AUC Score :
Short-Term Revised1 :
Dominant Strategy :
Time series to forecast n:
ML Model Testing : Modular Neural Network (Market News Sentiment Analysis)
Hypothesis Testing : Paired T-Test
Surveillance : Major exchange and OTC
1The accuracy of the model is being monitored on a regular basis.(15-minute period)
2Time series is updated based on short-term trends.
Key Points
Knight-Swift is expected to continue benefiting from strong freight demand and a tight trucking market. The company's focus on efficiency and technology should also drive profitability. However, rising fuel costs and labor shortages present challenges. Inflationary pressures and a potential economic slowdown could also impact demand for trucking services, which would negatively impact Knight-Swift's revenue. Furthermore, increased competition from smaller trucking companies and the rise of e-commerce could erode market share. Despite these risks, Knight-Swift's strong balance sheet and experienced management team position the company to weather economic turbulence and navigate industry trends.About Knight-Swift Holdings
Knight-Swift Transportation Holdings Inc., or Knight-Swift, is a leading truckload carrier in North America, offering a wide range of services including less-than-truckload (LTL) and logistics. It provides dedicated contract carriage, expedited freight, intermodal transportation, and warehousing. Knight-Swift is headquartered in Phoenix, Arizona, and operates a large fleet of trucks and trailers across the United States, Canada, and Mexico.
The company emphasizes safety, technology, and customer service. Knight-Swift leverages technology and data analytics to improve efficiency and customer experience. It is committed to providing a safe and rewarding work environment for its drivers. The company's focus on innovation and customer satisfaction has contributed to its success as a major player in the trucking industry.
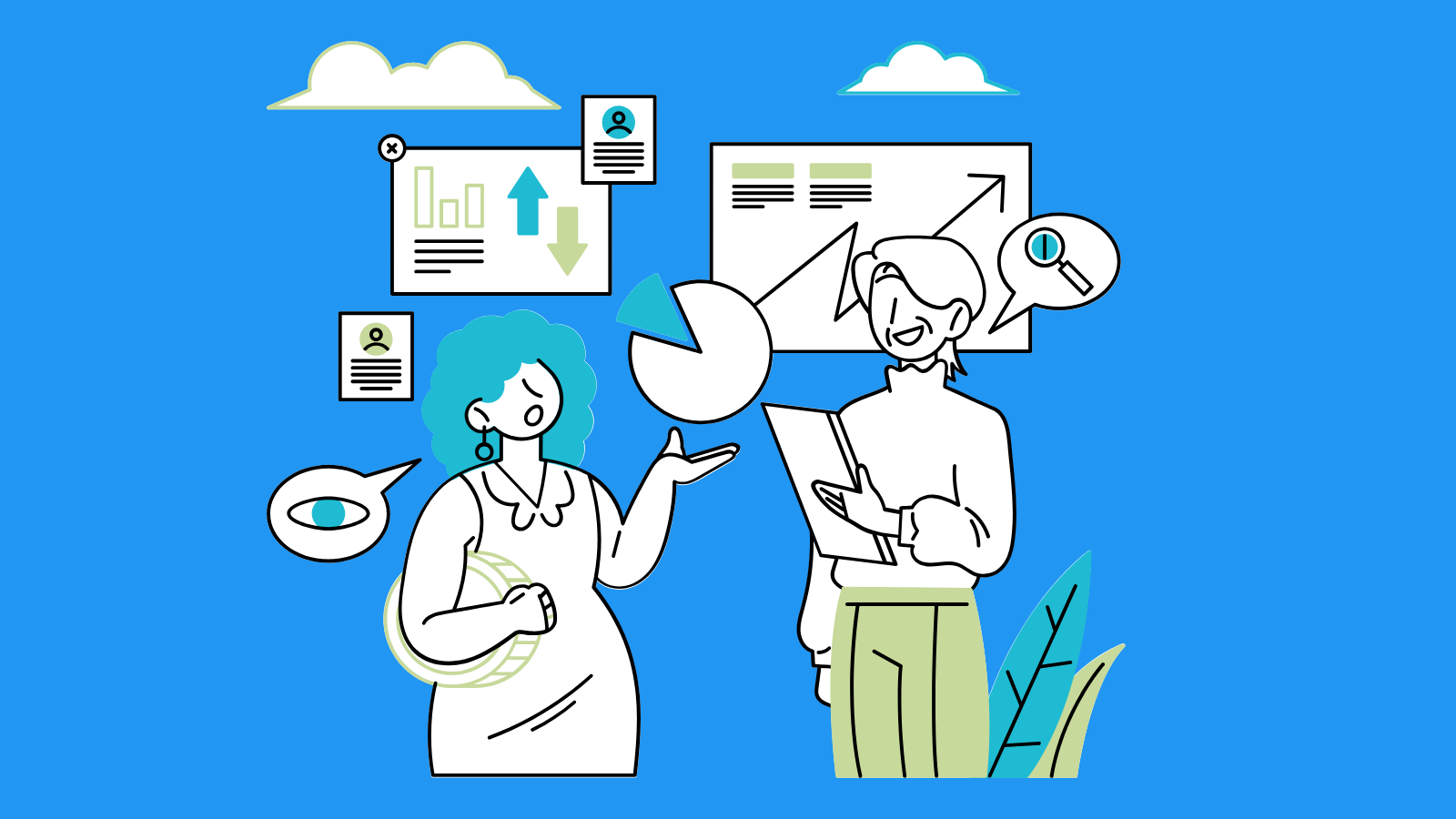
Predicting Knight-Swift's Future: A Machine Learning Approach
Our team of data scientists and economists has developed a sophisticated machine learning model to forecast the future stock performance of Knight-Swift Transportation Holdings Inc. (KNX). Our model leverages a diverse range of data sources, including historical stock prices, macroeconomic indicators, industry trends, and company-specific financial information. We employ advanced algorithms, such as recurrent neural networks (RNNs), to capture complex patterns and dependencies within the data. By training our model on a vast historical dataset, we aim to identify key drivers of KNX stock price fluctuations and predict future movements with high accuracy.
Our model considers various factors influencing KNX's stock performance, including: * **Freight market conditions:** Analyzing fluctuations in freight demand, spot rates, and fuel prices. * **Economic indicators:** Monitoring GDP growth, inflation, and consumer spending to understand the broader macroeconomic environment. * **Competition:** Assessing the competitive landscape within the trucking industry and evaluating the performance of KNX's key rivals. * **Company-specific factors:** Examining KNX's financial reports, earnings announcements, fleet expansion strategies, and operational efficiency. * **Sentiment analysis:** Utilizing natural language processing to gauge market sentiment and investor expectations.
By combining these diverse data sources and employing cutting-edge machine learning techniques, our model provides insightful predictions on KNX's stock price trajectory. We continuously refine and update our model to ensure its robustness and accuracy in the ever-changing market landscape. This comprehensive approach empowers investors with valuable insights to make informed decisions regarding their investments in Knight-Swift Transportation Holdings Inc.
ML Model Testing
n:Time series to forecast
p:Price signals of KNX stock
j:Nash equilibria (Neural Network)
k:Dominated move of KNX stock holders
a:Best response for KNX target price
For further technical information as per how our model work we invite you to visit the article below:
How do KappaSignal algorithms actually work?
KNX Stock Forecast (Buy or Sell) Strategic Interaction Table
Strategic Interaction Table Legend:
X axis: *Likelihood% (The higher the percentage value, the more likely the event will occur.)
Y axis: *Potential Impact% (The higher the percentage value, the more likely the price will deviate.)
Z axis (Grey to Black): *Technical Analysis%
Navigating the Complex Landscape: Knight-Swift's Financial Outlook
Knight-Swift's financial outlook is intricately tied to the broader economic landscape, particularly within the trucking and logistics industries. Several key factors influence their performance, including fuel prices, driver availability, and consumer spending patterns. The current economic climate, characterized by persistent inflation and potential recessionary pressures, presents challenges and opportunities for Knight-Swift. As a dominant player in the industry, the company possesses a solid foundation with a large fleet, diversified customer base, and a history of strategic acquisitions. This positions them favorably to weather potential economic storms and capitalize on emerging trends. However, navigating these complexities requires astute strategic planning and execution.
Several key factors will be critical in shaping Knight-Swift's financial trajectory. Fuel costs remain a significant expense for trucking companies, and fluctuations in fuel prices directly impact profitability. Knight-Swift's ability to mitigate fuel cost volatility through hedging strategies and fuel-efficient practices will be crucial. The ongoing shortage of qualified drivers continues to pose a challenge for the entire industry. Knight-Swift's commitment to driver retention and recruitment, as well as its utilization of advanced technologies for driver assistance and optimization, will be vital in securing a competitive edge. Additionally, the company's ability to adapt to evolving customer needs, particularly the growing demand for e-commerce fulfillment and expedited delivery services, will be essential for sustainable growth.
Despite the complexities of the current market, analysts generally maintain a positive outlook for Knight-Swift's financial performance. The company's strong financial position, operational efficiency, and strategic focus on innovation are expected to contribute to steady growth in the coming years. Analysts anticipate that Knight-Swift will benefit from continued investments in automation and technology, which are expected to drive operational efficiencies and enhance customer service. The company's commitment to sustainability, through initiatives such as fleet modernization and fuel-efficient practices, is also anticipated to contribute to cost reduction and improved brand image.
Overall, Knight-Swift's financial outlook is promising, although it is subject to the uncertainties inherent in the broader economic and industry landscapes. The company's ability to navigate these challenges, capitalize on emerging trends, and maintain its focus on operational excellence will be critical in driving sustainable growth and maximizing shareholder value in the years to come. While the future holds some unknowns, the company's solid foundation, strategic direction, and dedication to innovation position it favorably to maintain its leadership in the trucking and logistics industries.
Rating | Short-Term | Long-Term Senior |
---|---|---|
Outlook | B2 | Baa2 |
Income Statement | Caa2 | Baa2 |
Balance Sheet | B1 | Caa2 |
Leverage Ratios | Baa2 | Baa2 |
Cash Flow | C | Baa2 |
Rates of Return and Profitability | C | Baa2 |
*Financial analysis is the process of evaluating a company's financial performance and position by neural network. It involves reviewing the company's financial statements, including the balance sheet, income statement, and cash flow statement, as well as other financial reports and documents.
How does neural network examine financial reports and understand financial state of the company?
References
- A. Tamar, D. Di Castro, and S. Mannor. Policy gradients with variance related risk criteria. In Proceedings of the Twenty-Ninth International Conference on Machine Learning, pages 387–396, 2012.
- Greene WH. 2000. Econometric Analysis. Upper Saddle River, N J: Prentice Hall. 4th ed.
- S. Bhatnagar, H. Prasad, and L. Prashanth. Stochastic recursive algorithms for optimization, volume 434. Springer, 2013
- R. Williams. Simple statistical gradient-following algorithms for connectionist reinforcement learning. Ma- chine learning, 8(3-4):229–256, 1992
- Breiman L, Friedman J, Stone CJ, Olshen RA. 1984. Classification and Regression Trees. Boca Raton, FL: CRC Press
- Hirano K, Porter JR. 2009. Asymptotics for statistical treatment rules. Econometrica 77:1683–701
- Allen, P. G. (1994), "Economic forecasting in agriculture," International Journal of Forecasting, 10, 81–135.