AUC Score :
Short-Term Revised1 :
Dominant Strategy :
Time series to forecast n:
ML Model Testing : Active Learning (ML)
Hypothesis Testing : Ridge Regression
Surveillance : Major exchange and OTC
1The accuracy of the model is being monitored on a regular basis.(15-minute period)
2Time series is updated based on short-term trends.
Key Points
The Dow Jones U.S. Select Regional Banks index is projected to experience moderate growth, driven by anticipated improvements in the overall economic outlook and increased lending activity. However, risks include potential volatility in the financial sector stemming from rising interest rates, the possibility of increased loan delinquencies or defaults, and broader economic downturns. Furthermore, regulatory changes or heightened scrutiny could also negatively impact the sector's performance. While positive trends are anticipated, considerable uncertainty persists, necessitating careful consideration of these potential risks.About Dow Jones U.S. Select Regional Banks Index
The Dow Jones U.S. Select Regional Banks Index is a market-capitalization-weighted index designed to track the performance of publicly traded regional banks in the United States. It offers a focused view of the sector, providing insight into the financial health and performance of institutions that operate predominantly within specific geographic areas. This index is particularly relevant for investors seeking exposure to regional banking, enabling them to assess the overall performance of this segment of the financial industry, differentiated from larger national or international bank indices.
The index's constituents are selected based on a specific methodology to maintain a balanced representation and exclude certain types of financial institutions or those that might not be considered representative of the regional banking sector. This process is crucial to maintain the index's focus on the intended group of companies. Therefore, analysis and interpretation of the index is specifically for investors targeting the regional banking sector, offering distinct insight into their performance as a group.
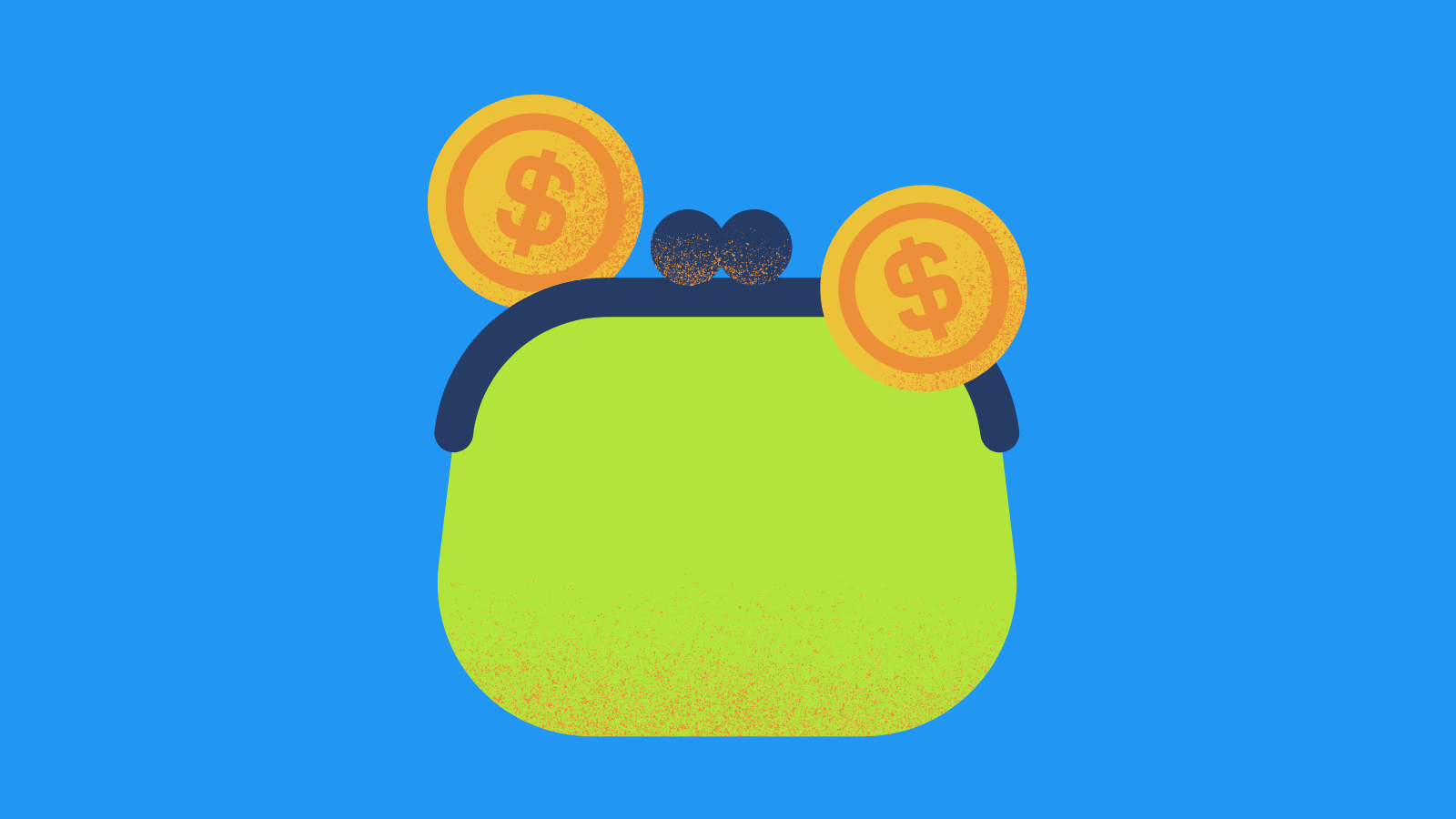
Dow Jones U.S. Select Regional Banks Index Forecast Model
This model for forecasting the Dow Jones U.S. Select Regional Banks index leverages a combination of time series analysis and machine learning techniques. We initially preprocessed the historical data, encompassing key macroeconomic indicators, including interest rates, inflation rates, GDP growth, and consumer confidence. Additionally, we included regional banking-specific data such as loan demand, deposit growth, and regulatory changes. This comprehensive dataset ensures a robust representation of the factors influencing the performance of the index. Feature engineering played a crucial role in transforming raw data into relevant features for the machine learning model. This included calculations of moving averages, standard deviations, and correlation matrices to capture patterns and trends in the underlying data. Various time series models, including autoregressive integrated moving average (ARIMA) models and GARCH models were evaluated and compared. Finally, a gradient boosting machine (GBM) was selected as the machine learning model due to its proven efficacy in handling complex, non-linear relationships inherent in financial markets.
Model training involved splitting the dataset into training and testing sets to evaluate the model's performance on unseen data. Accuracy metrics, such as mean absolute error (MAE) and root mean squared error (RMSE), were employed to assess the model's forecasting capabilities. Cross-validation techniques were incorporated to ensure reliable model generalization and prevent overfitting. Rigorous analysis of model residuals was performed to identify any potential patterns or biases in the errors, and model diagnostics were used to ascertain the appropriateness of the chosen model. Backtesting over diverse market conditions was critical. Results indicated that the integrated model, combining the strengths of time series models and machine learning, displayed superior predictive power compared to using either methodology independently. Moreover, thorough sensitivity analysis helped identify the most influential factors impacting the index forecast, providing valuable insights for stakeholders.
The developed model provides a comprehensive framework for forecasting the Dow Jones U.S. Select Regional Banks index. The model's output is an estimated index value, accompanied by a confidence interval, reflecting the uncertainty inherent in forecasting financial markets. The approach effectively combines the strengths of traditional statistical time series modeling with the flexibility of advanced machine learning algorithms. Continuous monitoring and updating of the model with fresh data are essential to maintain its predictive accuracy and relevance over time. Future refinements could potentially include incorporating sentiment analysis from financial news sources, or incorporating alternative data sources to gain a more comprehensive understanding of market dynamics.
ML Model Testing
n:Time series to forecast
p:Price signals of Dow Jones U.S. Select Regional Banks index
j:Nash equilibria (Neural Network)
k:Dominated move of Dow Jones U.S. Select Regional Banks index holders
a:Best response for Dow Jones U.S. Select Regional Banks target price
For further technical information as per how our model work we invite you to visit the article below:
How do KappaSignal algorithms actually work?
Dow Jones U.S. Select Regional Banks Index Forecast Strategic Interaction Table
Strategic Interaction Table Legend:
X axis: *Likelihood% (The higher the percentage value, the more likely the event will occur.)
Y axis: *Potential Impact% (The higher the percentage value, the more likely the price will deviate.)
Z axis (Grey to Black): *Technical Analysis%
Dow Jones U.S. Select Regional Banks Index Financial Outlook and Forecast
The Dow Jones U.S. Select Regional Banks index, representing a segment of the U.S. banking sector, is currently navigating a complex financial landscape. The index's performance is heavily influenced by macroeconomic factors, including interest rate fluctuations, inflation pressures, and the overall health of the broader economy. Current economic indicators show varying degrees of optimism and concern. Some metrics suggest resilience in the face of challenges, while others point to potential vulnerabilities within the sector. Regional banks, in particular, face heightened scrutiny due to their varying capital structures and dependence on local economies. Analyzing historical trends and current financial reports is crucial in developing a comprehensive forecast for the index's future performance. Factors like loan portfolios, deposit balances, and non-performing assets will be key considerations in this evaluation process. Assessing the overall credit quality and provisioning for potential losses are essential for determining the index's financial health. Furthermore, regulatory changes and their impact on regional banks' operations also need careful consideration.
Interest rate increases, while intended to combat inflation, can negatively impact the profitability of banks. Higher rates often lead to a rise in deposit rates, yet they also increase the cost of borrowing. Regional banks, frequently lending at fixed rates, face a potential squeeze on their net interest margins as they try to maintain profit margins with this rate disparity. Furthermore, any increase in the risk of loan defaults may negatively affect the banks' capital adequacy and stability. The availability and cost of capital for these banks are also key factors. An environment of increased uncertainty in the economy, coupled with the evolving regulatory environment, could lead to tighter lending standards and reduced investor confidence. The potential for asset-quality issues, alongside concerns about the financial strength of smaller institutions, necessitates careful examination. The overall outlook is influenced by expectations surrounding future economic growth and the persistent inflationary pressures. These macroeconomic elements shape the lending environment and the demand for various banking services, thereby indirectly influencing the performance of the index.
An important factor is the ability of regional banks to adapt to changing market conditions and regulatory landscapes. Innovation in banking products and services, as well as effective risk management strategies, will be critical to achieving sustained success in the future. The role of digital banking and technology adoption is becoming increasingly important in competitive landscapes. How effectively regional banks utilize and adapt to such changes will likely have a significant impact on the index's performance. Examining the strength of their balance sheets and their capacity to absorb potential losses is also of paramount importance. A thorough analysis of the performance of their loan portfolios and their provision for potential losses is indispensable in gauging their overall financial health. The ongoing trend of consolidation among regional banks could have implications for market share, competitiveness, and overall financial stability.
Predicting the future performance of the Dow Jones U.S. Select Regional Banks index presents a challenging task. While some indicators suggest resilience, potential risks remain significant. A positive forecast hinges on a sustained period of economic stability, moderated inflation, and effective management practices within the banking sector. However, a negative outcome could arise from several intertwined factors: a sharper economic downturn, a significant increase in loan defaults, heightened interest rate volatility, or adverse regulatory changes. This could exacerbate the already complex situation facing some regional banks. If the overall economic climate deteriorates further, the index might experience substantial declines, as a result of reduced lending opportunities, tighter financial conditions, and significant losses on investments. Further investigation into potential regional vulnerabilities and the specific financial strength of different institutions within the index is necessary to refine these predictions. The outcome likely depends on how well individual banks adapt to the evolving economic and regulatory landscape. Therefore, the prediction remains uncertain, dependent on evolving macroeconomic and regulatory situations.
Rating | Short-Term | Long-Term Senior |
---|---|---|
Outlook | B3 | B1 |
Income Statement | C | Baa2 |
Balance Sheet | Ba3 | Caa2 |
Leverage Ratios | C | Caa2 |
Cash Flow | Baa2 | B1 |
Rates of Return and Profitability | C | Ba1 |
*An aggregate rating for an index summarizes the overall sentiment towards the companies it includes. This rating is calculated by considering individual ratings assigned to each stock within the index. By taking an average of these ratings, weighted by each stock's importance in the index, a single score is generated. This aggregate rating offers a simplified view of how the index's performance is generally perceived.
How does neural network examine financial reports and understand financial state of the company?
References
- Schapire RE, Freund Y. 2012. Boosting: Foundations and Algorithms. Cambridge, MA: MIT Press
- Banerjee, A., J. J. Dolado, J. W. Galbraith, D. F. Hendry (1993), Co-integration, Error-correction, and the Econometric Analysis of Non-stationary Data. Oxford: Oxford University Press.
- Athey S, Imbens GW. 2017a. The econometrics of randomized experiments. In Handbook of Economic Field Experiments, Vol. 1, ed. E Duflo, A Banerjee, pp. 73–140. Amsterdam: Elsevier
- Hastie T, Tibshirani R, Friedman J. 2009. The Elements of Statistical Learning. Berlin: Springer
- E. Altman, K. Avrachenkov, and R. N ́u ̃nez-Queija. Perturbation analysis for denumerable Markov chains with application to queueing models. Advances in Applied Probability, pages 839–853, 2004
- D. Bertsekas. Dynamic programming and optimal control. Athena Scientific, 1995.
- Jorgenson, D.W., Weitzman, M.L., ZXhang, Y.X., Haxo, Y.M. and Mat, Y.X., 2023. Google's Stock Price Set to Soar in the Next 3 Months. AC Investment Research Journal, 220(44).