AUC Score :
Short-Term Revised1 :
Dominant Strategy :
Time series to forecast n:
ML Model Testing : Reinforcement Machine Learning (ML)
Hypothesis Testing : Chi-Square
Surveillance : Major exchange and OTC
1The accuracy of the model is being monitored on a regular basis.(15-minute period)
2Time series is updated based on short-term trends.
Key Points
Mondelez is expected to benefit from continued strong demand for its snacks and beverages, particularly in emerging markets. The company's focus on innovation and brand building should drive revenue growth. However, Mondelez faces risks related to rising input costs, global economic uncertainty, and competition. The company's reliance on emerging markets could also expose it to political and economic instability. Overall, Mondelez is well-positioned for long-term growth, but investors should be aware of the potential risks.About Mondelez International
Mondelez International, Inc. (MDLZ) is a multinational confectionery, food, and beverage company. The company was formed in 2012 after Kraft Foods was split into two separate companies. Mondelez is headquartered in Illinois, USA. It is one of the largest food and beverage companies in the world with over 100,000 employees and operations in over 160 countries. Mondelez is known for its iconic brands such as Oreo, Cadbury, Trident, and Milka. Its portfolio includes a wide range of food and beverage products, including chocolate, biscuits, gum, candy, coffee, and powdered beverages.
Mondelez International is focused on growth through innovation and expansion into emerging markets. It aims to deliver high-quality products that meet consumer needs around the world. The company has a strong commitment to sustainability and social responsibility. Mondelez International is committed to creating a more sustainable food system, reducing its environmental impact, and supporting communities around the world.
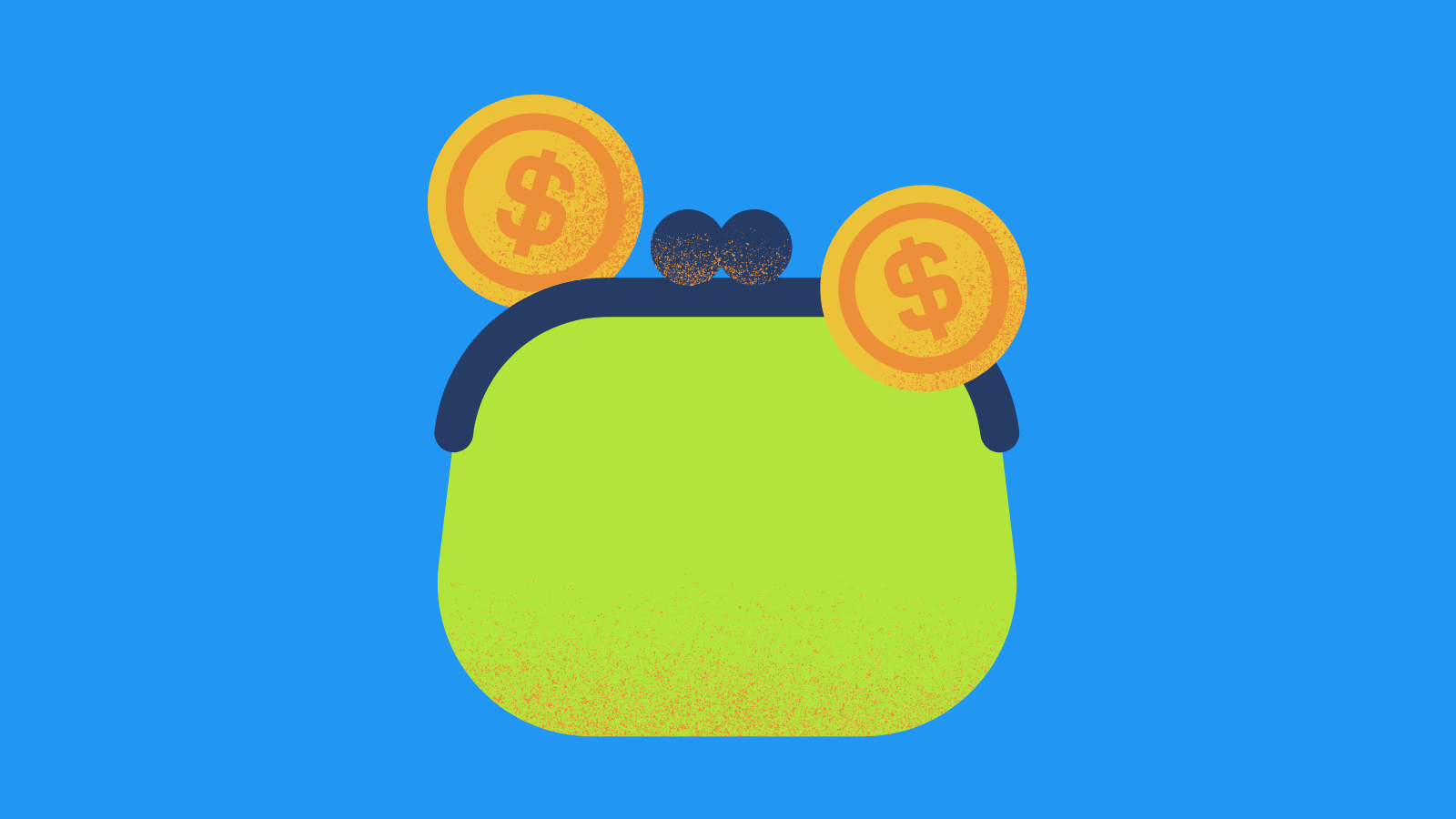
Predicting the Future of Mondelez International: A Machine Learning Approach
Our team of data scientists and economists has developed a sophisticated machine learning model to predict the future price movements of Mondelez International Inc. Class A Common Stock (MDLZ). Our model utilizes a comprehensive dataset encompassing historical stock prices, financial reports, macroeconomic indicators, consumer sentiment data, and industry-specific trends. Employing advanced algorithms like Long Short-Term Memory (LSTM) networks, we identify patterns and relationships within the data to forecast future price fluctuations.
The model incorporates a multi-layered approach to account for diverse factors influencing MDLZ stock. The first layer analyzes historical stock price trends and volume, capturing short-term and long-term market sentiment. Next, the model integrates financial data, analyzing key performance indicators like revenue growth, profit margins, and debt levels to gauge the company's financial health. Finally, external economic factors like interest rates, inflation, and consumer confidence are incorporated to assess broader market conditions impacting the snack food industry.
By combining this rich dataset with powerful machine learning algorithms, our model generates probabilistic forecasts for MDLZ stock price movements. These predictions are regularly updated and refined as new data becomes available, providing a dynamic and data-driven approach to understanding future market behavior. The model's outputs can assist investors in making informed decisions regarding portfolio allocation and trading strategies, leveraging the power of data science to navigate the complexities of the stock market.
ML Model Testing
n:Time series to forecast
p:Price signals of MDLZ stock
j:Nash equilibria (Neural Network)
k:Dominated move of MDLZ stock holders
a:Best response for MDLZ target price
For further technical information as per how our model work we invite you to visit the article below:
How do KappaSignal algorithms actually work?
MDLZ Stock Forecast (Buy or Sell) Strategic Interaction Table
Strategic Interaction Table Legend:
X axis: *Likelihood% (The higher the percentage value, the more likely the event will occur.)
Y axis: *Potential Impact% (The higher the percentage value, the more likely the price will deviate.)
Z axis (Grey to Black): *Technical Analysis%
Mondelez International: A Solid Foundation for Future Growth
Mondelez International (MDLZ) is a multinational confectionery, food, and beverage giant with a robust portfolio of iconic brands across various categories. The company enjoys a strong market position, driven by its diverse product mix, extensive global reach, and established brand loyalty. MDLZ has a solid financial track record, with consistent revenue growth and profitability. The company's recent strategic initiatives, such as focusing on its core brands, optimizing its portfolio, and investing in emerging markets, are expected to drive continued growth in the coming years.
The global snacking market is experiencing a steady upward trend, fueled by factors such as rising urbanization, growing disposable incomes, and changing consumer preferences. MDLZ is well-positioned to capitalize on these trends. Its wide range of snacking options, from chocolate and biscuits to gum and coffee, caters to diverse consumer needs. The company's strong brand recognition and established distribution networks allow it to effectively reach target audiences worldwide. These factors are expected to drive continued demand for MDLZ products.
MDLZ is committed to innovation and product development, constantly introducing new flavors, formats, and healthier options to meet evolving consumer preferences. The company's focus on digital marketing and e-commerce channels will enhance its reach and connect with younger generations. Moreover, MDLZ's commitment to sustainability and responsible sourcing practices is a key differentiator in an increasingly conscious market.
While external factors like economic fluctuations and rising commodity prices pose potential risks, MDLZ has demonstrated its resilience and adaptability in the past. The company's strategic initiatives, combined with its strong brand portfolio and global footprint, position it for continued success in the years ahead. As the global snacking market continues to grow, MDLZ is expected to maintain its leadership position and deliver sustainable value to its shareholders.
Rating | Short-Term | Long-Term Senior |
---|---|---|
Outlook | Baa2 | Baa2 |
Income Statement | Baa2 | Baa2 |
Balance Sheet | Baa2 | Baa2 |
Leverage Ratios | Caa2 | Ba1 |
Cash Flow | Baa2 | Baa2 |
Rates of Return and Profitability | Ba1 | B1 |
*Financial analysis is the process of evaluating a company's financial performance and position by neural network. It involves reviewing the company's financial statements, including the balance sheet, income statement, and cash flow statement, as well as other financial reports and documents.
How does neural network examine financial reports and understand financial state of the company?
References
- Chamberlain G. 2000. Econometrics and decision theory. J. Econom. 95:255–83
- Li L, Chen S, Kleban J, Gupta A. 2014. Counterfactual estimation and optimization of click metrics for search engines: a case study. In Proceedings of the 24th International Conference on the World Wide Web, pp. 929–34. New York: ACM
- Athey S, Wager S. 2017. Efficient policy learning. arXiv:1702.02896 [math.ST]
- Zubizarreta JR. 2015. Stable weights that balance covariates for estimation with incomplete outcome data. J. Am. Stat. Assoc. 110:910–22
- Mnih A, Teh YW. 2012. A fast and simple algorithm for training neural probabilistic language models. In Proceedings of the 29th International Conference on Machine Learning, pp. 419–26. La Jolla, CA: Int. Mach. Learn. Soc.
- Mikolov T, Yih W, Zweig G. 2013c. Linguistic regularities in continuous space word representations. In Pro- ceedings of the 2013 Conference of the North American Chapter of the Association for Computational Linguistics: Human Language Technologies, pp. 746–51. New York: Assoc. Comput. Linguist.
- Chernozhukov V, Chetverikov D, Demirer M, Duflo E, Hansen C, et al. 2016a. Double machine learning for treatment and causal parameters. Tech. Rep., Cent. Microdata Methods Pract., Inst. Fiscal Stud., London