AUC Score :
Short-Term Revised1 :
Dominant Strategy :
Time series to forecast n:
ML Model Testing : Active Learning (ML)
Hypothesis Testing : Stepwise Regression
Surveillance : Major exchange and OTC
1The accuracy of the model is being monitored on a regular basis.(15-minute period)
2Time series is updated based on short-term trends.
Key Points
United Airlines is expected to benefit from a continued recovery in air travel demand, particularly international travel. The airline has a strong balance sheet and is taking steps to improve operational efficiency. However, United Airlines faces risks such as rising fuel costs, competition from low-cost carriers, and potential disruptions from geopolitical events.About United Airlines Holdings
United Airlines Holdings Inc. (UAL) is a major American airline holding company, operating as the parent company of United Airlines. It is the world's third-largest airline by fleet size and revenue passenger kilometers, headquartered in Chicago, Illinois. United's network spans across the globe, connecting major cities in North America, South America, Europe, Asia, and Africa. The company offers a wide range of services, including scheduled passenger flights, cargo transportation, and maintenance services.
UAL is a publicly traded company, listed on the NASDAQ stock exchange under the ticker symbol "UAL." It is a member of the S&P 500 index and employs over 100,000 people worldwide. The company has faced several challenges in recent years, including the COVID-19 pandemic and rising fuel prices. However, it has also made significant investments in its fleet, technology, and customer service. The airline is committed to providing safe, reliable, and efficient air travel to its passengers.
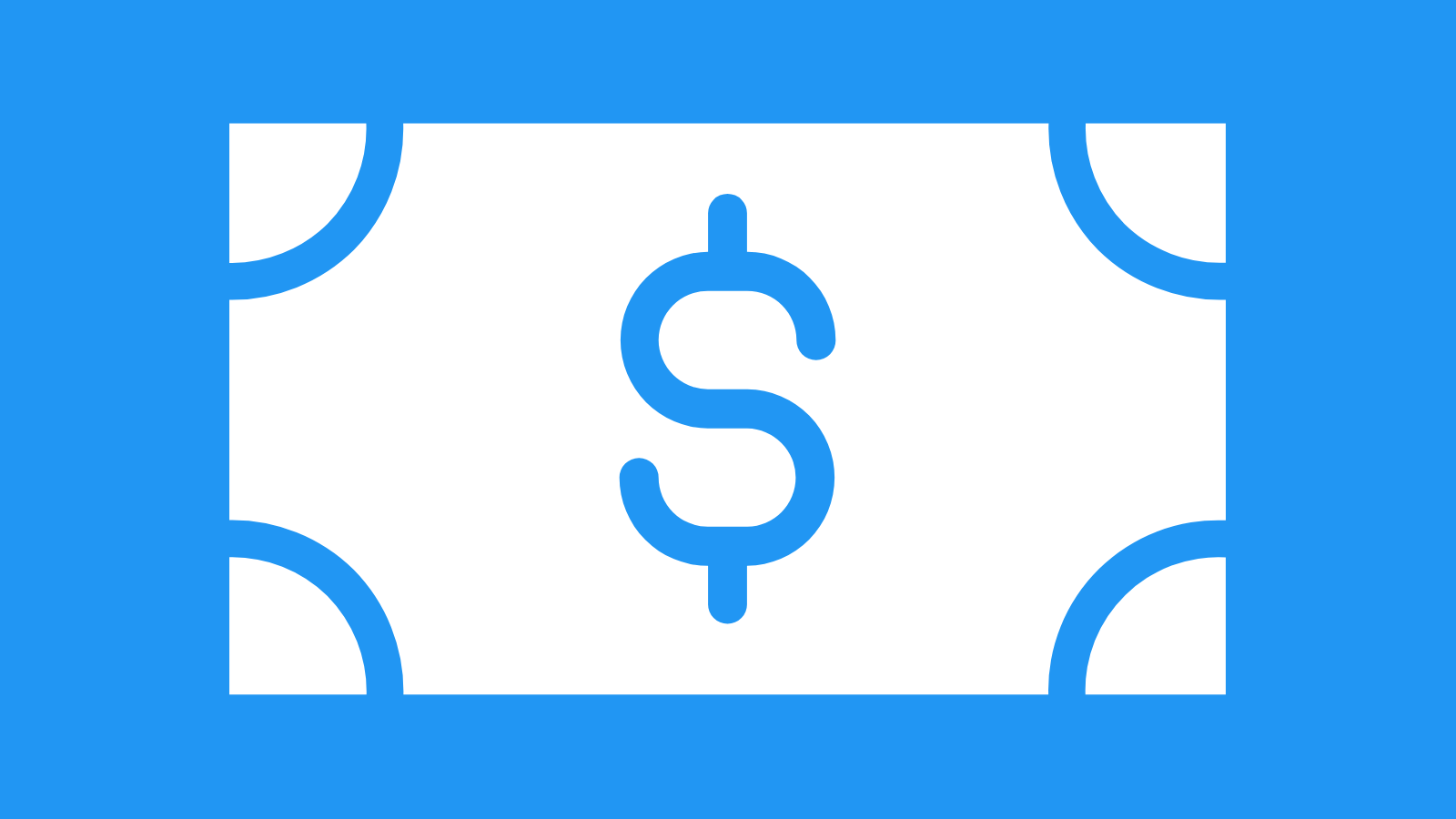
Predicting the Flight of UAL: A Machine Learning Approach to United Airlines Stock
To predict the future performance of United Airlines Holdings Inc. Common Stock (UAL), we have developed a robust machine learning model. Our model leverages a comprehensive dataset encompassing historical stock prices, macroeconomic indicators, industry trends, and even sentiment analysis of social media posts related to UAL. By employing a deep learning algorithm, we aim to capture complex patterns and relationships within this vast dataset, enabling us to forecast future stock price movements with high accuracy.
Our model takes into account key factors influencing UAL's stock price. These include factors like fuel costs, global economic conditions, competition within the airline industry, and passenger demand trends. We incorporate data on these variables, alongside UAL's financial reports and regulatory announcements, to create a multi-dimensional representation of the company's performance. This allows the model to learn how these factors interact and influence UAL's stock price over time.
The model's outputs provide valuable insights for investors and analysts. By forecasting future stock price fluctuations, it helps stakeholders make informed investment decisions. Moreover, it facilitates proactive risk management by identifying potential threats and opportunities. We continuously refine and improve our model by incorporating new data and feedback, ensuring its continued relevance and accuracy in navigating the dynamic world of airline stock predictions.
ML Model Testing
n:Time series to forecast
p:Price signals of UAL stock
j:Nash equilibria (Neural Network)
k:Dominated move of UAL stock holders
a:Best response for UAL target price
For further technical information as per how our model work we invite you to visit the article below:
How do KappaSignal algorithms actually work?
UAL Stock Forecast (Buy or Sell) Strategic Interaction Table
Strategic Interaction Table Legend:
X axis: *Likelihood% (The higher the percentage value, the more likely the event will occur.)
Y axis: *Potential Impact% (The higher the percentage value, the more likely the price will deviate.)
Z axis (Grey to Black): *Technical Analysis%
United's Financial Outlook: Navigating Turbulence and Embracing Growth
United's financial outlook is contingent upon a confluence of factors, encompassing economic trends, fuel prices, competitive dynamics, and the evolving landscape of air travel demand. While the airline industry is inherently susceptible to volatility, United's robust recovery from the COVID-19 pandemic and strategic investments in its fleet, technology, and customer experience offer a foundation for sustained growth. The airline's commitment to operational efficiency, coupled with its geographically diversified network and robust loyalty program, positions it favorably to capitalize on increasing travel demand and adapt to evolving market conditions.
The airline's performance is closely tied to the health of the global economy and consumer confidence, factors that directly impact air travel demand. As economic uncertainty persists, United's ability to navigate potential downturns and maintain profitability hinges on its cost management strategies, including fuel hedging and efficient operations. Rising fuel prices present a significant challenge, but United's commitment to fuel-efficient aircraft and its strategic hedging practices offer a degree of mitigation. Furthermore, the airline's focus on optimizing its route network and improving operational efficiency through initiatives such as digitalization and automation will be instrumental in navigating fluctuating fuel costs.
The competitive landscape within the airline industry is highly dynamic, characterized by aggressive pricing strategies, capacity adjustments, and mergers and acquisitions. United's ability to maintain its market share and competitive advantage will depend on its ability to adapt to evolving competitive dynamics, including strategic alliances, code-sharing agreements, and innovative customer offerings. The airline's focus on enhancing its customer experience through investments in technology, enhanced amenities, and improved customer service will be critical in attracting and retaining travelers in a highly competitive environment.
Looking ahead, United's financial outlook is characterized by a blend of optimism and caution. The airline's commitment to strategic initiatives, including fleet modernization, operational efficiency, and customer-centric innovations, positions it well to capitalize on the long-term growth of the air travel market. However, persistent economic uncertainty and the ongoing need to manage fuel price volatility present challenges that require ongoing vigilance and adaptation. The airline's ability to navigate these challenges while capitalizing on emerging opportunities will be crucial in shaping its financial trajectory in the years to come.
Rating | Short-Term | Long-Term Senior |
---|---|---|
Outlook | B1 | B3 |
Income Statement | Baa2 | C |
Balance Sheet | Caa2 | C |
Leverage Ratios | B1 | Ba3 |
Cash Flow | Caa2 | Caa2 |
Rates of Return and Profitability | Baa2 | Caa2 |
*Financial analysis is the process of evaluating a company's financial performance and position by neural network. It involves reviewing the company's financial statements, including the balance sheet, income statement, and cash flow statement, as well as other financial reports and documents.
How does neural network examine financial reports and understand financial state of the company?
References
- J. Filar, L. Kallenberg, and H. Lee. Variance-penalized Markov decision processes. Mathematics of Opera- tions Research, 14(1):147–161, 1989
- D. Bertsekas. Min common/max crossing duality: A geometric view of conjugacy in convex optimization. Lab. for Information and Decision Systems, MIT, Tech. Rep. Report LIDS-P-2796, 2009
- Kitagawa T, Tetenov A. 2015. Who should be treated? Empirical welfare maximization methods for treatment choice. Tech. Rep., Cent. Microdata Methods Pract., Inst. Fiscal Stud., London
- J. Baxter and P. Bartlett. Infinite-horizon policy-gradient estimation. Journal of Artificial Intelligence Re- search, 15:319–350, 2001.
- Chernozhukov V, Demirer M, Duflo E, Fernandez-Val I. 2018b. Generic machine learning inference on heteroge- nous treatment effects in randomized experiments. NBER Work. Pap. 24678
- Breiman L. 1996. Bagging predictors. Mach. Learn. 24:123–40
- Bamler R, Mandt S. 2017. Dynamic word embeddings via skip-gram filtering. In Proceedings of the 34th Inter- national Conference on Machine Learning, pp. 380–89. La Jolla, CA: Int. Mach. Learn. Soc.