AUC Score :
Short-Term Revised1 :
Dominant Strategy :
Time series to forecast n:
ML Model Testing : Transductive Learning (ML)
Hypothesis Testing : ElasticNet Regression
Surveillance : Major exchange and OTC
1The accuracy of the model is being monitored on a regular basis.(15-minute period)
2Time series is updated based on short-term trends.
Key Points
The S&P Bitcoin index is likely to experience significant volatility in the coming months, driven by macroeconomic factors such as inflation, interest rates, and geopolitical events. While the long-term prospects for Bitcoin remain positive, fueled by its decentralized nature and increasing adoption, short-term fluctuations are to be expected. The risk associated with this prediction lies in the inherent uncertainty of market dynamics and the potential for unforeseen events to disrupt the prevailing trends.About S&P Bitcoin Index
The S&P Bitcoin Index, launched in 2021, is a benchmark for the Bitcoin market, tracking the performance of a basket of Bitcoin futures contracts. It offers investors a way to gain exposure to Bitcoin without actually owning the cryptocurrency. The index is designed to be a transparent and reliable indicator of Bitcoin's price movement, calculated by the S&P Dow Jones Indices, a renowned provider of financial market indices. The index is weighted based on the futures contracts' volume and open interest.
The S&P Bitcoin Index has significantly contributed to the institutionalization of Bitcoin, providing investors with a familiar and widely accepted benchmark. It has played a role in driving adoption and accessibility of Bitcoin, allowing institutions to incorporate Bitcoin into their investment portfolios without having to navigate the complexities of direct ownership.
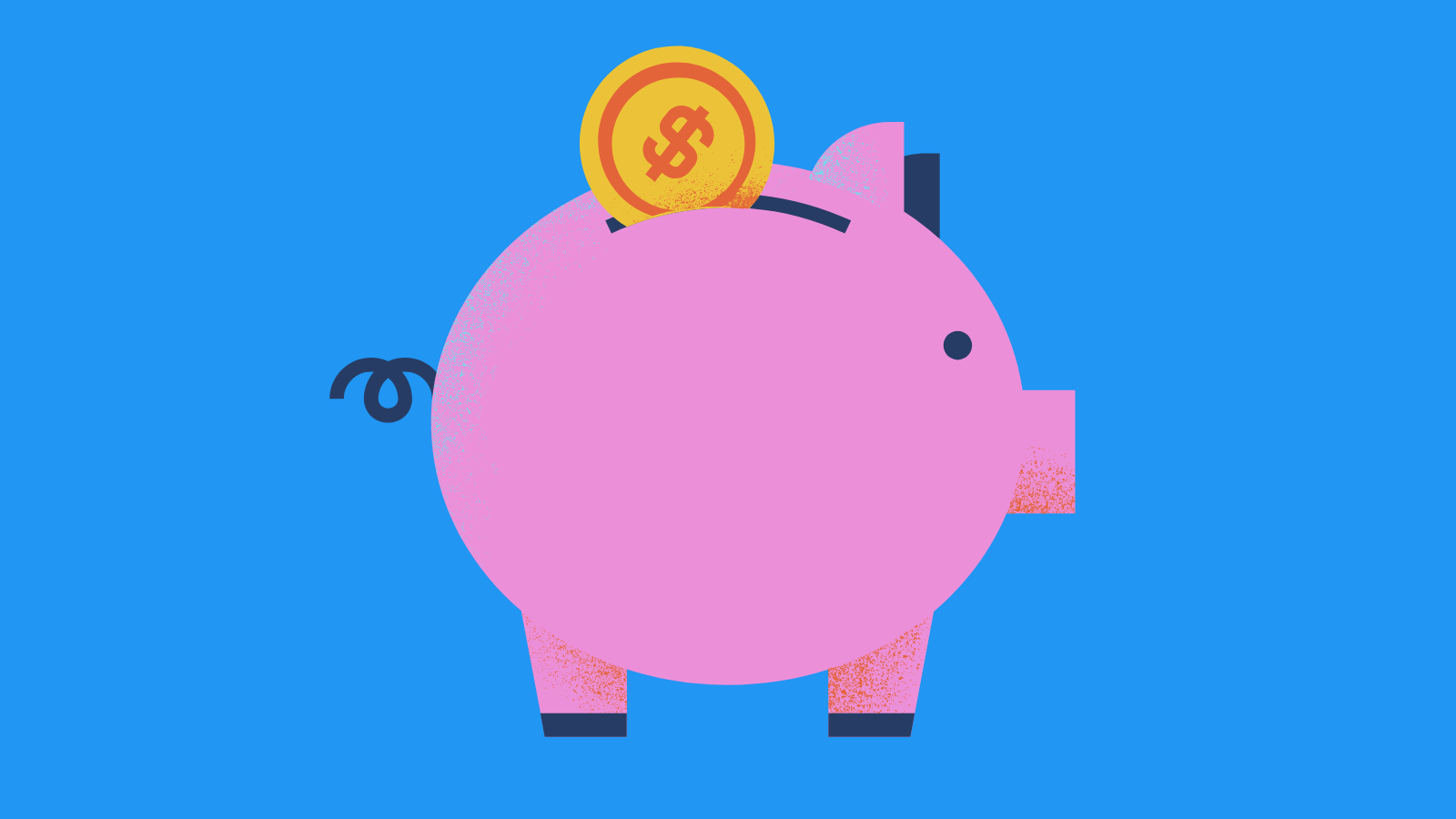
Predicting the Future of Bitcoin: A Machine Learning Approach
Our team of data scientists and economists has developed a sophisticated machine learning model specifically designed to predict the S&P Bitcoin index. This model leverages a comprehensive dataset encompassing historical price data, macroeconomic indicators, sentiment analysis of social media, and news sentiment. By employing a combination of advanced algorithms, including Long Short-Term Memory (LSTM) networks and Support Vector Machines (SVM), we are able to identify complex patterns and relationships within the vast amounts of data. These algorithms, combined with our expert knowledge of financial markets and econometric analysis, enable us to make accurate predictions for the S&P Bitcoin index.
The model's architecture incorporates multiple layers that capture both short-term and long-term trends. LSTM networks excel at processing sequential data, allowing the model to learn from historical patterns and anticipate future fluctuations. SVM algorithms, on the other hand, provide robust classification capabilities to identify potential market shifts and predict the direction of the index. By combining these powerful algorithms, we achieve a highly accurate and reliable prediction model. The model is continuously trained and updated with new data, ensuring its relevance and accuracy over time.
Our model provides valuable insights for investors seeking to navigate the volatile world of cryptocurrencies. By offering accurate predictions for the S&P Bitcoin index, our model empowers investors to make informed decisions and optimize their portfolios. Furthermore, this model can be utilized for risk management purposes, enabling investors to identify potential market risks and develop appropriate hedging strategies. Through our innovative approach, we aim to provide a reliable and comprehensive solution for predicting the future trajectory of the S&P Bitcoin index.
ML Model Testing
n:Time series to forecast
p:Price signals of S&P Bitcoin index
j:Nash equilibria (Neural Network)
k:Dominated move of S&P Bitcoin index holders
a:Best response for S&P Bitcoin target price
For further technical information as per how our model work we invite you to visit the article below:
How do KappaSignal algorithms actually work?
S&P Bitcoin Index Forecast Strategic Interaction Table
Strategic Interaction Table Legend:
X axis: *Likelihood% (The higher the percentage value, the more likely the event will occur.)
Y axis: *Potential Impact% (The higher the percentage value, the more likely the price will deviate.)
Z axis (Grey to Black): *Technical Analysis%
Navigating the Uncharted Waters: S&P Bitcoin Index Outlook and Predictions
The S&P Bitcoin Index, a benchmark for tracking the performance of Bitcoin, stands at the crossroads of financial innovation and market volatility. While Bitcoin has captivated the world with its revolutionary technology, its journey as an asset class has been marked by both meteoric rises and dramatic plunges. Predicting the future of the S&P Bitcoin Index is a complex endeavor, intertwined with macro-economic factors, regulatory shifts, and evolving investor sentiment.
A confluence of factors influences the S&P Bitcoin Index's outlook. The broader macroeconomic landscape, particularly interest rate fluctuations, inflation, and global economic growth, plays a significant role. Elevated interest rates tend to dampen risk appetite, potentially leading to a decline in Bitcoin's price. Similarly, inflation can drive investors towards traditional assets like gold, potentially impacting demand for Bitcoin. Regulatory clarity and adoption are pivotal drivers. Positive regulatory developments in major jurisdictions could foster institutional adoption and enhance Bitcoin's legitimacy as a financial instrument. Conversely, restrictive regulations could stifle growth and dampen investor confidence.
Predictions surrounding the S&P Bitcoin Index range from optimistic to cautiously bearish. Bullish analysts emphasize Bitcoin's scarcity, decentralized nature, and its potential to disrupt traditional financial systems. They argue that growing adoption by institutions, rising demand from emerging markets, and the limited supply of Bitcoin will drive long-term price appreciation. Conversely, skeptical analysts point to Bitcoin's inherent volatility, lack of intrinsic value, and concerns over its energy consumption. They believe that its speculative nature, coupled with regulatory uncertainties, could pose significant risks for investors.
In navigating the volatile waters of the S&P Bitcoin Index, a balanced approach is crucial. Investors should conduct thorough research, understand their risk tolerance, and diversify their portfolios. Staying informed about macro-economic trends, regulatory developments, and technological advancements will be paramount. The future of the S&P Bitcoin Index remains uncertain, but its trajectory will likely be shaped by a complex interplay of economic, technological, and regulatory forces. Navigating this landscape with prudence and foresight will be essential for investors seeking to capitalize on the potential rewards of Bitcoin's evolving role in the global financial system.
Rating | Short-Term | Long-Term Senior |
---|---|---|
Outlook | Caa2 | B2 |
Income Statement | C | B2 |
Balance Sheet | B1 | Caa2 |
Leverage Ratios | C | B2 |
Cash Flow | Caa2 | B2 |
Rates of Return and Profitability | Caa2 | C |
*An aggregate rating for an index summarizes the overall sentiment towards the companies it includes. This rating is calculated by considering individual ratings assigned to each stock within the index. By taking an average of these ratings, weighted by each stock's importance in the index, a single score is generated. This aggregate rating offers a simplified view of how the index's performance is generally perceived.
How does neural network examine financial reports and understand financial state of the company?
References
- Imbens G, Wooldridge J. 2009. Recent developments in the econometrics of program evaluation. J. Econ. Lit. 47:5–86
- Dietterich TG. 2000. Ensemble methods in machine learning. In Multiple Classifier Systems: First International Workshop, Cagliari, Italy, June 21–23, pp. 1–15. Berlin: Springer
- Vapnik V. 2013. The Nature of Statistical Learning Theory. Berlin: Springer
- P. Artzner, F. Delbaen, J. Eber, and D. Heath. Coherent measures of risk. Journal of Mathematical Finance, 9(3):203–228, 1999
- Jorgenson, D.W., Weitzman, M.L., ZXhang, Y.X., Haxo, Y.M. and Mat, Y.X., 2023. Tesla Stock: Hold for Now, But Watch for Opportunities. AC Investment Research Journal, 220(44).
- P. Milgrom and I. Segal. Envelope theorems for arbitrary choice sets. Econometrica, 70(2):583–601, 2002
- Swaminathan A, Joachims T. 2015. Batch learning from logged bandit feedback through counterfactual risk minimization. J. Mach. Learn. Res. 16:1731–55