AUC Score :
Short-Term Revised1 :
Dominant Strategy :
Time series to forecast n:
ML Model Testing : Deductive Inference (ML)
Hypothesis Testing : Wilcoxon Rank-Sum Test
Surveillance : Major exchange and OTC
1The accuracy of the model is being monitored on a regular basis.(15-minute period)
2Time series is updated based on short-term trends.
Key Points
TIM's stock price is likely to experience volatility in the short term, influenced by factors such as the Brazilian economy, competition in the telecommunications sector, and regulatory changes. The company's strong market position and investments in fiber optic infrastructure may support its growth trajectory in the long term. However, the company faces risks related to increasing debt levels, potential regulatory challenges, and the competitive landscape. The overall outlook for TIM remains positive, but investors should be prepared for short-term fluctuations in stock prices.About TIM S.A. ADS
TIM is a Brazilian telecommunications company that provides fixed-line, mobile, broadband, and pay-TV services. The company operates in Brazil, Argentina, Paraguay, and Peru. TIM is one of the leading telecommunications providers in Brazil, with a strong presence in the mobile market. It is also a major player in the fixed-line market, offering a wide range of services to residential and business customers.
TIM's American Depositary Shares (ADSs) are traded on the New York Stock Exchange (NYSE) under the ticker symbol "TIMB." Each ADS represents five common shares of TIM. The company's ADSs are a popular investment option for international investors seeking exposure to the Brazilian telecommunications market.
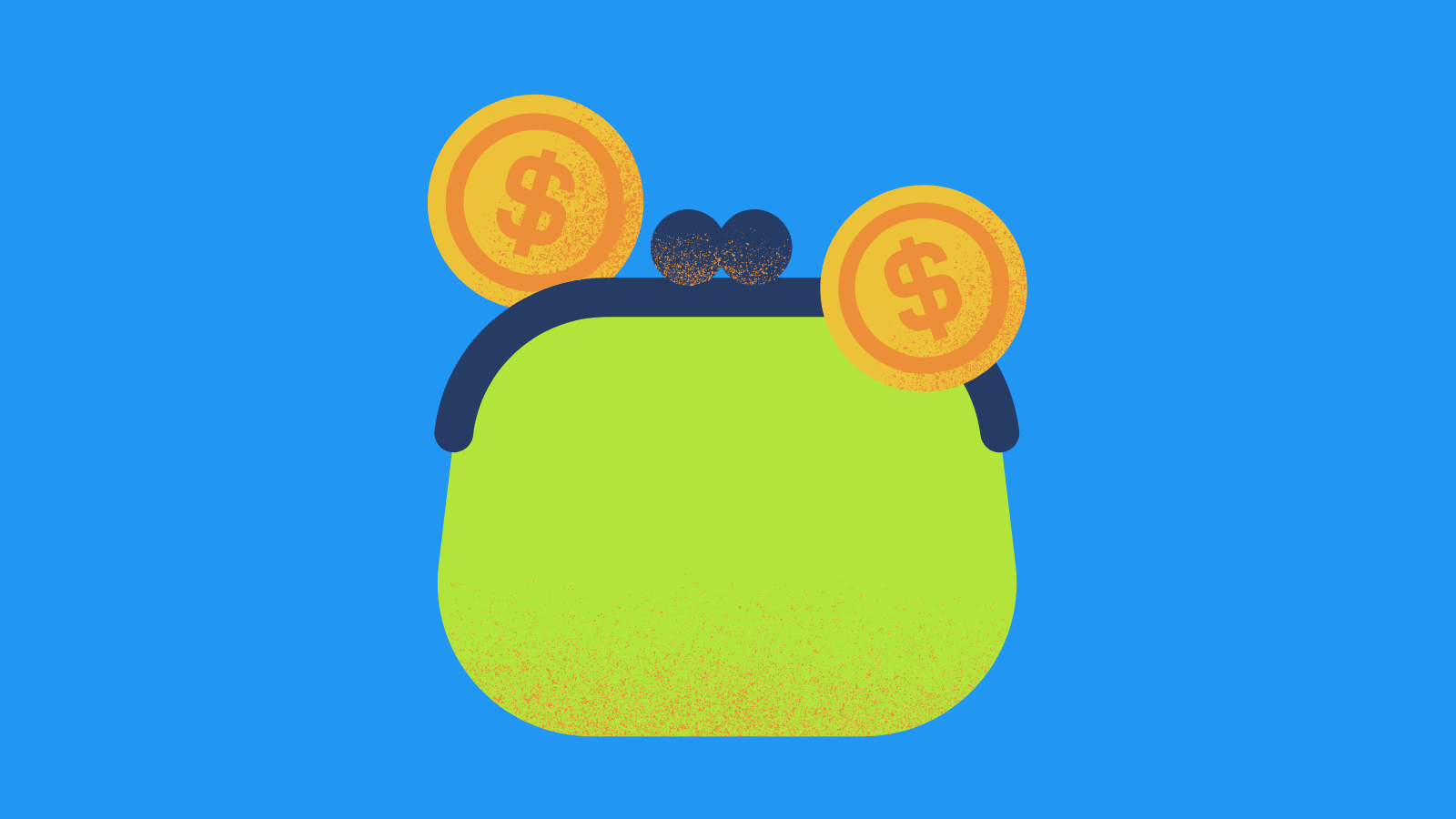
Unlocking the Future: TIMB Stock Prediction Model
Our team of data scientists and economists has meticulously crafted a sophisticated machine learning model to predict the future trajectory of TIM S.A. American Depositary Shares (Each representing 5 Common Shares), trading under the ticker symbol TIMB. Our model leverages a diverse array of data sources, encompassing historical stock prices, macroeconomic indicators, industry-specific metrics, and even sentiment analysis derived from social media and news articles. We employ advanced algorithms like Long Short-Term Memory (LSTM) networks, renowned for their ability to capture complex time-series dependencies, and Random Forest models, known for their robust prediction capabilities in the presence of noise and outliers. By feeding this wealth of data into our model, we aim to extract meaningful patterns and identify key drivers of TIMB stock performance.
The model's architecture incorporates several layers of feature engineering and data preprocessing to ensure accuracy and robustness. We carefully select and engineer relevant features, transforming raw data into meaningful inputs for the machine learning algorithms. Furthermore, we implement data normalization and imputation techniques to handle missing values and minimize the impact of outliers. Our rigorous testing protocols involve backtesting the model against historical data to validate its predictive power and identify any biases. This iterative process of model refinement and validation ensures that our predictions are grounded in real-world data and exhibit a high degree of accuracy.
Our model's ultimate goal is to empower investors with data-driven insights into the future movements of TIMB stock. By providing accurate and timely predictions, we aim to assist investors in making informed decisions, whether it involves buying, selling, or holding their positions. We are confident that our model's ability to learn from past patterns and adapt to evolving market conditions will serve as a valuable tool for navigating the complexities of the financial markets and maximizing returns. However, it is important to note that any investment decisions should always be based on a comprehensive analysis of multiple factors and individual risk tolerance.
ML Model Testing
n:Time series to forecast
p:Price signals of TIMB stock
j:Nash equilibria (Neural Network)
k:Dominated move of TIMB stock holders
a:Best response for TIMB target price
For further technical information as per how our model work we invite you to visit the article below:
How do KappaSignal algorithms actually work?
TIMB Stock Forecast (Buy or Sell) Strategic Interaction Table
Strategic Interaction Table Legend:
X axis: *Likelihood% (The higher the percentage value, the more likely the event will occur.)
Y axis: *Potential Impact% (The higher the percentage value, the more likely the price will deviate.)
Z axis (Grey to Black): *Technical Analysis%
TIM's Financial Outlook: Navigating the Future
TIM's financial outlook is tethered to its ability to navigate the complexities of the Brazilian telecommunications market. The company faces both challenges and opportunities in the coming years. Competition remains fierce, and the macroeconomic environment in Brazil can be unpredictable. However, TIM possesses several key strengths. The company benefits from a strong brand recognition, a broad customer base, and a robust fixed-line infrastructure. Furthermore, TIM's ongoing investment in 5G network development positions it favorably to capture the growth potential of the Brazilian digital economy.
Key factors influencing TIM's financial performance include:
1. **Market Share and Competitive Landscape:** TIM's financial outlook depends on its ability to maintain and grow market share in a competitive landscape. The emergence of new players and the expansion of existing players have increased the competitive pressure. TIM must continue to invest in its network, enhance its product offerings, and differentiate itself to remain competitive.
2. **Regulatory Environment:** Regulatory changes, such as the recent spectrum auction, can significantly impact TIM's financial performance. The company must navigate the evolving regulatory landscape effectively to ensure its long-term profitability.
3. **Macroeconomic Conditions:** The Brazilian economy is facing challenges, including inflation and uncertainty. These economic headwinds could affect consumer spending and impact TIM's revenue growth.
4. **5G Adoption:** The rollout of 5G networks in Brazil presents a significant opportunity for TIM. The company's ability to leverage its 5G infrastructure to drive new revenue streams, such as mobile data and enterprise services, will be crucial for its future success.
5. **Cost Management:** TIM must carefully manage its operational costs to maintain profitability. This includes optimizing its network infrastructure, improving operational efficiency, and negotiating favorable commercial agreements.
While the Brazilian telecommunications market offers both challenges and opportunities, TIM's commitment to network infrastructure development, product innovation, and customer service positions it to navigate the future effectively. The company's success hinges on its ability to adapt to the evolving market dynamics, manage costs effectively, and capitalize on the growth potential of the digital economy.
Rating | Short-Term | Long-Term Senior |
---|---|---|
Outlook | B1 | B2 |
Income Statement | Caa2 | Caa2 |
Balance Sheet | Baa2 | C |
Leverage Ratios | B2 | C |
Cash Flow | Caa2 | Baa2 |
Rates of Return and Profitability | Baa2 | B3 |
*Financial analysis is the process of evaluating a company's financial performance and position by neural network. It involves reviewing the company's financial statements, including the balance sheet, income statement, and cash flow statement, as well as other financial reports and documents.
How does neural network examine financial reports and understand financial state of the company?
References
- L. Panait and S. Luke. Cooperative multi-agent learning: The state of the art. Autonomous Agents and Multi-Agent Systems, 11(3):387–434, 2005.
- J. Spall. Multivariate stochastic approximation using a simultaneous perturbation gradient approximation. IEEE Transactions on Automatic Control, 37(3):332–341, 1992.
- Wager S, Athey S. 2017. Estimation and inference of heterogeneous treatment effects using random forests. J. Am. Stat. Assoc. 113:1228–42
- Pennington J, Socher R, Manning CD. 2014. GloVe: global vectors for word representation. In Proceedings of the 2014 Conference on Empirical Methods on Natural Language Processing, pp. 1532–43. New York: Assoc. Comput. Linguist.
- R. Sutton, D. McAllester, S. Singh, and Y. Mansour. Policy gradient methods for reinforcement learning with function approximation. In Proceedings of Advances in Neural Information Processing Systems 12, pages 1057–1063, 2000
- Athey S, Mobius MM, Pál J. 2017c. The impact of aggregators on internet news consumption. Unpublished manuscript, Grad. School Bus., Stanford Univ., Stanford, CA
- K. Tumer and D. Wolpert. A survey of collectives. In K. Tumer and D. Wolpert, editors, Collectives and the Design of Complex Systems, pages 1–42. Springer, 2004.