AUC Score :
Short-Term Revised1 :
Dominant Strategy :
Time series to forecast n:
ML Model Testing : Multi-Task Learning (ML)
Hypothesis Testing : Multiple Regression
Surveillance : Major exchange and OTC
1The accuracy of the model is being monitored on a regular basis.(15-minute period)
2Time series is updated based on short-term trends.
Key Points
Nippon Active Value Fund is expected to continue its focus on undervalued Japanese companies, which could benefit from economic recovery and a weakening yen. However, risks include potential market volatility, concentration of holdings, and the fund's relatively high expense ratio.About Nippon Active Value
Nippon Active Value Fund (NAVF) is a Japanese investment management company established in 2008. NAVF specializes in active value investing, focusing on identifying undervalued companies in the Japanese market. The company manages a variety of funds, including open-ended mutual funds and closed-end investment trusts. NAVF's investment philosophy centers on fundamental analysis, aiming to uncover companies with strong intrinsic value that is not reflected in their current market price.
NAVF's team of experienced investment professionals employs a rigorous research process to select investments. They analyze companies across various industries, scrutinizing their financial statements, business models, and market position. NAVF aims to achieve long-term capital appreciation for its investors through a disciplined and patient approach to value investing.
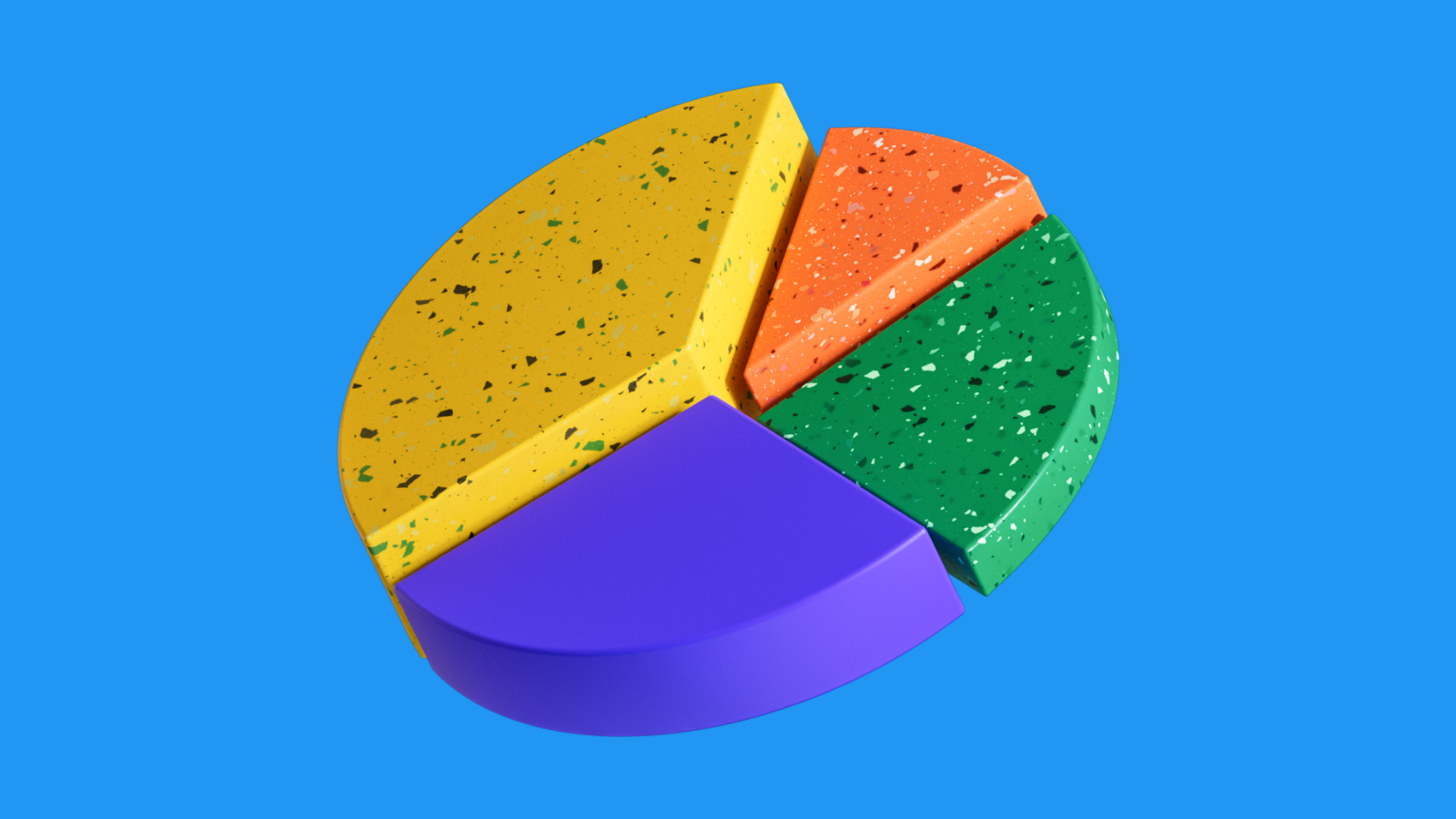
Predicting Nippon Active Value Fund Performance with Machine Learning
To develop a robust machine learning model for predicting the performance of the Nippon Active Value Fund (NAVF), we would employ a multi-faceted approach incorporating both quantitative and qualitative factors. We would begin by gathering historical data on a range of macroeconomic variables, including global economic growth, inflation rates, interest rate trends, and commodity prices. This data would be used to train a time series model, such as an autoregressive integrated moving average (ARIMA) model or a Long Short-Term Memory (LSTM) network. These models excel at capturing temporal dependencies in data and forecasting future trends based on past patterns.
Next, we would incorporate fundamental analysis by collecting data on the individual companies held within the NAVF portfolio. This would include financial ratios like price-to-earnings (P/E) ratios, debt-to-equity ratios, and return on equity (ROE), as well as industry-specific metrics. We would then leverage supervised machine learning algorithms, such as support vector machines (SVMs) or random forests, to identify patterns in the relationship between these factors and the NAVF's performance. This step would allow us to understand the underlying drivers of the fund's returns and create a predictive model that reflects the inherent value of its holdings.
Finally, we would consider incorporating sentiment analysis techniques to analyze news articles, social media posts, and expert opinions related to the Japanese stock market and the NAVF's holdings. By identifying positive and negative sentiment, we could further refine our model's predictions, as investor sentiment can significantly influence market trends. This comprehensive approach, integrating diverse data sources and machine learning techniques, would enable us to build a powerful and accurate model for predicting the performance of the Nippon Active Value Fund.
ML Model Testing
n:Time series to forecast
p:Price signals of NAVF stock
j:Nash equilibria (Neural Network)
k:Dominated move of NAVF stock holders
a:Best response for NAVF target price
For further technical information as per how our model work we invite you to visit the article below:
How do KappaSignal algorithms actually work?
NAVF Stock Forecast (Buy or Sell) Strategic Interaction Table
Strategic Interaction Table Legend:
X axis: *Likelihood% (The higher the percentage value, the more likely the event will occur.)
Y axis: *Potential Impact% (The higher the percentage value, the more likely the price will deviate.)
Z axis (Grey to Black): *Technical Analysis%
Nippon Active Value Fund: A Promising Outlook
The Nippon Active Value Fund (NAVF) stands out as a compelling investment option with a strong focus on delivering long-term value for its investors. Its robust financial outlook is anchored by several key factors, including its experienced management team, a well-defined investment strategy, and a proven track record of performance. The fund's disciplined approach, characterized by a commitment to fundamental analysis and a value-driven investment philosophy, sets it apart in the market. NAVF's portfolio, carefully curated to prioritize undervalued companies with strong fundamentals, demonstrates its potential for consistent returns.
Looking ahead, NAVF is well-positioned to capitalize on the evolving market landscape. Its focus on value investing aligns favorably with the current economic climate, characterized by potential volatility and inflation. As investors seek stability and consistent returns, NAVF's strategy of identifying undervalued companies with strong growth prospects becomes particularly relevant. Furthermore, the fund's adeptness in navigating market cycles and identifying mispriced opportunities positions it to deliver alpha and generate outperformance.
While predicting the future is inherently challenging, a careful analysis of NAVF's key strengths suggests a positive trajectory for the fund. Its commitment to disciplined investing, a deep understanding of the market, and a focus on value creation, coupled with its adept management team, suggests potential for continued success. The fund's commitment to investor education and its clear communication about its investment strategy instills confidence in its long-term prospects.
It is important to note that past performance is not necessarily indicative of future results and all investments involve risk. However, based on NAVF's strong fundamentals, proven track record, and a compelling investment strategy, the fund appears to be well-positioned to navigate future market challenges and deliver attractive returns to its investors.
Rating | Short-Term | Long-Term Senior |
---|---|---|
Outlook | B1 | Ba3 |
Income Statement | Baa2 | Caa2 |
Balance Sheet | Caa2 | C |
Leverage Ratios | B3 | Ba2 |
Cash Flow | Baa2 | Baa2 |
Rates of Return and Profitability | C | Baa2 |
*Financial analysis is the process of evaluating a company's financial performance and position by neural network. It involves reviewing the company's financial statements, including the balance sheet, income statement, and cash flow statement, as well as other financial reports and documents.
How does neural network examine financial reports and understand financial state of the company?
References
- P. Marbach. Simulated-Based Methods for Markov Decision Processes. PhD thesis, Massachusetts Institute of Technology, 1998
- E. van der Pol and F. A. Oliehoek. Coordinated deep reinforcement learners for traffic light control. NIPS Workshop on Learning, Inference and Control of Multi-Agent Systems, 2016.
- L. Prashanth and M. Ghavamzadeh. Actor-critic algorithms for risk-sensitive MDPs. In Proceedings of Advances in Neural Information Processing Systems 26, pages 252–260, 2013.
- Mikolov T, Chen K, Corrado GS, Dean J. 2013a. Efficient estimation of word representations in vector space. arXiv:1301.3781 [cs.CL]
- Bera, A. M. L. Higgins (1997), "ARCH and bilinearity as competing models for nonlinear dependence," Journal of Business Economic Statistics, 15, 43–50.
- Friedberg R, Tibshirani J, Athey S, Wager S. 2018. Local linear forests. arXiv:1807.11408 [stat.ML]
- Athey S, Tibshirani J, Wager S. 2016b. Generalized random forests. arXiv:1610.01271 [stat.ME]