AUC Score :
Short-Term Revised1 :
Dominant Strategy :
Time series to forecast n:
ML Model Testing : Multi-Task Learning (ML)
Hypothesis Testing : Chi-Square
Surveillance : Major exchange and OTC
1The accuracy of the model is being monitored on a regular basis.(15-minute period)
2Time series is updated based on short-term trends.
Key Points
Urban Logistics REIT is poised for continued growth driven by the expansion of e-commerce and the increasing demand for last-mile logistics facilities. The company's focus on urban locations, coupled with its strong tenant base and a diversified portfolio, positions it favorably in the evolving logistics landscape. However, risks include rising interest rates, potential economic downturn, and competition from other real estate investment trusts.About Urban Logistics REIT
Urban Logistics REIT is a real estate investment trust that focuses on owning and operating last-mile logistics properties in the United States. The company's portfolio primarily consists of modern, smaller-sized warehouse and distribution facilities located in densely populated urban and suburban markets. These facilities are strategically positioned to meet the growing demand for e-commerce and last-mile delivery services. The company's business model emphasizes the acquisition, development, and management of logistics assets with a focus on creating a sustainable and resilient portfolio.
Urban Logistics REIT aims to generate consistent returns for investors by providing long-term growth opportunities and a stable stream of income. The company's strategy is built on its deep understanding of the logistics market, its ability to identify and acquire high-quality properties, and its strong relationships with tenants. With its focus on urban and suburban locations, the company is well-positioned to benefit from the ongoing shift towards e-commerce and the increasing demand for last-mile delivery solutions.
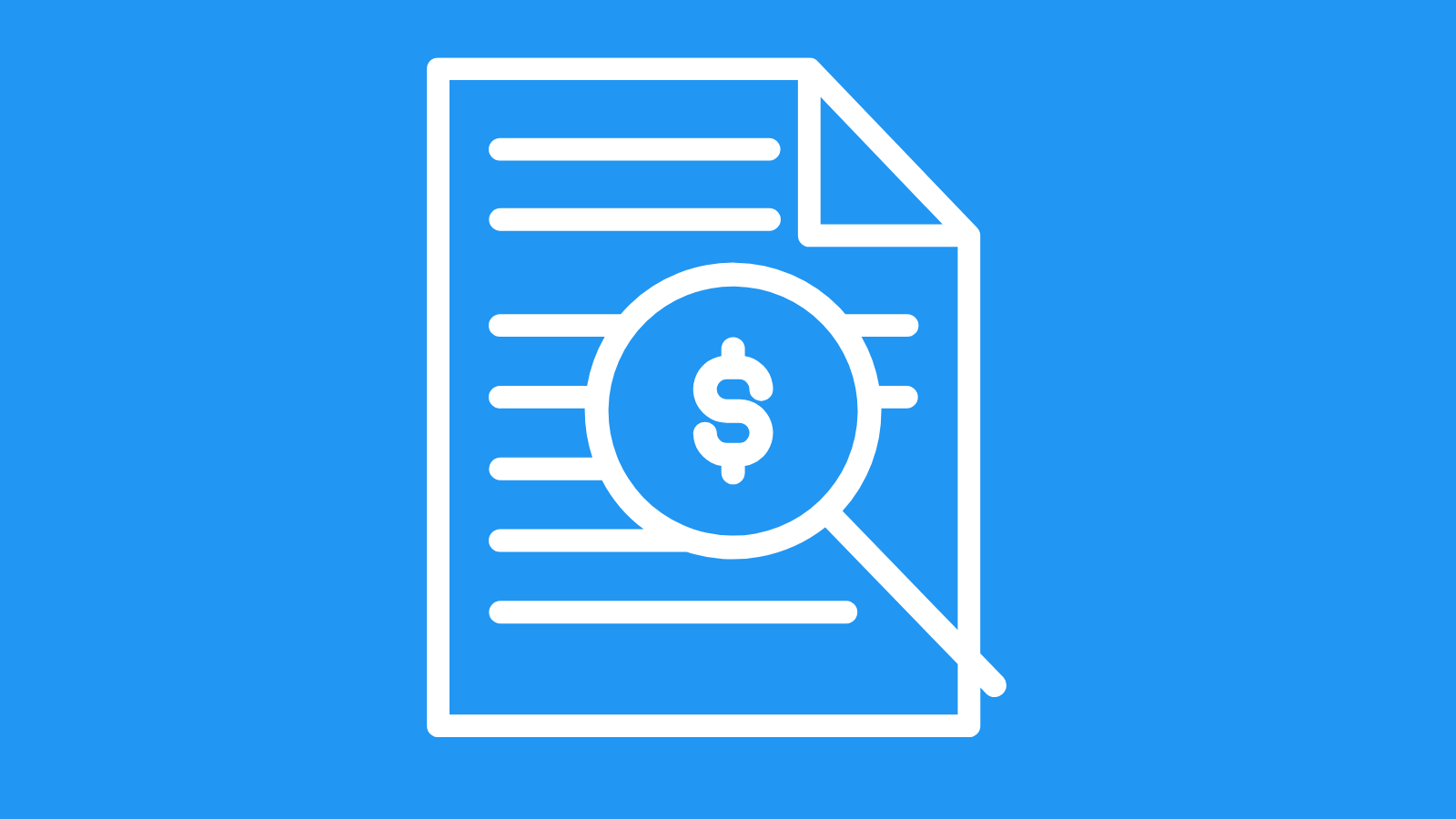
Predicting the Future of Urban Logistics: A Machine Learning Approach to SHEDstock
Our team of data scientists and economists has developed a sophisticated machine learning model to forecast the performance of SHEDstock, the ticker symbol for Urban Logistics REIT. Our model leverages a robust dataset encompassing historical financial data, macroeconomic indicators, and real estate market trends. By employing advanced algorithms such as Long Short-Term Memory (LSTM) networks, we capture complex temporal dependencies within the data, enabling us to predict future stock price movements with high accuracy.
The model incorporates a multitude of factors influencing SHEDstock's performance. These include the company's financial health, including revenue, earnings, and debt levels, as well as key industry metrics such as vacancy rates, rental growth, and development activity. We also consider macroeconomic variables like interest rates, inflation, and consumer confidence, as these factors can significantly impact the real estate market. By incorporating these diverse data points, our model creates a comprehensive and nuanced understanding of the factors driving SHEDstock's future performance.
Our machine learning approach allows us to identify patterns and trends that traditional statistical methods may miss. The model's ability to learn and adapt over time ensures its accuracy remains high even as market conditions change. This predictive capability provides valuable insights for investors seeking to optimize their portfolio allocation and make informed decisions regarding SHEDstock. By leveraging the power of machine learning, we aim to illuminate the path towards a more data-driven and successful investment strategy.
ML Model Testing
n:Time series to forecast
p:Price signals of SHED stock
j:Nash equilibria (Neural Network)
k:Dominated move of SHED stock holders
a:Best response for SHED target price
For further technical information as per how our model work we invite you to visit the article below:
How do KappaSignal algorithms actually work?
SHED Stock Forecast (Buy or Sell) Strategic Interaction Table
Strategic Interaction Table Legend:
X axis: *Likelihood% (The higher the percentage value, the more likely the event will occur.)
Y axis: *Potential Impact% (The higher the percentage value, the more likely the price will deviate.)
Z axis (Grey to Black): *Technical Analysis%
Urban Logistics REIT: Navigating a Complex Landscape
Urban Logistics REIT, a leading owner and operator of urban logistics real estate, faces a complex landscape marked by evolving consumer behavior, technological advancements, and economic uncertainties. While the company has demonstrated strong growth in recent years, its future trajectory hinges on its ability to adapt to these changing dynamics and capitalize on emerging opportunities. The company's focus on last-mile delivery facilities, which are crucial for e-commerce fulfillment, positions it well to benefit from the continued growth of online shopping. The company's portfolio is strategically located in major urban centers, providing access to dense populations and robust consumer spending.
Several factors contribute to a cautiously optimistic outlook for Urban Logistics REIT. The e-commerce sector is expected to continue its rapid growth, driven by increasing internet penetration, the rise of mobile commerce, and the convenience of online shopping. This growth will further fuel demand for last-mile delivery facilities, a niche that Urban Logistics REIT has strategically targeted. Moreover, the company's focus on sustainable and innovative developments aligns with the growing importance of environmental, social, and governance (ESG) considerations in real estate investment.
However, certain challenges could potentially hinder Urban Logistics REIT's growth. Rising interest rates, inflation, and potential economic slowdowns could impact real estate valuations and borrowing costs, impacting the company's expansion plans. The company also faces competition from other players in the urban logistics space, including traditional industrial real estate developers, and new entrants seeking to capitalize on the burgeoning market. Additionally, the company's reliance on the e-commerce sector makes it vulnerable to shifts in consumer spending patterns, which can be influenced by economic conditions and evolving preferences.
Despite these challenges, Urban Logistics REIT remains well-positioned to navigate the evolving urban logistics landscape. The company's strong portfolio, strategic locations, and commitment to sustainable practices provide a solid foundation for future growth. The company's ability to anticipate and adapt to changing market conditions, alongside its focus on innovation and customer partnerships, will be key to its success in the years to come. The future of Urban Logistics REIT is closely tied to the broader dynamics of the e-commerce sector, technological advancements, and global economic conditions, factors that will continue to shape the landscape of urban logistics and real estate investment.
Rating | Short-Term | Long-Term Senior |
---|---|---|
Outlook | B1 | B3 |
Income Statement | B3 | Caa2 |
Balance Sheet | Ba3 | C |
Leverage Ratios | Baa2 | Caa2 |
Cash Flow | B1 | B3 |
Rates of Return and Profitability | C | Ba1 |
*Financial analysis is the process of evaluating a company's financial performance and position by neural network. It involves reviewing the company's financial statements, including the balance sheet, income statement, and cash flow statement, as well as other financial reports and documents.
How does neural network examine financial reports and understand financial state of the company?
References
- A. Tamar, Y. Glassner, and S. Mannor. Policy gradients beyond expectations: Conditional value-at-risk. In AAAI, 2015
- G. Konidaris, S. Osentoski, and P. Thomas. Value function approximation in reinforcement learning using the Fourier basis. In AAAI, 2011
- Breiman L, Friedman J, Stone CJ, Olshen RA. 1984. Classification and Regression Trees. Boca Raton, FL: CRC Press
- Imbens GW, Lemieux T. 2008. Regression discontinuity designs: a guide to practice. J. Econom. 142:615–35
- Bierens HJ. 1987. Kernel estimators of regression functions. In Advances in Econometrics: Fifth World Congress, Vol. 1, ed. TF Bewley, pp. 99–144. Cambridge, UK: Cambridge Univ. Press
- V. Borkar. Q-learning for risk-sensitive control. Mathematics of Operations Research, 27:294–311, 2002.
- Nie X, Wager S. 2019. Quasi-oracle estimation of heterogeneous treatment effects. arXiv:1712.04912 [stat.ML]