AUC Score :
Short-Term Revised1 :
Dominant Strategy :
Time series to forecast n:
ML Model Testing : Modular Neural Network (CNN Layer)
Hypothesis Testing : Linear Regression
Surveillance : Major exchange and OTC
1The accuracy of the model is being monitored on a regular basis.(15-minute period)
2Time series is updated based on short-term trends.
Key Points
The OMXS30 index is anticipated to experience moderate volatility, with potential for both gains and losses. A sustained period of positive economic indicators, coupled with investor confidence, could drive upward momentum. Conversely, global economic uncertainty or unforeseen regional events could negatively impact the index, leading to periods of decline. The risk associated with these predictions hinges on the accuracy of economic forecasts and the stability of geopolitical conditions. Unforeseen catalysts could significantly alter the trajectory of the index.About OMXS30 Index
The OMXS30 is a stock market index that tracks the performance of the 30 largest and most actively traded companies listed on the Stockholm Stock Exchange. It represents a significant portion of the Swedish equity market and serves as a crucial benchmark for investors and analysts. The index is calculated using a free-float capitalization weighted method, which means that the weighting of each constituent stock reflects its proportional ownership by the public. This provides a comprehensive representation of the market's overall direction.
The index's composition is dynamic, with companies being added or removed based on various criteria, reflecting changes in market leadership and the evolving economy. Historical performance of the OMXS30 index has shown fluctuations, mirroring general economic trends and market sentiment. The index is a key indicator for assessing the health and trajectory of the Swedish economy and is frequently used for financial modeling and investment decision-making within the region.
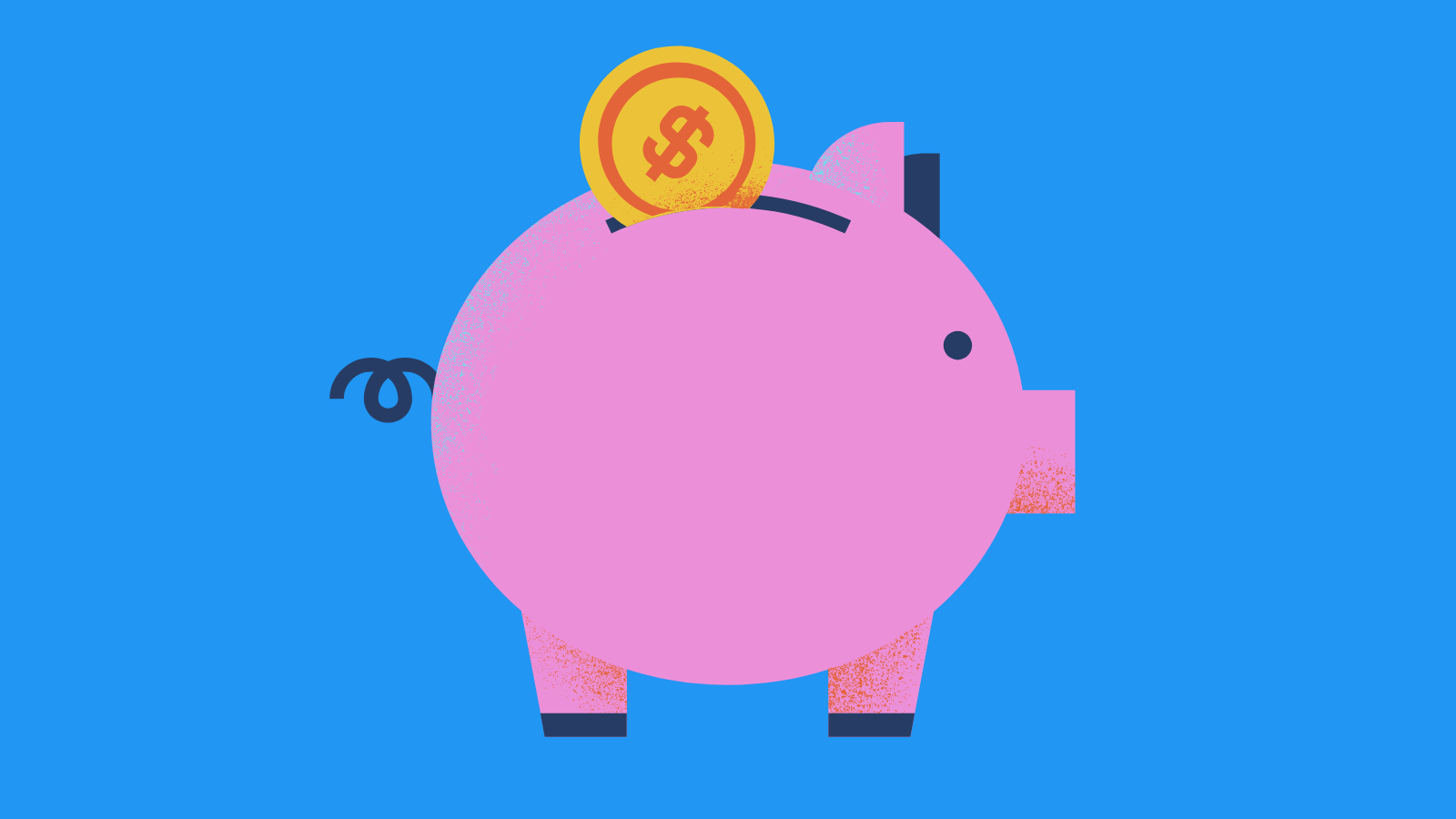
OMXS30 Index Forecasting Model
This model employs a hybrid approach combining time series analysis with machine learning techniques to forecast the OMXS30 index. We leverage historical data encompassing various economic indicators, including GDP growth, inflation rates, interest rates, and key corporate earnings reports. The initial phase involves meticulous data preprocessing, addressing potential issues such as missing values and outliers. This ensures the integrity and reliability of the input data for the subsequent model training. A key component of our methodology involves feature engineering, creating new variables from existing data to capture complex relationships. For instance, we will construct indicators of investor sentiment derived from news sentiment analysis and social media data. The choice of machine learning algorithms is contingent upon the specific characteristics of the dataset and the desired forecast horizon. We will potentially employ a combination of recurrent neural networks (RNNs), specifically LSTMs, to capture temporal dependencies within the data and support long-term forecasting, alongside classical statistical models for a comprehensive approach.
The model training phase will involve splitting the historical dataset into training and testing sets. The selection of appropriate hyperparameters for the chosen algorithms will be optimized using techniques such as grid search or Bayesian optimization. Model evaluation will be rigorous, employing metrics such as Mean Absolute Error (MAE) and Root Mean Squared Error (RMSE) to assess the accuracy and robustness of the forecasting performance. Extensive backtesting will be conducted on the hold-out test set to further validate the model's performance under different market conditions. This comprehensive testing process ensures the model's generalizability and reliability in projecting future index movements. The incorporation of external factors like geopolitical events and global market trends into the model will also be considered and potentially included in future iterations, enhancing model predictive accuracy.
Following the validation stage, the model will be deployed for real-time forecasting. A crucial aspect of the deployment process involves continuously monitoring the model's performance and retraining it periodically with updated data to maintain its predictive accuracy and adaptability. This continual refinement process ensures that the model remains relevant and responsive to the dynamic nature of the OMXS30 index and the associated economic factors. This proactive approach to model maintenance is essential for ensuring the reliability and effectiveness of the forecasts for various stakeholders, including investors, policymakers, and financial institutions.
ML Model Testing
n:Time series to forecast
p:Price signals of OMXS30 index
j:Nash equilibria (Neural Network)
k:Dominated move of OMXS30 index holders
a:Best response for OMXS30 target price
For further technical information as per how our model work we invite you to visit the article below:
How do KappaSignal algorithms actually work?
OMXS30 Index Forecast Strategic Interaction Table
Strategic Interaction Table Legend:
X axis: *Likelihood% (The higher the percentage value, the more likely the event will occur.)
Y axis: *Potential Impact% (The higher the percentage value, the more likely the price will deviate.)
Z axis (Grey to Black): *Technical Analysis%
OMXS30 Index Financial Outlook and Forecast
The OMXS30, a benchmark index representing the 30 largest and most liquid companies listed on the Stockholm Stock Exchange, currently exhibits a complex financial outlook. Several key factors influence its trajectory. Recent macroeconomic conditions, including global inflation, rising interest rates, and geopolitical uncertainties, pose significant headwinds to overall market performance. These external pressures are impacting profitability projections for companies within the index, which are diversified across sectors, including technology, financials, and industrials. Market analysts are keenly observing the sector-specific performance of these companies to assess their resilience and adaptability in response to the prevailing economic climate. The ongoing evaluation of earnings reports and projections provides valuable insights into the current and future financial health of the constituent companies.
Furthermore, anticipated changes in monetary policy by central banks globally are a crucial determinant of future market trends. The potential for further interest rate hikes aimed at curbing inflation carries the risk of dampening economic growth and potentially triggering a slowdown. This dynamic environment necessitates careful consideration of the overall economic picture, in which interest rate hikes may lead to diminished consumer spending and reduced investment activity. These trends will likely affect the performance of specific companies within the OMXS30, particularly those heavily reliant on external financing or with high levels of debt. The market is closely watching whether interest rate adjustments effectively curb inflation without excessively impacting economic activity. Furthermore, developments in the European Union, a major trading partner for many companies in the index, are also important considerations for future forecasting.
Despite the aforementioned challenges, some pockets of potential growth and resilience exist within the OMXS30. Specific sectors, particularly those with exposure to technological advancements or sustainable practices, may experience sustained growth. Innovation and technological advancements offer potential for increased productivity and profitability. The global transition to greener technologies could also generate opportunities for companies within the index that are invested in those areas. The performance of these sectors, in tandem with company-specific strategies for navigating economic headwinds, plays a pivotal role in shaping the overall outlook for the index. Companies demonstrating sound financial management, efficient operational strategies, and strong leadership often prove more resilient during periods of market uncertainty.
The overall prediction for the OMXS30 index is cautiously optimistic, but with significant caveats. A positive outlook is predicated on companies within the index effectively managing costs, navigating macroeconomic volatility, and capitalizing on growth opportunities. Risks to this prediction include a deeper economic downturn than anticipated, prolonged periods of high inflation, or unforeseen geopolitical events. The recent rise in energy prices and supply chain disruptions also present material risks. Ultimately, the success of the OMXS30 will depend on the collective ability of its constituent companies to adapt to evolving market conditions and seize any growth opportunities arising from those changes. The sustained performance of the global economy and how well OMXS30 companies adapt to the shifting economic landscape will determine whether the predicted positive trajectory is achieved.
Rating | Short-Term | Long-Term Senior |
---|---|---|
Outlook | B2 | B3 |
Income Statement | Baa2 | C |
Balance Sheet | Baa2 | Caa2 |
Leverage Ratios | C | Baa2 |
Cash Flow | C | C |
Rates of Return and Profitability | B3 | Caa2 |
*An aggregate rating for an index summarizes the overall sentiment towards the companies it includes. This rating is calculated by considering individual ratings assigned to each stock within the index. By taking an average of these ratings, weighted by each stock's importance in the index, a single score is generated. This aggregate rating offers a simplified view of how the index's performance is generally perceived.
How does neural network examine financial reports and understand financial state of the company?
References
- Arjovsky M, Bottou L. 2017. Towards principled methods for training generative adversarial networks. arXiv:1701.04862 [stat.ML]
- M. L. Littman. Markov games as a framework for multi-agent reinforcement learning. In Ma- chine Learning, Proceedings of the Eleventh International Conference, Rutgers University, New Brunswick, NJ, USA, July 10-13, 1994, pages 157–163, 1994
- J. Z. Leibo, V. Zambaldi, M. Lanctot, J. Marecki, and T. Graepel. Multi-agent Reinforcement Learning in Sequential Social Dilemmas. In Proceedings of the 16th International Conference on Autonomous Agents and Multiagent Systems (AAMAS 2017), Sao Paulo, Brazil, 2017
- Angrist JD, Pischke JS. 2008. Mostly Harmless Econometrics: An Empiricist's Companion. Princeton, NJ: Princeton Univ. Press
- Byron, R. P. O. Ashenfelter (1995), "Predicting the quality of an unborn grange," Economic Record, 71, 40–53.
- H. Kushner and G. Yin. Stochastic approximation algorithms and applications. Springer, 1997.
- E. Collins. Using Markov decision processes to optimize a nonlinear functional of the final distribution, with manufacturing applications. In Stochastic Modelling in Innovative Manufacturing, pages 30–45. Springer, 1997