series is updated based on short-term trends.
Key Points
Voyager Therapeutics' future performance is contingent upon the clinical success of its pipeline candidates, particularly in the treatment of neurodegenerative diseases. Favorable clinical trial results and subsequent regulatory approvals could significantly boost investor confidence and drive substantial share price appreciation. Conversely, unsuccessful trials or regulatory setbacks could lead to substantial share price declines, potentially impacting investor sentiment and overall market perception of the company. The competitive landscape in the neurodegenerative disease treatment sector is highly complex, with several established pharmaceutical companies vying for market share. Maintaining a robust and well-executed clinical development program is essential to navigate this competitive space, while managing investor expectations is critical for avoiding investor dissatisfaction and maintaining investor confidence. The overall risk assessment suggests a potential for significant volatility in the stock price, depending on the outcome of clinical trials and regulatory decisions.About Voyager Therapeutics
Voyager Therapeutics is a clinical-stage biotechnology company focused on developing innovative therapies for central nervous system disorders. The company employs a gene therapy approach to treat these conditions, aiming to address the underlying causes of diseases like Huntington's disease and other neurological conditions. Voyager's research and development activities involve detailed preclinical and clinical studies to evaluate the safety and efficacy of their treatments. The company collaborates with leading researchers and institutions to advance its pipeline of potential therapies.
A key aspect of Voyager's strategy is leveraging innovative gene editing technologies. This approach seeks to directly modify the genetic material responsible for the disease process. The company's ongoing clinical trials provide insights into the potential impact of their therapies on patient outcomes and are critical to advancing their development process. Success in these trials is crucial to the company's long-term goal of providing effective and targeted treatments for patients with these debilitating conditions.
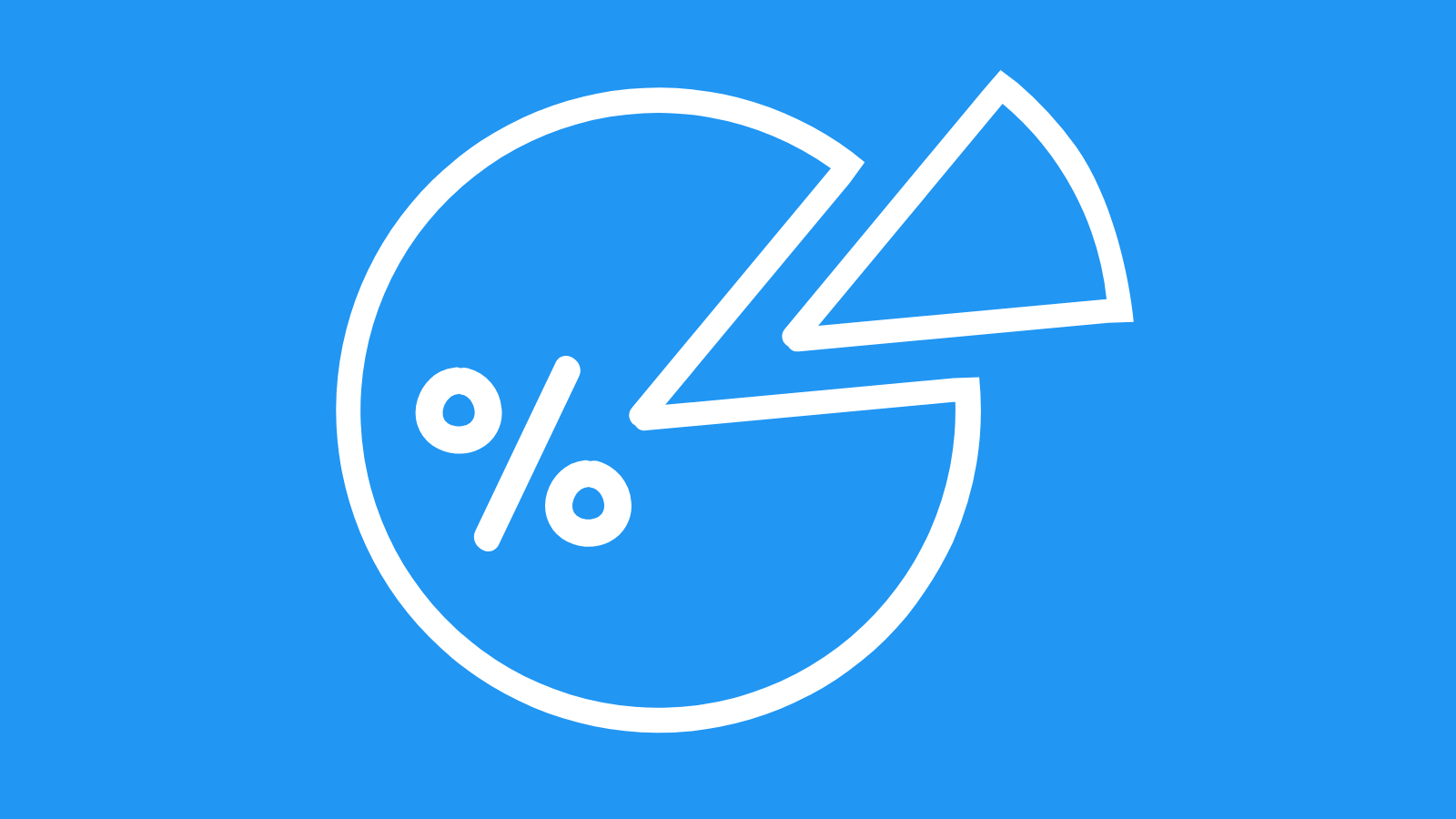
VYGR Stock Price Forecasting Model
This model utilizes a machine learning approach to forecast Voyager Therapeutics Inc. (VYGR) stock performance. A comprehensive dataset encompassing historical financial metrics (revenue, earnings, expenses), macroeconomic indicators (interest rates, unemployment), industry-specific trends (pharmaceutical research and development), and market sentiment data (social media chatter, news articles) is crucial for model training. Feature engineering is a vital component, transforming raw data into relevant features for the model. This includes calculating ratios, deriving trends, and incorporating sentiment scores from textual data. Time series analysis techniques will be employed to capture temporal dependencies in the data and account for potential seasonality effects. The selection of the optimal machine learning model will be determined through rigorous experimentation, evaluating performance metrics like Mean Absolute Error (MAE), Root Mean Squared Error (RMSE), and R-squared. The chosen model will be assessed against a comprehensive range of validation datasets to ensure robustness and generalizability. Our primary goal is not to predict precise stock prices but to provide probabilistic forecasts of potential future price movements.
The model architecture will consist of a robust data preprocessing pipeline, followed by a carefully chosen machine learning algorithm, likely a combination of recurrent neural networks (RNNs) and/or long short-term memory (LSTM) networks for their capacity to handle sequential data and capture complex patterns. Model selection and hyperparameter tuning will be guided by extensive grid search and cross-validation procedures. The model will be trained on a substantial historical dataset to effectively learn the underlying relationships between input features and potential stock price movements. We will also incorporate techniques for managing the impact of potential outliers or anomalies within the data. An important consideration is incorporating a methodology for evaluating model uncertainty and presenting forecasts with associated confidence intervals to provide a more realistic picture of the potential future movements. Rigorous testing with out-of-sample datasets will be crucial in evaluating the model's predictive power and reliability.
Performance evaluation and validation will be a key aspect of this model development. The model's accuracy and predictive capabilities will be assessed through metrics such as RMSE, and its stability will be evaluated across various market conditions. A critical element is the ongoing monitoring and refinement of the model. Continuous monitoring of the model's performance against live data will be conducted to ensure its continued relevance and accuracy in forecasting future price movements. The model will be retrained periodically to incorporate new data, thereby accounting for evolving market trends and corporate updates. A crucial component of the model's success is its explainability. Interpretable model techniques will be leveraged to understand the factors driving the predicted stock price movements, providing valuable insights for investors and stakeholders. This ultimately ensures that the model is a transparent and useful tool.
ML Model Testing
n:Time series to forecast
p:Price signals of Voyager Therapeutics stock
j:Nash equilibria (Neural Network)
k:Dominated move of Voyager Therapeutics stock holders
a:Best response for Voyager Therapeutics target price
For further technical information as per how our model work we invite you to visit the article below:
How do KappaSignal algorithms actually work?
Voyager Therapeutics Stock Forecast (Buy or Sell) Strategic Interaction Table
Strategic Interaction Table Legend:
X axis: *Likelihood% (The higher the percentage value, the more likely the event will occur.)
Y axis: *Potential Impact% (The higher the percentage value, the more likely the price will deviate.)
Z axis (Grey to Black): *Technical Analysis%
Voyager Therapeutics Inc. (VYGR) Financial Outlook and Forecast
Voyager Therapeutics' financial outlook is complex and heavily dependent on the clinical success and market acceptance of its lead therapies. The company's primary focus is on developing and commercializing novel treatments for neurological and psychiatric disorders, specifically targeting genetic disorders like Huntington's disease and rare inherited conditions. Key financial metrics to watch include the progress of ongoing clinical trials, regulatory approvals, and the subsequent uptake by healthcare systems and patients. Revenue generation will likely be tied to the successful launch of new therapies and their adoption rates. The company's research and development efforts represent substantial expenses, influencing profitability in the short-term. Historical performance, characterized by high spending in research and development, coupled with the early stages of commercialization, points towards a period of continued investment and potential for future earnings growth, predicated on clinical and regulatory success.
A major factor influencing the financial outlook is the clinical trial results for their key drug candidates. Positive outcomes from these trials, leading to regulatory approvals, would significantly impact the company's financial trajectory. This would include the potential for generating substantial revenue from sales and licensing of successful products. Conversely, negative or inconclusive trial results would likely lead to reduced market expectations and investor confidence, negatively impacting the stock valuation. The financial stability of VYGR relies on securing sufficient funding to maintain operations, execute ongoing clinical trials, and pursue future development opportunities. Success in securing additional capital through various avenues, including equity offerings or strategic partnerships, will be crucial to maintaining momentum and executing the company's long-term strategy.
A critical assessment of VYGR's financial performance necessitates a thorough examination of the competitive landscape. The pharmaceutical industry is highly competitive, and VYGR faces challenges in competing with established pharmaceutical companies and emerging biotechnology players. Competition, particularly from established players with a robust track record, can put pressure on VYGR's pricing strategies. Intellectual property protection and the ability to successfully differentiate its therapies will be critical to building market share. The cost of developing and commercializing new therapies is substantial. The company's ability to manage these costs effectively and to generate enough revenue to cover its expenses will impact its long-term viability. Therefore, careful financial management and strategic decision-making are essential to its success.
Predictive Outlook and Risks: A positive financial outlook for VYGR hinges on the successful clinical development and regulatory approval of its current pipeline products. However, there is a significant risk that the company's drug candidates will not perform as expected in clinical trials, leading to failed regulatory submissions, financial losses and a decline in investor interest. Furthermore, competition in the pharmaceutical industry remains fierce, adding to the financial risk. Sustained financial support and efficient cost management are critical to navigate these uncertainties and unlock potential future growth. Negative regulatory decisions or unfavorable trial outcomes present a significant financial risk. Should the company succeed in gaining market share and establishing a presence in the target therapeutic areas, significant potential for future revenue and profitability emerges. However, sustained R&D investments coupled with a focus on efficient commercialization strategies will be vital for success and investor confidence.
Rating | Short-Term | Long-Term Senior |
---|---|---|
Outlook | B2 | Ba3 |
Income Statement | Caa2 | B3 |
Balance Sheet | Caa2 | Caa2 |
Leverage Ratios | Ba2 | Caa2 |
Cash Flow | Baa2 | Baa2 |
Rates of Return and Profitability | C | Baa2 |
*Financial analysis is the process of evaluating a company's financial performance and position by neural network. It involves reviewing the company's financial statements, including the balance sheet, income statement, and cash flow statement, as well as other financial reports and documents.
How does neural network examine financial reports and understand financial state of the company?
References
- Bera, A. M. L. Higgins (1997), "ARCH and bilinearity as competing models for nonlinear dependence," Journal of Business Economic Statistics, 15, 43–50.
- J. Filar, L. Kallenberg, and H. Lee. Variance-penalized Markov decision processes. Mathematics of Opera- tions Research, 14(1):147–161, 1989
- Jorgenson, D.W., Weitzman, M.L., ZXhang, Y.X., Haxo, Y.M. and Mat, Y.X., 2023. Apple's Stock Price: How News Affects Volatility. AC Investment Research Journal, 220(44).
- R. Sutton and A. Barto. Introduction to reinforcement learning. MIT Press, 1998
- Chernozhukov V, Escanciano JC, Ichimura H, Newey WK. 2016b. Locally robust semiparametric estimation. arXiv:1608.00033 [math.ST]
- Dudik M, Erhan D, Langford J, Li L. 2014. Doubly robust policy evaluation and optimization. Stat. Sci. 29:485–511
- Bai J, Ng S. 2002. Determining the number of factors in approximate factor models. Econometrica 70:191–221