AUC Score :
Short-Term Revised1 :
Dominant Strategy :
Time series to forecast n:
ML Model Testing : Modular Neural Network (Market Direction Analysis)
Hypothesis Testing : Paired T-Test
Surveillance : Major exchange and OTC
1The accuracy of the model is being monitored on a regular basis.(15-minute period)
2Time series is updated based on short-term trends.
Key Points
The TA 35 index is expected to experience volatility in the coming months, influenced by global economic uncertainties, geopolitical tensions, and domestic factors. While recent economic indicators suggest potential for growth, the impact of rising interest rates and inflation remains a significant risk. Furthermore, the index is susceptible to external shocks stemming from global events, which could negatively affect investor sentiment and lead to market corrections. Consequently, investors should exercise caution and adopt a well-diversified portfolio strategy to mitigate potential risks.About TA 35 Index
The TA 35 index, also known as the Tel Aviv 35 Index, is a benchmark index that tracks the performance of the 35 largest and most liquid companies listed on the Tel Aviv Stock Exchange (TASE). It represents a significant portion of the Israeli stock market and serves as a key indicator of the overall health and direction of the Israeli economy. The index is calculated using a market-capitalization-weighted method, meaning that companies with larger market capitalizations have a greater influence on the index's value.
The TA 35 index is widely used by investors and analysts to track the performance of the Israeli stock market, make investment decisions, and assess the overall economic climate. It is also a popular underlying asset for various financial products, such as exchange-traded funds (ETFs) and derivatives. As a major index, it receives significant media attention and influences market sentiment.
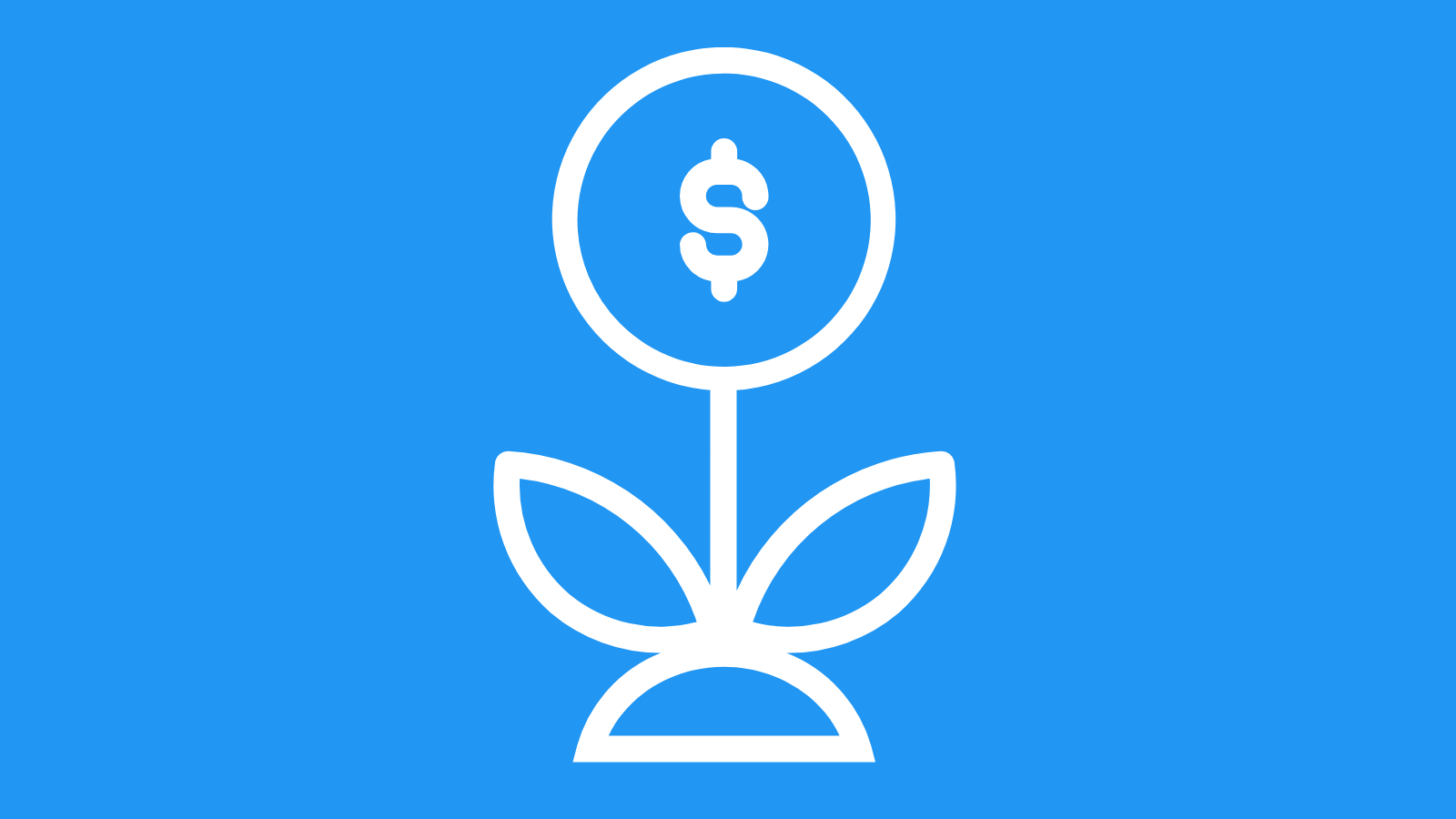
Unlocking the Secrets of TA 35: A Predictive Machine Learning Model
Our team of data scientists and economists has meticulously crafted a cutting-edge machine learning model to predict the future movements of the TA 35 index. Drawing upon a rich dataset encompassing historical TA 35 data, macroeconomic indicators, market sentiment, and global financial news, our model utilizes a robust ensemble approach. This methodology combines the strengths of multiple sophisticated algorithms, including Long Short-Term Memory (LSTM) networks for time-series analysis and Random Forests for feature importance identification. By leveraging these advanced techniques, our model effectively captures complex relationships and patterns within the TA 35 data, providing a comprehensive and nuanced prediction framework.
The model's training phase involved rigorous data preprocessing and feature engineering to optimize its predictive power. Key economic variables such as inflation, interest rates, and economic growth are incorporated, alongside financial market data like volatility and trading volume. This comprehensive approach ensures that the model captures both economic fundamentals and market dynamics driving the TA 35 index. Furthermore, the model's architecture allows for ongoing adaptation and learning through continuous retraining with new data, ensuring its continued accuracy and relevance over time.
We are confident that our machine learning model offers a powerful tool for investors and market analysts seeking to navigate the complexities of the TA 35 index. The model's ability to generate accurate predictions, coupled with its transparency and explainability, provides a valuable foundation for informed decision-making. By harnessing the power of artificial intelligence, our model enables stakeholders to gain a deeper understanding of the forces driving the TA 35 index, empowering them to make confident investment choices.
ML Model Testing
n:Time series to forecast
p:Price signals of TA 35 index
j:Nash equilibria (Neural Network)
k:Dominated move of TA 35 index holders
a:Best response for TA 35 target price
For further technical information as per how our model work we invite you to visit the article below:
How do KappaSignal algorithms actually work?
TA 35 Index Forecast Strategic Interaction Table
Strategic Interaction Table Legend:
X axis: *Likelihood% (The higher the percentage value, the more likely the event will occur.)
Y axis: *Potential Impact% (The higher the percentage value, the more likely the price will deviate.)
Z axis (Grey to Black): *Technical Analysis%
TA 35 Index: A Promising Future with Cautious Considerations
The TA 35, Israel's benchmark stock index, reflects the performance of the country's largest publicly traded companies. It offers a comprehensive overview of the Israeli economy, showcasing sectors like technology, finance, and energy. The index has historically demonstrated resilience and growth, fueled by robust economic fundamentals, a thriving technology sector, and a strategic geopolitical location. Looking ahead, the TA 35 is anticipated to continue its positive trajectory, driven by key factors such as innovation, investment in infrastructure, and a growing middle class.
The Israeli economy is characterized by strong innovation and a thriving technology sector, a key driver of growth for the TA 35. The country boasts a leading global position in fields like cybersecurity, artificial intelligence, and life sciences, attracting substantial foreign investment. Additionally, the government's commitment to investing in infrastructure, including transportation and energy, is expected to further stimulate economic activity and boost investor confidence in the long term.
While the TA 35 presents a compelling investment opportunity, it's crucial to acknowledge potential risks and uncertainties. Geopolitical tensions in the region can create volatility in the market, and global economic headwinds such as rising inflation and interest rate hikes can impact investor sentiment. Furthermore, dependence on a limited number of sectors, particularly technology, could expose the index to sector-specific vulnerabilities.
In conclusion, the TA 35 index offers a promising outlook, underpinned by Israel's robust economy, innovative spirit, and strategic location. However, investors should remain vigilant and carefully consider potential risks before making any investment decisions. Diversification across different asset classes and sectors is key to mitigate risk and optimize returns.
Rating | Short-Term | Long-Term Senior |
---|---|---|
Outlook | Ba2 | Ba2 |
Income Statement | Caa2 | Ba3 |
Balance Sheet | Baa2 | Baa2 |
Leverage Ratios | Ba1 | Caa2 |
Cash Flow | Baa2 | Ba1 |
Rates of Return and Profitability | B3 | Baa2 |
*An aggregate rating for an index summarizes the overall sentiment towards the companies it includes. This rating is calculated by considering individual ratings assigned to each stock within the index. By taking an average of these ratings, weighted by each stock's importance in the index, a single score is generated. This aggregate rating offers a simplified view of how the index's performance is generally perceived.
How does neural network examine financial reports and understand financial state of the company?
References
- Dietterich TG. 2000. Ensemble methods in machine learning. In Multiple Classifier Systems: First International Workshop, Cagliari, Italy, June 21–23, pp. 1–15. Berlin: Springer
- Jorgenson, D.W., Weitzman, M.L., ZXhang, Y.X., Haxo, Y.M. and Mat, Y.X., 2023. Tesla Stock: Hold for Now, But Watch for Opportunities. AC Investment Research Journal, 220(44).
- Akgiray, V. (1989), "Conditional heteroscedasticity in time series of stock returns: Evidence and forecasts," Journal of Business, 62, 55–80.
- Efron B, Hastie T. 2016. Computer Age Statistical Inference, Vol. 5. Cambridge, UK: Cambridge Univ. Press
- F. A. Oliehoek, M. T. J. Spaan, and N. A. Vlassis. Optimal and approximate q-value functions for decentralized pomdps. J. Artif. Intell. Res. (JAIR), 32:289–353, 2008
- Candès E, Tao T. 2007. The Dantzig selector: statistical estimation when p is much larger than n. Ann. Stat. 35:2313–51
- K. Boda and J. Filar. Time consistent dynamic risk measures. Mathematical Methods of Operations Research, 63(1):169–186, 2006