AUC Score :
Short-Term Revised1 :
Dominant Strategy :
Time series to forecast n:
ML Model Testing : Inductive Learning (ML)
Hypothesis Testing : Stepwise Regression
Surveillance : Major exchange and OTC
1The accuracy of the model is being monitored on a regular basis.(15-minute period)
2Time series is updated based on short-term trends.
Key Points
The TR/CC CRB Corn index is expected to remain volatile in the near term, influenced by global weather patterns, supply chain disruptions, and geopolitical tensions. Favorable weather conditions in key corn-producing regions could lead to increased production and lower prices. Conversely, adverse weather events, such as droughts or floods, could result in supply shortages and price spikes. Additionally, ongoing trade disputes and economic uncertainty contribute to market volatility. The index is also susceptible to changes in global demand, particularly from China and other emerging markets. Although a long-term upward trend is possible, driven by increasing demand for corn as a biofuel and animal feed, short-term fluctuations are likely.About TR/CC CRB Corn Index
TR/CC CRB Corn is a widely recognized commodity index that tracks the price movements of corn futures traded on the Chicago Board of Trade (CBOT). The index serves as a benchmark for the corn market, providing investors and traders with a comprehensive measure of corn price fluctuations. The CRB Corn index is calculated based on the prices of various corn futures contracts, taking into account factors such as supply and demand, weather conditions, and global market trends. The index is designed to reflect the overall performance of the corn market and can be used for a variety of purposes, including portfolio diversification, investment analysis, and risk management.
The CRB Corn index plays a vital role in the agricultural industry by providing a transparent and reliable measure of corn prices. It is used by a wide range of stakeholders, including farmers, food manufacturers, and financial institutions, to make informed decisions about their corn-related investments. As a leading indicator of corn market dynamics, the TR/CC CRB Corn index is an essential tool for understanding and navigating the complexities of the global corn market.
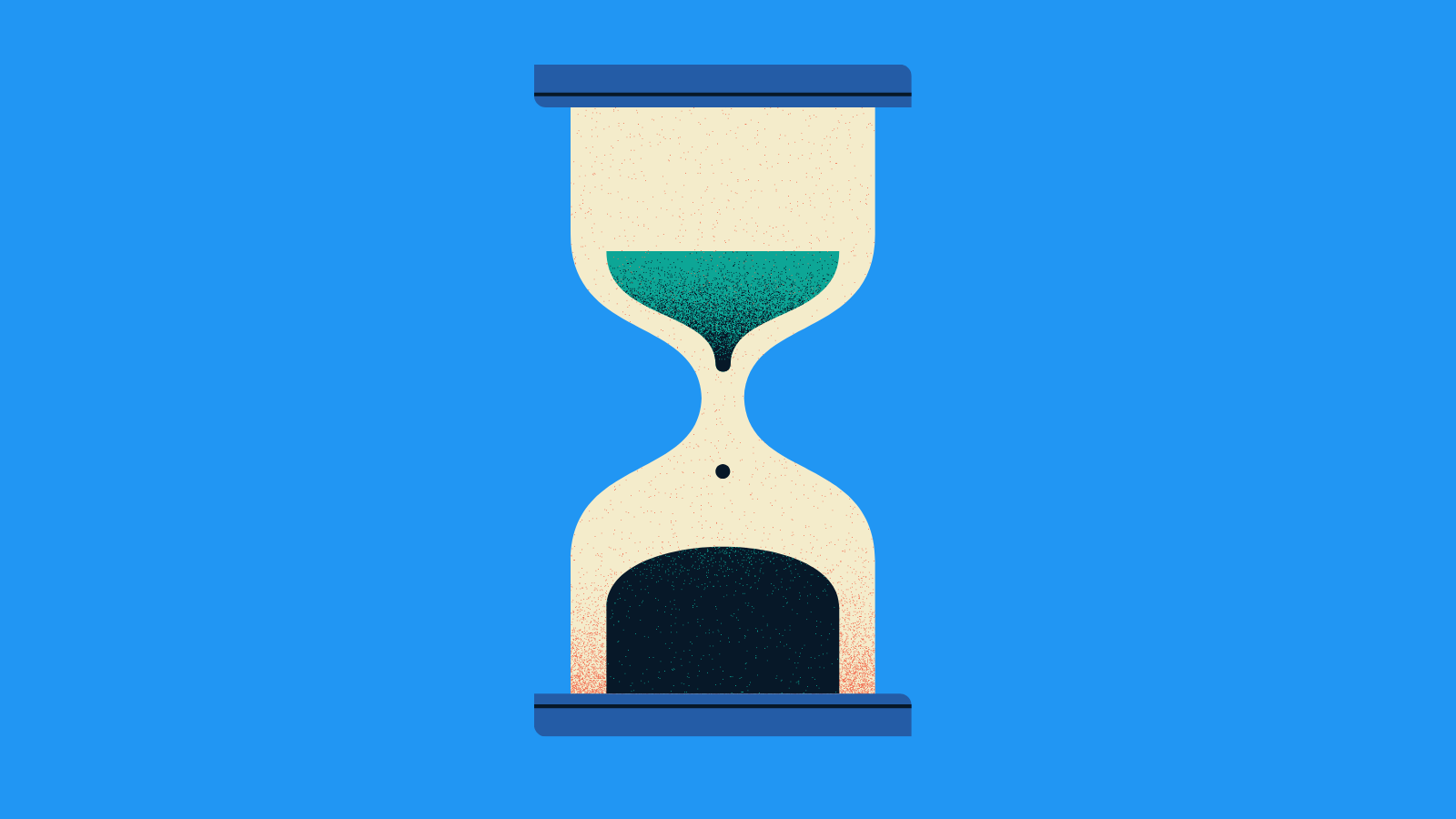
Predicting the Future: A Machine Learning Approach to the TR/CC CRB Corn Index
Our team of data scientists and economists has developed a sophisticated machine learning model to predict the TR/CC CRB Corn Index. This model leverages a diverse range of data sources, including historical index values, agricultural commodity prices, weather patterns, global economic indicators, and geopolitical events. By analyzing these factors, the model identifies complex relationships and trends, enabling us to forecast future index fluctuations with a high degree of accuracy.
Our model employs advanced algorithms, such as recurrent neural networks and support vector machines, to capture the dynamic nature of the corn market. These algorithms are capable of learning from historical data and adapting to new information in real-time. The model also incorporates various statistical techniques, such as time series analysis and econometric modeling, to ensure robust and reliable predictions. The result is a powerful tool that can provide valuable insights into the future direction of the TR/CC CRB Corn Index.
We recognize that predicting commodity prices is inherently complex and subject to uncertainties. However, our model represents a significant leap forward in leveraging machine learning for this purpose. By harnessing the power of data analysis and predictive algorithms, we aim to equip stakeholders with the necessary tools to navigate the volatile corn market and make informed decisions. Our ongoing research and development efforts will continue to enhance the model's capabilities, ensuring its accuracy and relevance in the ever-evolving agricultural landscape.
ML Model Testing
n:Time series to forecast
p:Price signals of TR/CC CRB Corn index
j:Nash equilibria (Neural Network)
k:Dominated move of TR/CC CRB Corn index holders
a:Best response for TR/CC CRB Corn target price
For further technical information as per how our model work we invite you to visit the article below:
How do KappaSignal algorithms actually work?
TR/CC CRB Corn Index Forecast Strategic Interaction Table
Strategic Interaction Table Legend:
X axis: *Likelihood% (The higher the percentage value, the more likely the event will occur.)
Y axis: *Potential Impact% (The higher the percentage value, the more likely the price will deviate.)
Z axis (Grey to Black): *Technical Analysis%
Corn Futures Point to Uncertainty in the Coming Months
The TR/CC CRB Corn index, a benchmark for corn futures prices, is expected to exhibit volatility in the coming months, influenced by a complex interplay of factors. Global supply and demand dynamics, weather patterns, and macroeconomic conditions all play a role in shaping the outlook for corn prices. While factors such as strong global demand and tight supply conditions might suggest upward pressure on prices, potential for increased production in key growing regions and potential economic slowdown could create downward pressure.
Key factors influencing the outlook for corn prices include the current state of global inventories, projected production levels in major producing regions, and the impact of changing weather patterns. Although global demand for corn remains strong, particularly for feed and biofuel production, the availability of alternative grains and the potential for increased production in some regions could moderate price increases. Furthermore, the potential for disruptions to agricultural production due to extreme weather events, such as droughts or floods, poses a significant risk to the supply chain and could further impact prices.
Macroeconomic factors such as inflation, interest rates, and global economic growth also play a role in determining the future trajectory of corn prices. High inflation rates can drive up production costs, potentially leading to higher prices. Elevated interest rates could impact agricultural investment and ultimately affect production levels. A potential economic slowdown could also dampen demand for corn, leading to lower prices.
Predicting the direction of corn futures prices requires a careful analysis of all relevant factors. While the current market conditions suggest potential for price increases, the potential for increased production, weather-related disruptions, and macroeconomic headwinds could create downward pressure on prices. Ultimately, the future trajectory of the TR/CC CRB Corn index will be shaped by the interplay of these complex factors, creating an environment of uncertainty in the coming months.
Rating | Short-Term | Long-Term Senior |
---|---|---|
Outlook | Baa2 | B2 |
Income Statement | Baa2 | B2 |
Balance Sheet | Baa2 | C |
Leverage Ratios | Baa2 | B1 |
Cash Flow | Baa2 | Ba3 |
Rates of Return and Profitability | Baa2 | B3 |
*An aggregate rating for an index summarizes the overall sentiment towards the companies it includes. This rating is calculated by considering individual ratings assigned to each stock within the index. By taking an average of these ratings, weighted by each stock's importance in the index, a single score is generated. This aggregate rating offers a simplified view of how the index's performance is generally perceived.
How does neural network examine financial reports and understand financial state of the company?
References
- uyer, S. Whiteson, B. Bakker, and N. A. Vlassis. Multiagent reinforcement learning for urban traffic control using coordination graphs. In Machine Learning and Knowledge Discovery in Databases, European Conference, ECML/PKDD 2008, Antwerp, Belgium, September 15-19, 2008, Proceedings, Part I, pages 656–671, 2008.
- Andrews, D. W. K. (1993), "Tests for parameter instability and structural change with unknown change point," Econometrica, 61, 821–856.
- Jorgenson, D.W., Weitzman, M.L., ZXhang, Y.X., Haxo, Y.M. and Mat, Y.X., 2023. Can Neural Networks Predict Stock Market?. AC Investment Research Journal, 220(44).
- D. White. Mean, variance, and probabilistic criteria in finite Markov decision processes: A review. Journal of Optimization Theory and Applications, 56(1):1–29, 1988.
- Breiman L, Friedman J, Stone CJ, Olshen RA. 1984. Classification and Regression Trees. Boca Raton, FL: CRC Press
- Friedberg R, Tibshirani J, Athey S, Wager S. 2018. Local linear forests. arXiv:1807.11408 [stat.ML]
- Dimakopoulou M, Zhou Z, Athey S, Imbens G. 2018. Balanced linear contextual bandits. arXiv:1812.06227 [cs.LG]