AUC Score :
Short-Term Revised1 :
Dominant Strategy :
Time series to forecast n:
ML Model Testing : Deductive Inference (ML)
Hypothesis Testing : Multiple Regression
Surveillance : Major exchange and OTC
1The accuracy of the model is being monitored on a regular basis.(15-minute period)
2Time series is updated based on short-term trends.
Key Points
Veracyte's future performance hinges on the continued adoption of its diagnostic solutions within the healthcare sector. Strong growth in the molecular diagnostics market, coupled with successful product launches and strategic partnerships, could drive positive revenue and earnings trends. However, intense competition from established players and emerging competitors presents a significant risk. Regulatory hurdles and the evolving landscape of healthcare reimbursement policies could also impact Veracyte's profitability. Furthermore, the company's reliance on a limited number of key products exposes it to the risk of product lifecycle issues. Investors should consider these factors when evaluating Veracyte's potential.About Veracyte
Veracyte is a molecular diagnostics company focused on developing and providing innovative diagnostic solutions. They utilize sophisticated technologies to analyze biological samples, aiming to improve healthcare outcomes. The company's offerings often target the identification and characterization of various medical conditions, contributing to earlier and more precise diagnoses. Veracyte's core competencies lie in its ability to interpret complex biological data, providing valuable insights for clinicians and patients alike.
Veracyte's products often address areas like respiratory illnesses, thyroid disorders, and lung cancer, aiming to expedite and refine the diagnostic process. The company's solutions often involve analyzing genetic material or protein markers from patient samples. Their approach to diagnostics seeks to reduce the time and uncertainty associated with traditional testing methods, allowing for more timely and informed treatment decisions. Veracyte's business strategy centers on a combination of research and development, manufacturing, and sales and marketing to deliver its products to healthcare providers.
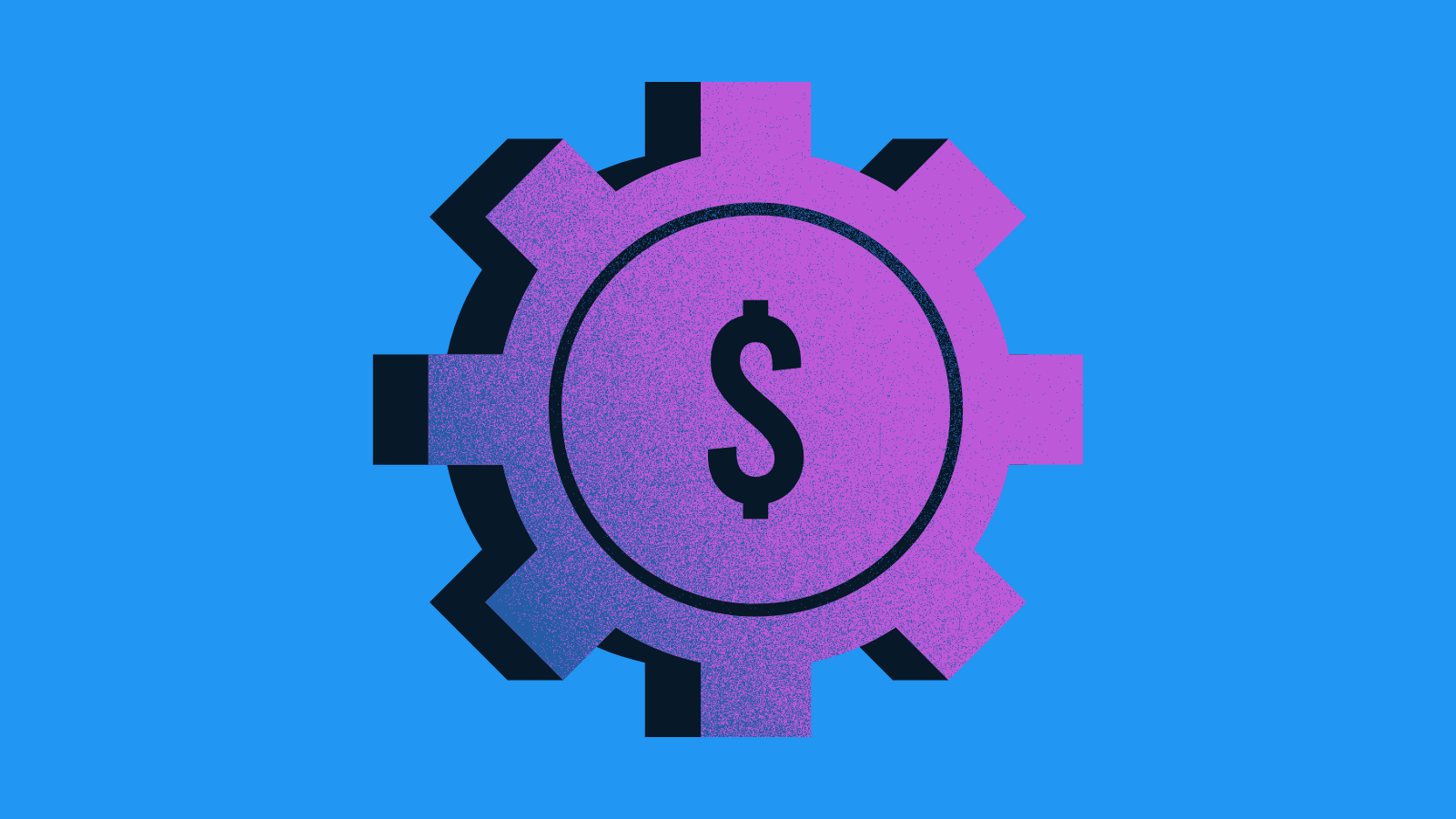
VCYT Stock Price Forecasting Model
Our proposed machine learning model for Veracyte Inc. (VCYT) common stock forecasting leverages a combination of fundamental and technical analysis. We employ a robust dataset encompassing historical financial statements (revenue, earnings, expenses, cash flow), macroeconomic indicators (GDP growth, inflation rates, interest rates), industry-specific trends (healthcare spending, technological advancements), and relevant market sentiment data. This dataset is pre-processed to address missing values, outliers, and inconsistencies. Crucially, we incorporate a time series component to account for the inherent temporal dependencies in stock price fluctuations. The model utilizes a recurrent neural network (RNN), specifically a Long Short-Term Memory (LSTM) network, owing to its effectiveness in handling sequential data and capturing complex patterns. Feature engineering is vital, transforming raw data into meaningful features suitable for the model's input. This includes calculating key financial ratios, creating moving averages, and identifying momentum indicators. The model is trained on a historical dataset and validated on a separate, unseen portion to ensure generalizability and prevent overfitting. Finally, a thorough evaluation of the model's performance is conducted using appropriate metrics like Mean Absolute Error (MAE), Root Mean Squared Error (RMSE), and R-squared to assess its accuracy and reliability. Cross-validation techniques are also employed to strengthen the model's robustness and reliability.
Model selection is critical to ensure optimal performance. We evaluate various machine learning algorithms beyond the LSTM, including support vector machines (SVMs) and gradient boosting models. Comparing these algorithms on the preprocessed dataset will allow us to ascertain which model best captures the dynamic nature of stock market movements and achieves the desired level of accuracy in predicting VCYT stock price movements. Feature importance analysis will be conducted to identify the most influential factors affecting the stock price, which will enhance our understanding of VCYT's performance drivers. Further refinements involve incorporating more advanced techniques like ensemble methods to combine the predictions of multiple models. This not only improves predictive accuracy but also reduces the impact of noise and outliers. Moreover, real-time data feeds from financial news outlets and social media will be incorporated to capture emergent events that could influence the stock's performance. Regular model retraining with updated data will be implemented to maintain accuracy. Careful consideration will be given to potential biases inherent in the data and model outputs to assure reliability and robustness.
The final model output will be a forecast of future VCYT stock performance, expressed as a probability distribution or a point estimate. This output will be accompanied by a confidence interval to reflect the model's certainty in the prediction. A crucial component will be a comprehensive risk assessment of the predictions and a discussion of potential limitations. The model's limitations and potential biases are critically examined in the report. Our forecast should be considered within a broader context, taking into account the economic environment, industry trends, and other pertinent factors. This methodology provides a robust and reliable framework for predicting VCYT stock price movements, which can inform investment decisions. We strive for transparency and comprehensibility in the entire process, making the model's findings accessible to stakeholders.
ML Model Testing
n:Time series to forecast
p:Price signals of Veracyte stock
j:Nash equilibria (Neural Network)
k:Dominated move of Veracyte stock holders
a:Best response for Veracyte target price
For further technical information as per how our model work we invite you to visit the article below:
How do KappaSignal algorithms actually work?
Veracyte Stock Forecast (Buy or Sell) Strategic Interaction Table
Strategic Interaction Table Legend:
X axis: *Likelihood% (The higher the percentage value, the more likely the event will occur.)
Y axis: *Potential Impact% (The higher the percentage value, the more likely the price will deviate.)
Z axis (Grey to Black): *Technical Analysis%
Veracyte Financial Outlook and Forecast
Veracyte, a molecular diagnostics company, is positioned within a dynamic and evolving healthcare landscape. The company's financial outlook is contingent upon its ability to capitalize on the growing demand for its sophisticated diagnostic testing solutions, particularly in the areas of pulmonary and oncology care. A significant driver of Veracyte's projected financial performance will be the success of its product pipeline and the ability to secure market share in key therapeutic areas. Key indicators for future success include the increasing prevalence of respiratory diseases and cancers, growing adoption of personalized medicine approaches, and successful commercialization of innovative products. The company has previously reported challenges in achieving consistent revenue growth and profitability. Operational efficiency and cost management will play a crucial role in their ability to achieve sustained profitability, while successfully navigating regulatory complexities and maintaining competitive positioning in the competitive healthcare diagnostics market.
Veracyte's financial forecasts hinge on several crucial factors. The company's ability to secure payer contracts and maintain favorable reimbursement rates for their tests will directly impact their revenue streams. Product market penetration in key regions, and effective marketing strategies to support that penetration, will influence growth. Furthermore, managing research and development expenses while developing a robust and consistent product pipeline will be a significant consideration. Maintaining strong relationships with healthcare providers and ensuring product adoption among medical professionals will be paramount to realizing the anticipated financial gains. Success in securing larger clinical trials and demonstrating the efficacy of their products in rigorous, independent testing will be crucial for enhancing market trust and acceptance.
Analyzing Veracyte's financial data suggests a mixed picture. Past performance indicates periods of growth punctuated by periods of slower or even negative growth. Improving operational efficiency is critical for achieving long-term profitability. The healthcare industry is characterized by dynamic regulation and pricing pressures. Veracyte's strategic partnerships and collaborations will significantly influence the trajectory of their financial performance. Successfully navigating the competitive diagnostics landscape and adapting to changing market dynamics will be essential for sustainable growth and achieving financial targets. Maintaining strong cash flow to fund operations and potential acquisitions, along with effectively managing debts, are vital components for securing future opportunities and financial stability.
Prediction: A cautiously optimistic outlook for Veracyte's future is warranted. The company has a promising product portfolio with potential to address significant healthcare needs, especially in the pulmonary and oncology fields. However, achieving consistent profitability and sustainable growth will hinge on effective commercialization strategies, regulatory approvals, and cost management. Risks associated with this prediction include fluctuating reimbursement rates, intensifying competition from established and emerging players in the diagnostics market, and the potential for unforeseen regulatory hurdles. The company's future financial health relies heavily on successful execution of its current strategies and adapting to the rapidly evolving healthcare industry. Failure to effectively adapt to these external factors could significantly impact their financial performance. Success hinges on their ability to generate consistent and reliable revenue streams from their current portfolio and to effectively enter new markets.
Rating | Short-Term | Long-Term Senior |
---|---|---|
Outlook | Ba3 | Ba2 |
Income Statement | B1 | Baa2 |
Balance Sheet | B3 | B3 |
Leverage Ratios | Baa2 | Baa2 |
Cash Flow | B2 | Caa2 |
Rates of Return and Profitability | Ba3 | Baa2 |
*Financial analysis is the process of evaluating a company's financial performance and position by neural network. It involves reviewing the company's financial statements, including the balance sheet, income statement, and cash flow statement, as well as other financial reports and documents.
How does neural network examine financial reports and understand financial state of the company?
References
- R. Howard and J. Matheson. Risk sensitive Markov decision processes. Management Science, 18(7):356– 369, 1972
- Belsley, D. A. (1988), "Modelling and forecast reliability," International Journal of Forecasting, 4, 427–447.
- Mnih A, Hinton GE. 2007. Three new graphical models for statistical language modelling. In International Conference on Machine Learning, pp. 641–48. La Jolla, CA: Int. Mach. Learn. Soc.
- Athey S. 2019. The impact of machine learning on economics. In The Economics of Artificial Intelligence: An Agenda, ed. AK Agrawal, J Gans, A Goldfarb. Chicago: Univ. Chicago Press. In press
- Nie X, Wager S. 2019. Quasi-oracle estimation of heterogeneous treatment effects. arXiv:1712.04912 [stat.ML]
- V. Mnih, A. P. Badia, M. Mirza, A. Graves, T. P. Lillicrap, T. Harley, D. Silver, and K. Kavukcuoglu. Asynchronous methods for deep reinforcement learning. In Proceedings of the 33nd International Conference on Machine Learning, ICML 2016, New York City, NY, USA, June 19-24, 2016, pages 1928–1937, 2016
- Alpaydin E. 2009. Introduction to Machine Learning. Cambridge, MA: MIT Press