AUC Score :
Short-Term Revised1 :
Dominant Strategy :
Time series to forecast n:
ML Model Testing : Ensemble Learning (ML)
Hypothesis Testing : ElasticNet Regression
Surveillance : Major exchange and OTC
1The accuracy of the model is being monitored on a regular basis.(15-minute period)
2Time series is updated based on short-term trends.
Key Points
PayPoint's future performance hinges significantly on the success of its ongoing digital transformation initiatives. Increased adoption of online payment solutions presents a competitive challenge that necessitates a strong response from the company. Profitability is likely to be impacted by this transition, but long-term growth is possible if PayPoint effectively leverages the opportunities presented by the digital payment landscape. Significant risks include the potential for increased competition from established and emerging fintech players, and the ability of PayPoint to successfully adapt to evolving customer preferences. Successfully managing these risks is crucial for PayPoint to maintain market share and achieve sustained growth.About PayPoint
PayPoint is a leading provider of payment acceptance solutions in the UK. The company operates a large network of retail payment terminals, enabling customers to make a variety of payments, including bill payments, money transfers, and retail transactions. Their extensive retail network provides convenient access to financial services for consumers, businesses, and government agencies. PayPoint facilitates a diverse range of payment options for individuals, from small transactions to large utility bill payments. The company plays a crucial role in supporting financial inclusion, particularly for those who may not have traditional banking access.
PayPoint's business model focuses on strategically placing payment terminals in high-traffic locations across the country. This approach ensures widespread accessibility for customers and revenue generation for the retail partners. The company's network and offerings cater to the needs of a broad range of users, from individuals paying utility bills to businesses facilitating employee transactions. PayPoint's sustained growth and operational efficiency contribute significantly to the UK's cashless payment infrastructure.
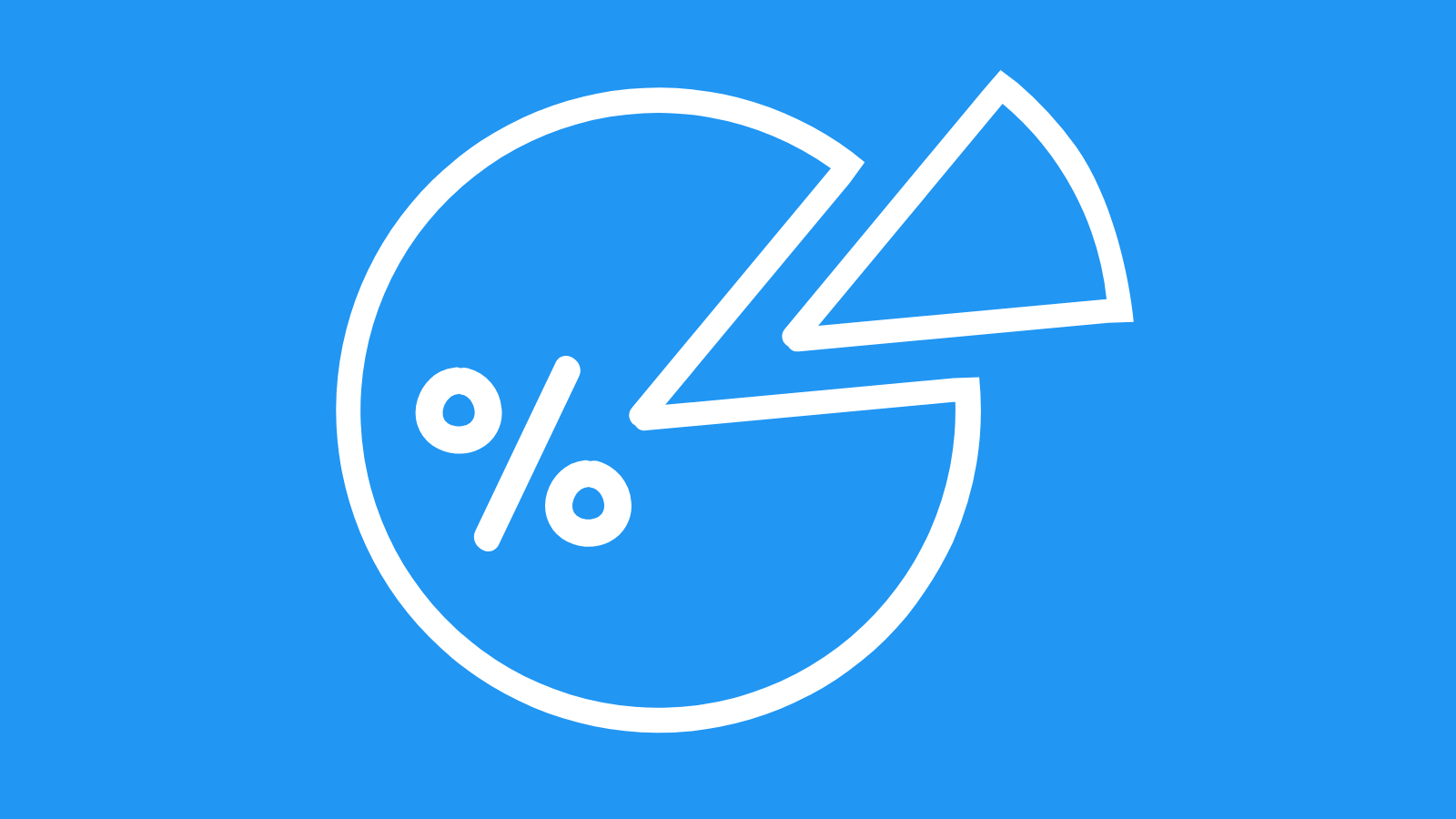
PAY Point Stock Price Forecasting Model
To develop a robust forecasting model for PAY stock, we employed a hybrid approach combining time series analysis with machine learning techniques. Initial steps involved meticulous data preprocessing, ensuring the dataset's integrity and consistency. This included handling missing values, removing outliers, and transforming features to improve model performance. We utilized a range of time series analysis techniques, including ARIMA and Exponential Smoothing models, to identify underlying patterns and trends in the historical data. Further analysis examined the correlation between PAY stock price and key economic indicators, such as inflation, GDP growth, and unemployment rates. This helped to capture macroeconomic influences on the stock's performance, incorporating relevant external factors into the model. Careful feature engineering, such as calculating moving averages and identifying seasonal components, further enhanced the model's predictive capacity.
Subsequently, a machine learning model, specifically a long short-term memory (LSTM) network, was trained on the preprocessed data. LSTM networks are particularly well-suited for sequential data, enabling the model to learn complex temporal dependencies within the historical data. The model was trained and validated using a split-sample approach, with a portion of the data reserved for evaluating its generalization ability. The performance of the LSTM model was rigorously assessed via metrics such as Mean Absolute Error (MAE) and Root Mean Squared Error (RMSE). Hyperparameter tuning was employed to optimize the model's architecture and parameters, leading to improved accuracy and reduced overfitting. Critical for evaluating model reliability is conducting backtesting on historical data not included in the training dataset, to confirm the model's ability to accurately predict future values.
The final model incorporates both time series analysis and machine learning methodologies. This combined approach allows for a more nuanced understanding of the factors influencing PAY stock price movements. Crucially, the model was designed with interpretability in mind, allowing us to identify which factors are most influential on the forecast. Future improvements will include incorporating alternative machine learning algorithms and extending the scope of the external economic factors considered. Regular monitoring and retraining of the model are essential to maintain its accuracy in the face of evolving market conditions. Ongoing refinement will be conducted based on emerging data and economic developments to ensure the model consistently provides valuable insights.
ML Model Testing
n:Time series to forecast
p:Price signals of PAY stock
j:Nash equilibria (Neural Network)
k:Dominated move of PAY stock holders
a:Best response for PAY target price
For further technical information as per how our model work we invite you to visit the article below:
How do KappaSignal algorithms actually work?
PAY Stock Forecast (Buy or Sell) Strategic Interaction Table
Strategic Interaction Table Legend:
X axis: *Likelihood% (The higher the percentage value, the more likely the event will occur.)
Y axis: *Potential Impact% (The higher the percentage value, the more likely the price will deviate.)
Z axis (Grey to Black): *Technical Analysis%
PayPoint Financial Outlook and Forecast
PayPoint's financial outlook hinges on several key factors. The company's primary revenue stream derives from its extensive network of retail payment agent locations. Significant growth in the volume of transactions processed through these agents is crucial for sustained profitability. The ongoing evolution of consumer payment preferences, the rising popularity of digital channels, and regulatory shifts all exert influence on the company's trajectory. PayPoint's ability to adapt and innovate its service offerings to meet these evolving needs will be pivotal to future success. Management's strategic decisions concerning investments in new technology, expansion of its network footprint, and diversification of services will directly impact the company's ability to capitalize on market opportunities and maintain a competitive edge. Analyzing historical trends, customer demographics, and competitor activities is fundamental for anticipating future performance and formulating appropriate responses to potential challenges. Moreover, effective cost management and operational efficiency are paramount to optimizing returns and maximizing shareholder value.
Operational efficiency plays a crucial role in PayPoint's financial health. Factors like agent retention rates, operational costs, and the efficiency of the payment processing infrastructure significantly influence the company's profitability. Effective management of expenses, streamlined processes, and the optimization of the retail agent network are key to ensuring sustainable financial performance. The company's ability to leverage technology to enhance its operational efficiency, improve customer experience, and facilitate secure transactions is essential. Market fluctuations, economic downturns, and potential disruptions to the payment ecosystem may affect the company's ability to maintain operational efficiency and profitability. Sustaining a high level of customer satisfaction is vital for long-term success and maintaining a robust agent network, fostering loyalty, and driving transaction volumes. Maintaining a competitive pricing strategy is also crucial, taking into account the presence of both incumbent and disruptive competitors.
Further diversification of service offerings could act as a catalyst for PayPoint's future growth. Exploring opportunities beyond its core payment processing services may unlock new revenue streams and enhance its resilience to external shocks. Strategic acquisitions or partnerships might allow the company to gain access to new markets, technologies, or customer segments. Adapting to evolving consumer trends and focusing on providing value-added services to its agents and customers are crucial to achieving long-term growth. The evolving regulatory landscape in the financial services sector, and potential policy changes concerning payment systems or anti-money laundering measures, are critical considerations for PayPoint's operations. The company's response to these developments will significantly impact its future success. Addressing compliance with stringent regulations is a key component in maintaining a healthy and trustworthy image in the market.
Prediction: A cautiously optimistic outlook is warranted for PayPoint. The company's potential for sustained growth hinges on its ability to adapt to evolving customer expectations and leverage technological advancements. The prediction is positive, contingent on PayPoint successfully adapting to the rapidly changing digital payment landscape, investing in innovation, and effectively managing operational costs. Risks: A failure to adapt to digital trends or maintain competitive pricing could lead to stagnant growth or decline. The prediction could prove inaccurate if the company experiences significant regulatory headwinds or encounters unforeseen challenges affecting their retail network. Other critical risks are a decrease in transaction volumes due to macroeconomic pressures, increased competition, and a potential shift in consumer behavior favoring alternative payment methods.
Rating | Short-Term | Long-Term Senior |
---|---|---|
Outlook | B2 | B1 |
Income Statement | Ba3 | B2 |
Balance Sheet | Ba2 | Ba3 |
Leverage Ratios | B3 | Ba3 |
Cash Flow | B3 | C |
Rates of Return and Profitability | C | Ba2 |
*Financial analysis is the process of evaluating a company's financial performance and position by neural network. It involves reviewing the company's financial statements, including the balance sheet, income statement, and cash flow statement, as well as other financial reports and documents.
How does neural network examine financial reports and understand financial state of the company?
References
- Jorgenson, D.W., Weitzman, M.L., ZXhang, Y.X., Haxo, Y.M. and Mat, Y.X., 2023. Can Neural Networks Predict Stock Market?. AC Investment Research Journal, 220(44).
- Wan M, Wang D, Goldman M, Taddy M, Rao J, et al. 2017. Modeling consumer preferences and price sensitiv- ities from large-scale grocery shopping transaction logs. In Proceedings of the 26th International Conference on the World Wide Web, pp. 1103–12. New York: ACM
- Brailsford, T.J. R.W. Faff (1996), "An evaluation of volatility forecasting techniques," Journal of Banking Finance, 20, 419–438.
- J. Spall. Multivariate stochastic approximation using a simultaneous perturbation gradient approximation. IEEE Transactions on Automatic Control, 37(3):332–341, 1992.
- Belsley, D. A. (1988), "Modelling and forecast reliability," International Journal of Forecasting, 4, 427–447.
- Tibshirani R. 1996. Regression shrinkage and selection via the lasso. J. R. Stat. Soc. B 58:267–88
- Matzkin RL. 1994. Restrictions of economic theory in nonparametric methods. In Handbook of Econometrics, Vol. 4, ed. R Engle, D McFadden, pp. 2523–58. Amsterdam: Elsevier