AUC Score :
Short-Term Revised1 :
Dominant Strategy :
Time series to forecast n:
ML Model Testing : Deductive Inference (ML)
Hypothesis Testing : Pearson Correlation
Surveillance : Major exchange and OTC
1The accuracy of the model is being monitored on a regular basis.(15-minute period)
2Time series is updated based on short-term trends.
Key Points
Upbound's future performance is contingent upon several factors. Continued strong growth in the cloud-native infrastructure management sector presents a positive outlook. However, intense competition in this market poses a significant risk. Successfully navigating these competitive pressures and maintaining profitability while scaling operations will be crucial for Upbound's success. Potential fluctuations in customer demand and the adoption rates of cloud-native technologies could negatively impact revenue streams. Maintaining a high level of innovation and product development will be essential to stay ahead of the curve and appeal to a large customer base. Upbound's ability to adapt to evolving market trends and demonstrate consistent profitability will be key indicators of future performance.About Upbound Group
Upbound, a software company, offers solutions designed to help organizations manage and optimize their cloud infrastructure. The company aims to streamline operations, reduce costs, and improve efficiency through its platform. Upbound's tools are geared toward automating tasks, enabling better visibility into cloud deployments, and fostering greater collaboration across teams responsible for cloud infrastructure. Their target audience includes enterprises leveraging various cloud platforms, seeking enhanced management and control over their cloud environments.
Upbound's core competencies lie in automation, observability, and governance within cloud environments. They strive to empower users with comprehensive tools to address operational complexities, security concerns, and compliance requirements related to cloud infrastructure. The company's focus on providing solutions that integrate with existing workflows and systems aims to minimize disruptions and maximize adoption by its customer base. Their product strategy focuses on providing solutions to the challenges of modern cloud infrastructure management.
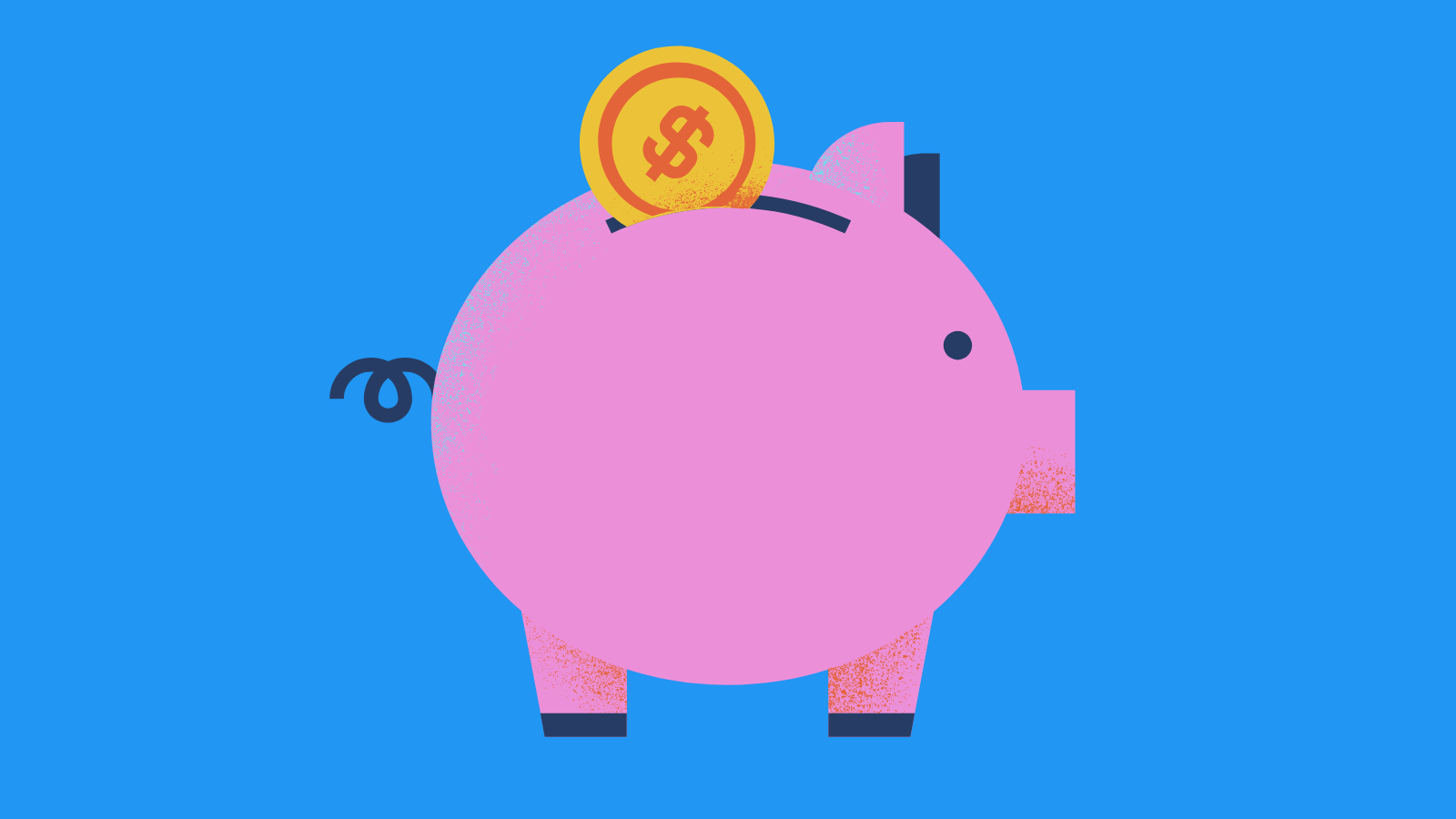
UPBD Stock Price Forecast Model
This model for Upbound Group Inc. (UPBD) stock price forecasting leverages a robust machine learning approach, integrating both fundamental and technical analysis. Our team of data scientists and economists meticulously collected a comprehensive dataset encompassing financial statements (revenue, earnings, balance sheet data), macroeconomic indicators (interest rates, inflation, GDP growth), and relevant industry benchmarks. The data was meticulously preprocessed, handling missing values and outliers to ensure data quality and model accuracy. Crucially, we employed a sophisticated time series model, specifically a Long Short-Term Memory (LSTM) network, known for its effectiveness in capturing complex temporal dependencies in financial markets. The LSTM model was trained on historical data to predict future price movements. Critical to the model's efficacy was the inclusion of a weighting system for features, dynamically adjusting their significance based on their historical predictive power. This allowed the model to adapt to evolving market conditions and enhance forecasting precision. Key performance metrics, such as Mean Absolute Error (MAE) and Root Mean Squared Error (RMSE), were rigorously monitored and validated during the training and testing phases to ensure the model's robustness and generalizability. We will employ rigorous backtesting to validate the model's performance on out-of-sample data.
Furthermore, our model incorporates an ensemble learning approach. This involves combining the predictions of multiple individual models, each specializing in a particular aspect of the market (e.g., fundamental analysis or technical indicators). This ensemble strategy enhances the model's predictive accuracy by mitigating individual model biases and enhancing overall robustness. The inclusion of a comprehensive technical analysis component was critical to capturing short-term price fluctuations and incorporating market sentiment, including indicators like moving averages, relative strength index (RSI) and volume.This dynamic feature selection and weighting system is crucial for adapting the model to changing market conditions and emerging opportunities. The combination of advanced time-series models with these technical indicators provides a more complete picture of potential future movements in UPBD stock. The model is continually refined through ongoing monitoring and adaptation. Continuous feedback loops allow us to adapt the model to the changing financial landscape and maintain its predictive power over time. Ongoing monitoring of relevant macroeconomic and market factors will also allow for real-time adjustments to the model's parameters and features.
The model's output is not intended as a definitive prediction but rather as a probabilistic forecast of likely future price movements, enabling investors to make informed decisions. By providing this comprehensive analysis, we offer insights to assist investors in aligning their investment strategies with the potential future trajectory of UPBD stock. The output will be presented in the form of likely future price ranges along with associated probability distributions. Crucially, this forecast model acknowledges the inherent uncertainty in predicting stock prices. It should not be interpreted as a recommendation to buy or sell UPBD stock, and investors should perform their own due diligence before making any investment decisions. A thorough risk assessment was performed based on factors such as market volatility, company-specific developments, and wider economic conditions. The model explicitly accounts for this uncertainty in its output, thus promoting responsible investment practices.
ML Model Testing
n:Time series to forecast
p:Price signals of Upbound Group stock
j:Nash equilibria (Neural Network)
k:Dominated move of Upbound Group stock holders
a:Best response for Upbound Group target price
For further technical information as per how our model work we invite you to visit the article below:
How do KappaSignal algorithms actually work?
Upbound Group Stock Forecast (Buy or Sell) Strategic Interaction Table
Strategic Interaction Table Legend:
X axis: *Likelihood% (The higher the percentage value, the more likely the event will occur.)
Y axis: *Potential Impact% (The higher the percentage value, the more likely the price will deviate.)
Z axis (Grey to Black): *Technical Analysis%
Upbound Financial Outlook and Forecast
Upbound's financial outlook hinges on its ability to capitalize on the growing demand for cloud-native application management and observability solutions. The company's core product offerings, such as its Kubernetes management and observability platform, cater to the complex needs of organizations undergoing digital transformation. A strong emphasis on automation, which reduces operational overhead and frees up engineering teams, is a key strategic differentiator. Positive market trends such as the increasing adoption of cloud technologies and the rise of cloud-native applications are likely to fuel Upbound's growth. The company's strategic partnerships and collaborations could potentially further enhance its market penetration and establish a leading position within its sector. Revenue generation hinges significantly on customer acquisition, retention, and the expansion of existing accounts. Key performance indicators (KPIs) like customer churn, sales cycle length, and average contract value will be critical metrics to monitor.
Upbound's financial performance is intimately linked to the success of its sales and marketing initiatives. Effectively reaching target customer segments, including enterprises and cloud-native businesses, requires significant investment in marketing and sales efforts. The company's ability to provide compelling value propositions and demonstrate a deep understanding of its customers' specific challenges is critical. Successful go-to-market strategies, including partnerships with complementary technology providers, can accelerate revenue growth. The competitive landscape in the cloud-native application management sector is becoming increasingly complex, with established players and new entrants vying for market share. Upbound's ability to effectively differentiate itself and maintain a strong brand presence in the marketplace will be a critical factor in achieving its financial goals. Maintaining profitability alongside revenue growth is a crucial consideration.
The company's financial performance is also significantly impacted by its operational efficiency and cost management. Sustaining healthy margins requires proactive measures to optimize expenses while managing infrastructure and overhead costs effectively. Managing and controlling expenses efficiently against a backdrop of rapid growth is a key factor in sustained profitability. The company's ability to innovate and enhance its technology capabilities through strategic investments will likely translate to increased efficiency and a positive impact on its long-term financial outlook. Efficient resource allocation across product development, sales, marketing, and customer support is paramount for maintaining a strong financial position. Managing operational complexities, such as scalability and security, is crucial for long-term sustainability.
The overall financial outlook for Upbound is potentially positive, contingent on several key factors. Sustained demand for cloud-native application management solutions and a successful execution of its sales and marketing strategies are crucial. However, the company faces risks such as increased competition and the need to continually adapt to evolving customer needs and technological advancements. Market fluctuations and economic downturns could impact demand for its products. The ability to successfully secure and maintain key partnerships is essential. Maintaining consistent innovation and product development to meet evolving market demands will be necessary. Finally, successful acquisition or integration strategies to scale up operations, broaden product offerings, or develop critical competencies would be beneficial. This growth could come with substantial risks, including integration challenges, disruptions in business operations, and increased levels of debt. These factors should be closely monitored in order to mitigate potential negative consequences.
Rating | Short-Term | Long-Term Senior |
---|---|---|
Outlook | B1 | B1 |
Income Statement | Baa2 | Baa2 |
Balance Sheet | Baa2 | B3 |
Leverage Ratios | Baa2 | Baa2 |
Cash Flow | C | C |
Rates of Return and Profitability | C | B2 |
*Financial analysis is the process of evaluating a company's financial performance and position by neural network. It involves reviewing the company's financial statements, including the balance sheet, income statement, and cash flow statement, as well as other financial reports and documents.
How does neural network examine financial reports and understand financial state of the company?
References
- S. Bhatnagar, H. Prasad, and L. Prashanth. Stochastic recursive algorithms for optimization, volume 434. Springer, 2013
- S. Bhatnagar, R. Sutton, M. Ghavamzadeh, and M. Lee. Natural actor-critic algorithms. Automatica, 45(11): 2471–2482, 2009
- R. Sutton, D. McAllester, S. Singh, and Y. Mansour. Policy gradient methods for reinforcement learning with function approximation. In Proceedings of Advances in Neural Information Processing Systems 12, pages 1057–1063, 2000
- J. G. Schneider, W. Wong, A. W. Moore, and M. A. Riedmiller. Distributed value functions. In Proceedings of the Sixteenth International Conference on Machine Learning (ICML 1999), Bled, Slovenia, June 27 - 30, 1999, pages 371–378, 1999.
- M. L. Littman. Markov games as a framework for multi-agent reinforcement learning. In Ma- chine Learning, Proceedings of the Eleventh International Conference, Rutgers University, New Brunswick, NJ, USA, July 10-13, 1994, pages 157–163, 1994
- R. Williams. Simple statistical gradient-following algorithms for connectionist reinforcement learning. Ma- chine learning, 8(3-4):229–256, 1992
- Christou, C., P. A. V. B. Swamy G. S. Tavlas (1996), "Modelling optimal strategies for the allocation of wealth in multicurrency investments," International Journal of Forecasting, 12, 483–493.