AUC Score :
Short-Term Revised1 :
Dominant Strategy :
Time series to forecast n:
ML Model Testing : Modular Neural Network (Market Volatility Analysis)
Hypothesis Testing : Stepwise Regression
Surveillance : Major exchange and OTC
1The accuracy of the model is being monitored on a regular basis.(15-minute period)
2Time series is updated based on short-term trends.
Key Points
SunCoke Energy's future performance is contingent upon several factors, including global steel demand, metallurgical coal prices, and the company's ability to optimize its operations. The company's focus on cost reduction and operational efficiency may lead to improved profitability, but its reliance on a single industry, the steel sector, exposes it to volatility. Should global steel demand weaken or coal prices decline, SunCoke Energy's revenue and earnings could be negatively impacted. However, the company's strategic initiatives and long-term contracts provide some level of stability.About SunCoke Energy
SunCoke Energy is a leading producer of metallurgical coke, a key ingredient in steelmaking, in the United States. The company operates coke plants in Pennsylvania, West Virginia, Alabama, and Illinois, supplying coke to major steel producers across the country. SunCoke Energy also owns and operates a network of coal mines and processing facilities, ensuring a reliable supply of high-quality coal for its coke production. The company is committed to sustainable operations, implementing environmental initiatives to minimize its impact on the environment.
SunCoke Energy also provides a range of services to its customers, including blending, pelletizing, and coke handling. The company's focus on quality, reliability, and customer service has made it a trusted partner to the steel industry. SunCoke Energy is committed to innovation and technology, investing in advanced processes and equipment to improve its efficiency and sustainability.
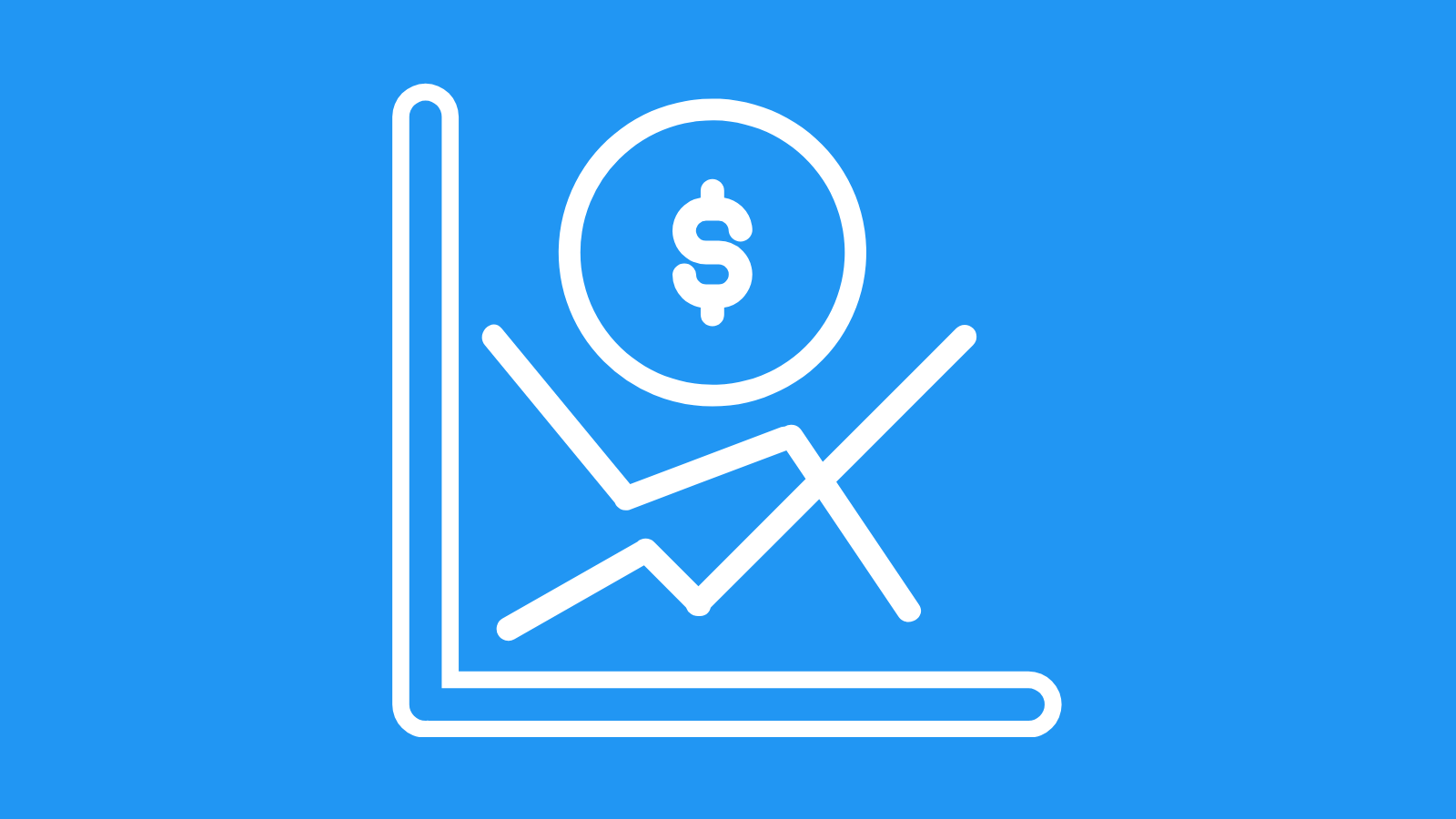
Predicting SunCoke Energy Inc. Stock Performance: A Machine Learning Approach
To develop a robust machine learning model for predicting the stock performance of SunCoke Energy Inc. (SXC), we will leverage a multifaceted approach incorporating historical stock data, relevant economic indicators, and industry-specific variables. Our model will utilize a combination of supervised and unsupervised learning algorithms. We will begin by gathering historical data for SXC, encompassing features such as daily closing price, trading volume, moving averages, and volatility metrics. This data will be preprocessed and cleansed to ensure accuracy and consistency. We will then incorporate external factors influencing the coal industry, including global coal prices, natural gas prices, and steel production levels. These economic indicators will serve as crucial predictors of SXC's financial performance.
Next, we will employ a variety of machine learning algorithms, such as Support Vector Machines (SVM), Random Forests, and Neural Networks, to train our model. These algorithms will be trained on the historical data, identifying patterns and relationships between the input features and the target variable, SXC's stock price. To enhance model performance, we will utilize techniques like feature engineering to extract relevant information from the raw data, including lagged variables and technical indicators. We will also employ cross-validation to assess the model's predictive accuracy and prevent overfitting.
Finally, we will continuously monitor and evaluate the model's performance using metrics like mean squared error (MSE) and R-squared, making necessary adjustments and updates as new data becomes available. This iterative process will ensure the model remains relevant and accurate in capturing the dynamic nature of the stock market. By integrating historical data, economic indicators, and industry-specific variables, our machine learning model will provide valuable insights into the future performance of SXC stock, empowering informed decision-making for investors and stakeholders.
ML Model Testing
n:Time series to forecast
p:Price signals of SXC stock
j:Nash equilibria (Neural Network)
k:Dominated move of SXC stock holders
a:Best response for SXC target price
For further technical information as per how our model work we invite you to visit the article below:
How do KappaSignal algorithms actually work?
SXC Stock Forecast (Buy or Sell) Strategic Interaction Table
Strategic Interaction Table Legend:
X axis: *Likelihood% (The higher the percentage value, the more likely the event will occur.)
Y axis: *Potential Impact% (The higher the percentage value, the more likely the price will deviate.)
Z axis (Grey to Black): *Technical Analysis%
SunCoke Energy's Financial Outlook: A Balanced Perspective
SunCoke Energy's (SNE) financial outlook is characterized by a mix of challenges and opportunities. While the company faces headwinds from a volatile and competitive global steel market, it benefits from a solid position in key metallurgical coke markets and a commitment to operational efficiency. The company is expected to navigate these complexities, achieving moderate growth and maintaining a healthy balance sheet.
The primary driver of SunCoke Energy's performance is the demand for metallurgical coke, a key input in steelmaking. The company's operations are concentrated in the United States, with significant exposure to the North American steel industry. While the steel market is experiencing some recovery, it remains subject to geopolitical risks, cyclical fluctuations, and intense competition from international producers. This volatility can impact the pricing of metallurgical coke, affecting SunCoke Energy's revenue and profitability.
However, SunCoke Energy is well-positioned to capitalize on opportunities within the steel market. The company has a strong network of coke production facilities strategically located near its key customers, enabling efficient logistics and cost optimization. Furthermore, SunCoke Energy has a proven track record of implementing cost-saving measures and optimizing production processes, which contribute to its resilience in a challenging environment. The company is actively pursuing growth opportunities in renewable energy and carbon capture technologies, demonstrating its commitment to long-term sustainability and diversification.
In summary, SunCoke Energy's financial outlook is marked by a balanced mix of challenges and opportunities. The company is well-positioned to weather short-term volatility and capitalize on long-term growth prospects in the steel and energy sectors. While its future performance will be influenced by macroeconomic factors, SunCoke Energy's focus on operational efficiency, strategic investments, and sustainability will contribute to its continued success in the years to come.
Rating | Short-Term | Long-Term Senior |
---|---|---|
Outlook | Ba3 | B1 |
Income Statement | Ba2 | B3 |
Balance Sheet | Baa2 | B1 |
Leverage Ratios | Ba2 | C |
Cash Flow | Baa2 | Baa2 |
Rates of Return and Profitability | C | Ba2 |
*Financial analysis is the process of evaluating a company's financial performance and position by neural network. It involves reviewing the company's financial statements, including the balance sheet, income statement, and cash flow statement, as well as other financial reports and documents.
How does neural network examine financial reports and understand financial state of the company?
References
- D. White. Mean, variance, and probabilistic criteria in finite Markov decision processes: A review. Journal of Optimization Theory and Applications, 56(1):1–29, 1988.
- Chernozhukov V, Chetverikov D, Demirer M, Duflo E, Hansen C, Newey W. 2017. Double/debiased/ Neyman machine learning of treatment effects. Am. Econ. Rev. 107:261–65
- S. Bhatnagar. An actor-critic algorithm with function approximation for discounted cost constrained Markov decision processes. Systems & Control Letters, 59(12):760–766, 2010
- S. J. Russell and A. Zimdars. Q-decomposition for reinforcement learning agents. In Machine Learning, Proceedings of the Twentieth International Conference (ICML 2003), August 21-24, 2003, Washington, DC, USA, pages 656–663, 2003.
- J. Harb and D. Precup. Investigating recurrence and eligibility traces in deep Q-networks. In Deep Reinforcement Learning Workshop, NIPS 2016, Barcelona, Spain, 2016.
- S. Bhatnagar and K. Lakshmanan. An online actor-critic algorithm with function approximation for con- strained Markov decision processes. Journal of Optimization Theory and Applications, 153(3):688–708, 2012.
- Imbens G, Wooldridge J. 2009. Recent developments in the econometrics of program evaluation. J. Econ. Lit. 47:5–86