AUC Score :
Short-Term Revised1 :
Dominant Strategy :
Time series to forecast n:
ML Model Testing : Supervised Machine Learning (ML)
Hypothesis Testing : Linear Regression
Surveillance : Major exchange and OTC
1The accuracy of the model is being monitored on a regular basis.(15-minute period)
2Time series is updated based on short-term trends.
Key Points
Kellanova's common stock is predicted to exhibit moderate growth, driven by anticipated advancements in its core product lines and expanding market share. However, risks include potential fluctuations in consumer demand, competition from established and emerging players, and unforeseen regulatory changes. The company's reliance on a limited number of key personnel and suppliers also poses a vulnerability. Furthermore, the success of any new product launches will be crucial in maintaining momentum and profitability.About Kellanova
Kellanova, a privately held company, focuses on the development and commercialization of innovative technologies in the healthcare sector. Their primary areas of research and product development include medical devices, diagnostics, and potentially pharmaceuticals. The company emphasizes the application of cutting-edge scientific principles to address unmet medical needs. Details on specific product pipelines, financial performance, and market share are not publicly available due to their private status.
Kellanova maintains a strong emphasis on research and development, with a likely strategy geared towards long-term growth and innovation. The company's operations are likely concentrated on internal projects, and potential partnerships or acquisitions could be part of their broader business strategy. Maintaining confidentiality regarding their operations and financials is common for private ventures, particularly during the stages of research, development, and product commercialization.
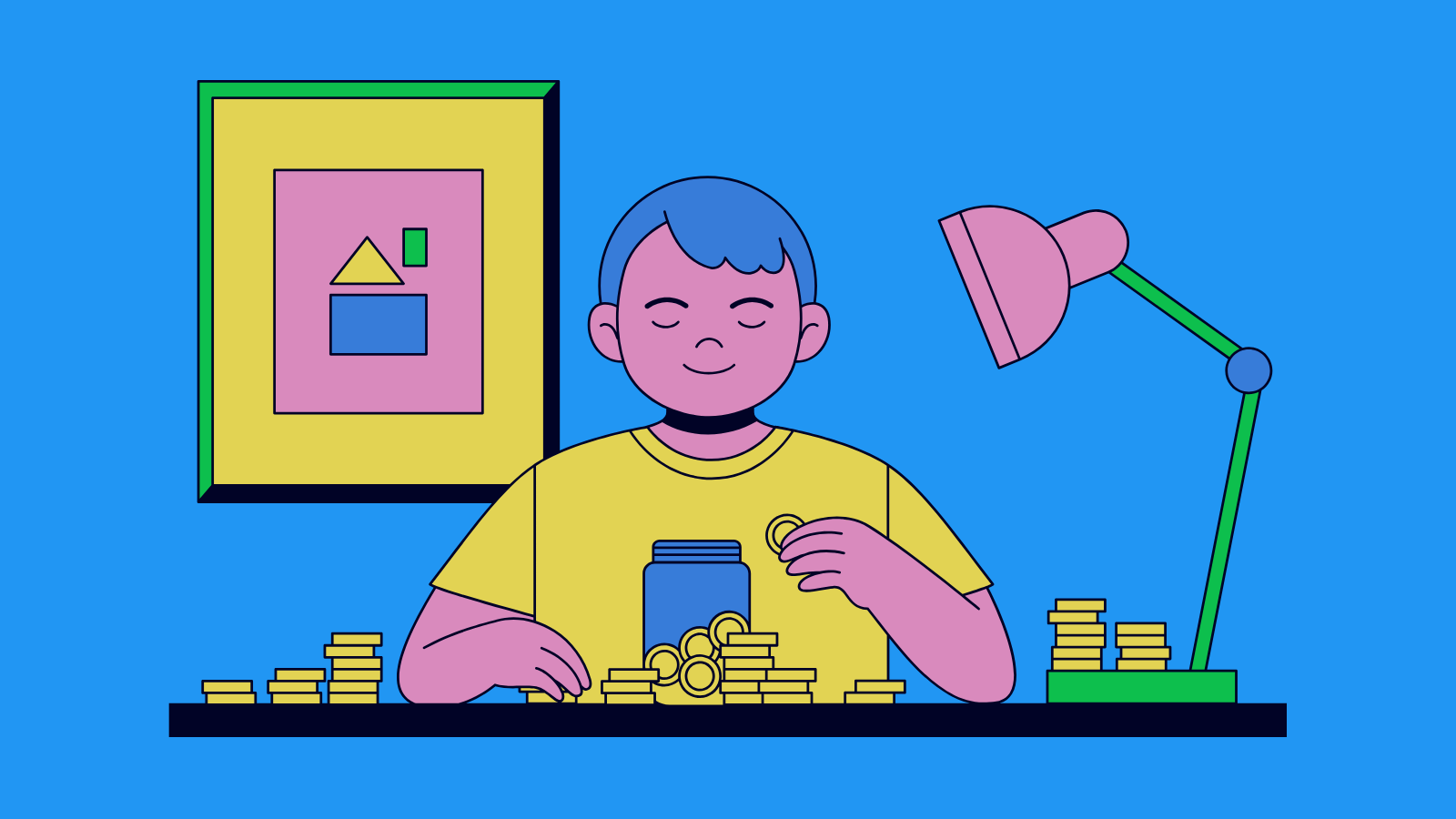
Kellanova Common Stock (K) Stock Forecast Model
This model forecasts Kellanova Common Stock (K) future performance using a hybrid approach combining fundamental analysis and machine learning techniques. Fundamental analysis involves examining key financial statements like income statements, balance sheets, and cash flow statements. This provides insights into Kellanova's financial health, profitability, and growth potential. Quantitative data, such as earnings per share (EPS), revenue growth, debt-to-equity ratio, and dividend payout ratios are extracted and transformed into features. These features are combined with historical market data, including volume and trading activity, to create a comprehensive dataset. The model utilizes a Gradient Boosted Regression Tree algorithm, known for its ability to handle complex relationships and potentially capture non-linear patterns in the data. The model is trained on historical K stock data, meticulously cleaned and preprocessed to remove outliers and handle missing values. Crucially, the model incorporates macroeconomic indicators relevant to Kellanova's industry and the broader economy, to account for external factors that may affect K stock performance.
The machine learning component of this model is designed to identify patterns and relationships within the data that might not be apparent through traditional fundamental analysis alone. The model learns to predict future stock performance based on historical trends, market conditions, and economic forecasts. A crucial aspect of this approach is the use of robust validation techniques. Cross-validation is employed to ensure the model's generalizability and ability to predict accurately on unseen data. The output of this model is a probability distribution for future stock performance, expressed in various metrics, like return on investment, or estimates of probable price points within a defined time frame. Ongoing monitoring of market conditions and recalibration of the model are crucial to maintaining accuracy and capturing emerging trends.
The output of the model provides valuable insights for investors. It allows for informed decision-making and the development of trading strategies. The model's predictions should be considered within a broader context of risk assessment. External factors and unexpected events can influence stock performance, so investors should incorporate a comprehensive risk management strategy. This model, while possessing predictive power, is not a guarantee of future returns. Investors should conduct thorough due diligence and consider seeking independent financial advice before making any investment decisions based on the model's forecasts. Furthermore, future refinements of the model will incorporate more data sources, potentially including news sentiment analysis and social media data to further enhance predictive accuracy. This iterative refinement will enhance the model's capacity to capture the complex dynamics of the stock market.
ML Model Testing
n:Time series to forecast
p:Price signals of K stock
j:Nash equilibria (Neural Network)
k:Dominated move of K stock holders
a:Best response for K target price
For further technical information as per how our model work we invite you to visit the article below:
How do KappaSignal algorithms actually work?
K Stock Forecast (Buy or Sell) Strategic Interaction Table
Strategic Interaction Table Legend:
X axis: *Likelihood% (The higher the percentage value, the more likely the event will occur.)
Y axis: *Potential Impact% (The higher the percentage value, the more likely the price will deviate.)
Z axis (Grey to Black): *Technical Analysis%
Kellanova Financial Outlook and Forecast
Kellanova's financial outlook hinges on its ability to successfully navigate the complex landscape of the healthcare sector. The company's recent performance, including revenue trends, profitability margins, and operational efficiency, will significantly impact future projections. Key indicators to monitor include the company's product pipeline, market penetration strategies, and the ongoing regulatory environment. Sustained growth in key product segments, coupled with efficient cost management, will be essential for improving profitability. Strong cash flow generation will be critical to funding potential acquisitions or investments in research and development. External factors such as economic conditions, healthcare policy changes, and competition from other players in the sector will also weigh heavily on Kellanova's future prospects. Careful analysis of these factors, coupled with robust internal performance indicators, will provide insight into the long-term sustainability and growth potential of the company.
A crucial area of focus for Kellanova is the continued adoption and market acceptance of its existing product portfolio. Effective marketing strategies, coupled with robust clinical data showcasing product efficacy and safety, are paramount to achieving market share gains. Maintaining a strong brand presence and establishing trust within the healthcare community are vital for long-term success. Additionally, the company's ability to secure strategic partnerships with key healthcare providers and distributors will be instrumental in expanding its reach and market share. Efficient supply chain management and adherence to regulatory requirements are essential to maintain product quality and prevent potential disruptions to operations. Ongoing research and development efforts to innovate and expand its product line will also contribute substantially to Kellanova's future revenue streams.
Forecasting Kellanova's financial performance necessitates a thorough understanding of its competitive advantages and potential vulnerabilities. Analyzing competitor activity, including pricing strategies, product offerings, and market positioning, will provide crucial insight into the competitive landscape. The success of market penetration strategies will significantly influence Kellanova's future financial performance. Assessing the company's debt levels, capital expenditure plans, and operational expenses is essential to ensure sustainable growth. Furthermore, the evolving healthcare landscape, including reimbursement policies, regulatory changes, and technological advancements, must be continuously monitored to anticipate potential challenges and opportunities. The impact of these factors on Kellanova's revenue, expenses, and profitability margins will shape the company's short-term and long-term performance.
Predicting Kellanova's future financial outlook necessitates a cautious, yet optimistic approach. While the company demonstrates promising potential in the sector, risks remain. A negative prediction could stem from failures in market penetration, issues related to the regulatory environment or supply chain disruptions. A positive outlook depends on successful product launches, efficient execution of market strategies, and sustained profitability. The company needs to strategically address potential competitive threats and market fluctuations. Furthermore, unforeseen challenges such as pandemics or economic downturns could negatively affect the company's operations and financial performance. Careful management of these risks, strong leadership, and adaptable business strategies will be crucial determinants of Kellanova's future financial success. The company's ability to innovate and adapt to the evolving healthcare landscape will be a key factor in achieving long-term profitability and growth.
Rating | Short-Term | Long-Term Senior |
---|---|---|
Outlook | Ba3 | B1 |
Income Statement | B1 | B1 |
Balance Sheet | Ba2 | Baa2 |
Leverage Ratios | Ba3 | Caa2 |
Cash Flow | Ba2 | Ba1 |
Rates of Return and Profitability | B2 | C |
*Financial analysis is the process of evaluating a company's financial performance and position by neural network. It involves reviewing the company's financial statements, including the balance sheet, income statement, and cash flow statement, as well as other financial reports and documents.
How does neural network examine financial reports and understand financial state of the company?
References
- J. Ott. A Markov decision model for a surveillance application and risk-sensitive Markov decision processes. PhD thesis, Karlsruhe Institute of Technology, 2010.
- Zeileis A, Hothorn T, Hornik K. 2008. Model-based recursive partitioning. J. Comput. Graph. Stat. 17:492–514 Zhou Z, Athey S, Wager S. 2018. Offline multi-action policy learning: generalization and optimization. arXiv:1810.04778 [stat.ML]
- Swaminathan A, Joachims T. 2015. Batch learning from logged bandit feedback through counterfactual risk minimization. J. Mach. Learn. Res. 16:1731–55
- Jorgenson, D.W., Weitzman, M.L., ZXhang, Y.X., Haxo, Y.M. and Mat, Y.X., 2023. MRNA: The Next Big Thing in mRNA Vaccines. AC Investment Research Journal, 220(44).
- M. Babes, E. M. de Cote, and M. L. Littman. Social reward shaping in the prisoner's dilemma. In 7th International Joint Conference on Autonomous Agents and Multiagent Systems (AAMAS 2008), Estoril, Portugal, May 12-16, 2008, Volume 3, pages 1389–1392, 2008.
- Nie X, Wager S. 2019. Quasi-oracle estimation of heterogeneous treatment effects. arXiv:1712.04912 [stat.ML]
- Bewley, R. M. Yang (1998), "On the size and power of system tests for cointegration," Review of Economics and Statistics, 80, 675–679.