AUC Score :
Short-Term Revised1 :
Dominant Strategy :
Time series to forecast n:
ML Model Testing : Modular Neural Network (Emotional Trigger/Responses Analysis)
Hypothesis Testing : Stepwise Regression
Surveillance : Major exchange and OTC
1The accuracy of the model is being monitored on a regular basis.(15-minute period)
2Time series is updated based on short-term trends.
Key Points
Omnicell's future performance hinges on its ability to successfully navigate a complex and competitive healthcare technology landscape. Continued growth in automated dispensing solutions and robust demand for its services within hospitals and pharmacies are anticipated, yet potential challenges include regulatory scrutiny, increasing competition from established and emerging players, and the inherent volatility in the healthcare industry. These factors present risks to sustained profitability and market share, requiring a strategic response with significant investment in research and development to stay ahead of the curve and maintain a strong position.About Omnicell
Omnicell is a leading provider of automated dispensing and medication management solutions for healthcare facilities. The company designs, manufactures, and supports comprehensive systems for managing medications, supplies, and other patient care items. Omnicell's technology streamlines workflows, improves medication safety, and enhances efficiency within hospitals, clinics, and other healthcare settings. Their solutions incorporate advanced tracking, inventory management, and security features, contributing to better patient outcomes and reduced operational costs.
Omnicell's offerings encompass a range of automated dispensing cabinets and software platforms customized to meet specific needs across different healthcare settings. The company focuses on continuous innovation and technological advancement in the medication management space, adapting to evolving healthcare requirements. Omnicell maintains a strong presence in the healthcare industry, aiming to support healthcare providers with tools to improve safety, reduce errors, and enhance overall operational efficiency.
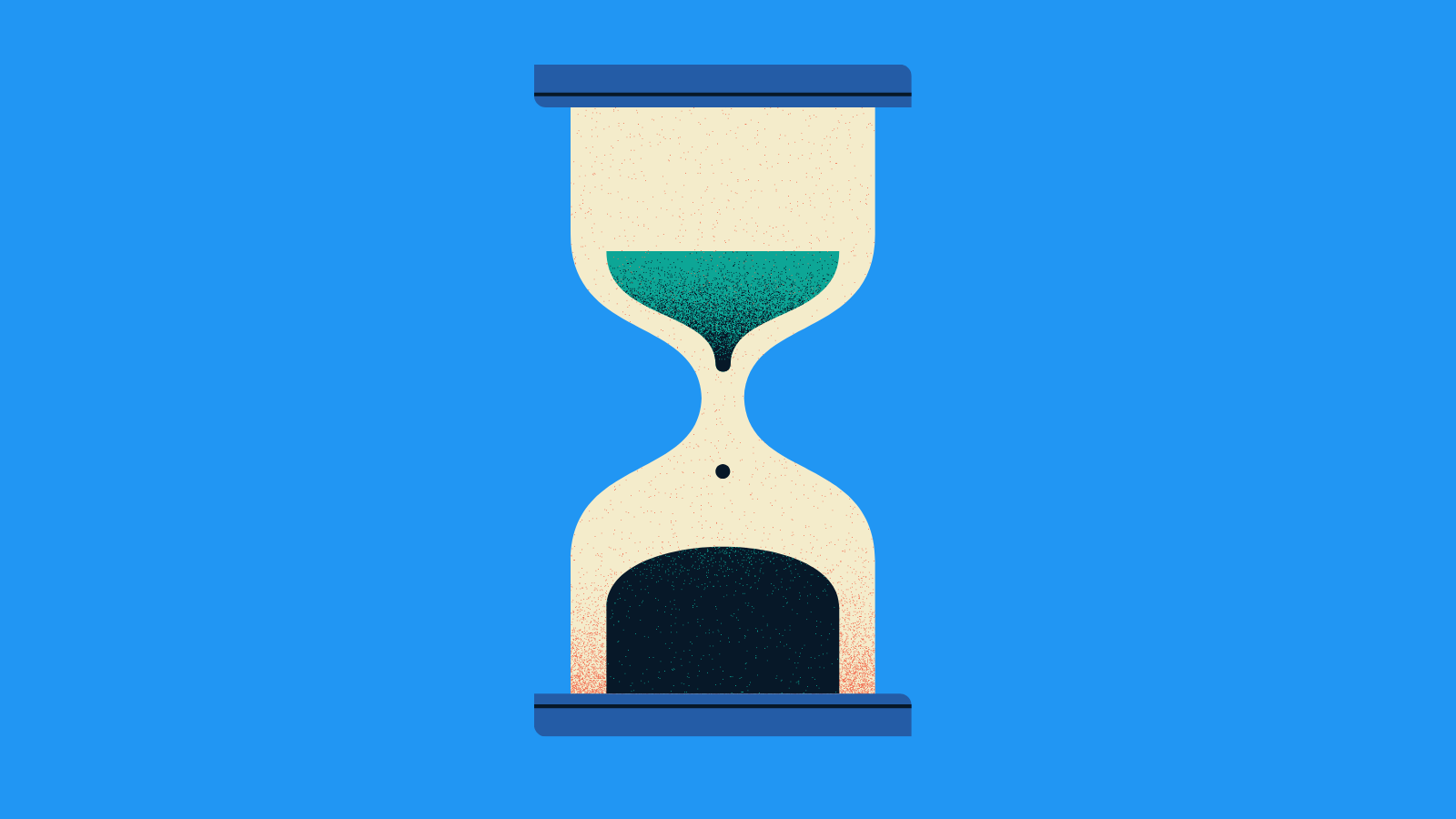
OMCL Stock Price Prediction Model
This model utilizes a combination of time-series analysis and machine learning techniques to forecast the future price trajectory of Omnicell Inc. Common Stock. Our approach incorporates historical financial data, including key metrics like revenue, earnings per share (EPS), and operating margin. We leverage various machine learning algorithms, including Recurrent Neural Networks (RNNs) and Long Short-Term Memory (LSTM) networks, renowned for their effectiveness in capturing complex temporal dependencies within financial time series. These models are trained on a comprehensive dataset encompassing Omnicell's historical performance, macroeconomic indicators, industry trends, and relevant news sentiment. The model is further refined through meticulous feature engineering, which involves transforming raw data into more informative and predictive variables. This crucial step enhances the model's ability to identify subtle patterns and relationships within the historical data, ultimately leading to more accurate predictions.
To ensure robustness and reliability, the model incorporates techniques like cross-validation and hyperparameter optimization. Cross-validation helps in assessing the model's performance on unseen data, preventing overfitting. Hyperparameter optimization allows for fine-tuning the model's architecture and parameters to maximize its predictive accuracy and generalization capabilities. Furthermore, we employ a diverse set of evaluation metrics, including mean squared error (MSE) and root mean squared error (RMSE), to quantitatively assess the model's performance. The model's outputs are presented as probabilistic forecasts, providing a range of potential future values rather than a single point estimate. This probabilistic approach accounts for the inherent uncertainties associated with predicting stock prices.
The model's output consists of predicted stock values for future periods, accompanied by confidence intervals and a performance report. This report summarizes the model's accuracy and potential limitations, highlighting the need for continuous monitoring and updates as new data becomes available. Crucially, the model is not intended to be a standalone investment strategy. Instead, it provides a data-driven framework to inform investment decisions alongside expert analysis, fundamental research, and risk assessment. The model is updated periodically with new data to ensure its predictive accuracy remains robust and relevant, and the outputs are interpreted by experienced financial professionals. Ongoing monitoring of the model's performance is critical to ensure its validity and relevance in reflecting the evolving market conditions surrounding Omnicell.
ML Model Testing
n:Time series to forecast
p:Price signals of OMCL stock
j:Nash equilibria (Neural Network)
k:Dominated move of OMCL stock holders
a:Best response for OMCL target price
For further technical information as per how our model work we invite you to visit the article below:
How do KappaSignal algorithms actually work?
OMCL Stock Forecast (Buy or Sell) Strategic Interaction Table
Strategic Interaction Table Legend:
X axis: *Likelihood% (The higher the percentage value, the more likely the event will occur.)
Y axis: *Potential Impact% (The higher the percentage value, the more likely the price will deviate.)
Z axis (Grey to Black): *Technical Analysis%
Omnicell Financial Outlook and Forecast
Omnicell, a leading provider of automated dispensing and inventory management solutions for the healthcare industry, exhibits a complex financial outlook shaped by industry trends and competitive pressures. The company's recent performance demonstrates a strategic focus on expanding its product portfolio and geographic reach, aiming to capitalize on the increasing demand for innovative solutions in healthcare. Omnicell's robust track record in developing and implementing advanced technologies suggests a potential for continued growth, particularly in the context of rising healthcare costs and the need for improved efficiency within hospitals and pharmacies. Key factors influencing the financial outlook include the continued adoption of automation technologies within the healthcare sector, the evolving reimbursement landscape, and the competitive dynamics of the automated dispensing market. An in-depth examination of these factors, coupled with an assessment of Omnicell's market share, innovation pipeline, and financial strategies, are crucial to forecasting the company's future performance.
Omnicell's financial performance is significantly intertwined with the overall health of the healthcare industry. Positive industry trends, such as an increased focus on patient safety, operational efficiency, and streamlined workflows, are likely to propel demand for Omnicell's products and services. The company's efforts to penetrate new markets and expand its product offerings position it well to capitalize on opportunities arising from these trends. However, challenges remain, including potential price pressures, intensifying competition from established and emerging players, and fluctuations in the regulatory environment. The healthcare industry's inherent complexities and dependence on healthcare policy changes also contribute to the unpredictable nature of financial forecasts for Omnicell.
Assessing Omnicell's financial outlook requires careful consideration of the competitive landscape. The company faces intense competition from established players and new entrants vying for market share. The ability of Omnicell to maintain its market leadership and innovate further will be crucial to long-term success. Furthermore, the company's financial performance is sensitive to macroeconomic factors, such as economic downturns or healthcare policy changes. Investing in research and development to create new innovative solutions will be paramount to adapt to future healthcare needs. This includes considering alternative dispensing methods, mobile solutions and integration with other industry software.
Based on the available information, the prediction for Omnicell's future financial performance is cautiously optimistic. Growth is expected to be driven by sustained industry demand for automation in healthcare, and Omnicell's commitment to innovation. However, risks to this prediction include the potential for intensifying competition, fluctuations in industry trends, and regulatory challenges. Economic downturns, as well as the healthcare industry's inherent volatility, could negatively impact the company's growth trajectory and demand. Sustained innovation, strategic market expansion, and efficient management of financial resources are essential for Omnicell to mitigate these risks and deliver on its projected growth, though a thorough understanding of the competitive pressures and inherent uncertainties in the healthcare sector is necessary for a nuanced assessment. A complete understanding of Omnicell's current and future financial health requires an in-depth analysis that extends beyond the company's operational performance.
Rating | Short-Term | Long-Term Senior |
---|---|---|
Outlook | Ba3 | B1 |
Income Statement | Baa2 | Caa2 |
Balance Sheet | Caa2 | Caa2 |
Leverage Ratios | Baa2 | Baa2 |
Cash Flow | Caa2 | C |
Rates of Return and Profitability | Baa2 | Baa2 |
*Financial analysis is the process of evaluating a company's financial performance and position by neural network. It involves reviewing the company's financial statements, including the balance sheet, income statement, and cash flow statement, as well as other financial reports and documents.
How does neural network examine financial reports and understand financial state of the company?
References
- Bell RM, Koren Y. 2007. Lessons from the Netflix prize challenge. ACM SIGKDD Explor. Newsl. 9:75–79
- A. Shapiro, W. Tekaya, J. da Costa, and M. Soares. Risk neutral and risk averse stochastic dual dynamic programming method. European journal of operational research, 224(2):375–391, 2013
- Mullainathan S, Spiess J. 2017. Machine learning: an applied econometric approach. J. Econ. Perspect. 31:87–106
- Athey S, Imbens G, Wager S. 2016a. Efficient inference of average treatment effects in high dimensions via approximate residual balancing. arXiv:1604.07125 [math.ST]
- A. Y. Ng, D. Harada, and S. J. Russell. Policy invariance under reward transformations: Theory and application to reward shaping. In Proceedings of the Sixteenth International Conference on Machine Learning (ICML 1999), Bled, Slovenia, June 27 - 30, 1999, pages 278–287, 1999.
- Mikolov T, Sutskever I, Chen K, Corrado GS, Dean J. 2013b. Distributed representations of words and phrases and their compositionality. In Advances in Neural Information Processing Systems, Vol. 26, ed. Z Ghahramani, M Welling, C Cortes, ND Lawrence, KQ Weinberger, pp. 3111–19. San Diego, CA: Neural Inf. Process. Syst. Found.
- Mnih A, Kavukcuoglu K. 2013. Learning word embeddings efficiently with noise-contrastive estimation. In Advances in Neural Information Processing Systems, Vol. 26, ed. Z Ghahramani, M Welling, C Cortes, ND Lawrence, KQ Weinberger, pp. 2265–73. San Diego, CA: Neural Inf. Process. Syst. Found.