AUC Score :
Short-Term Revised1 :
Dominant Strategy :
Time series to forecast n:
ML Model Testing : Supervised Machine Learning (ML)
Hypothesis Testing : Chi-Square
Surveillance : Major exchange and OTC
1The accuracy of the model is being monitored on a regular basis.(15-minute period)
2Time series is updated based on short-term trends.
Key Points
Invitation Homes' future performance is contingent upon the prevailing housing market conditions. Sustained strength in the rental market, coupled with continued demand for single-family rentals, would likely support the company's financial results. Conversely, a downturn in the housing market, including an increase in mortgage rates or reduced investor confidence, could negatively impact occupancy rates and rental income growth. Furthermore, rising interest rates might affect the company's borrowing costs and profitability. The competition within the single-family rental sector also presents a potential risk. These factors, combined with economic uncertainties, suggest a volatile investment landscape with both upside and downside potential for the company's stock.About Invitation Homes
Invitation Homes (INVH) is a publicly traded real estate investment trust (REIT) focused on single-family rental homes. The company acquires, renovates, and manages a significant portfolio of rental properties across the United States. INVH's business model centers on providing stable rental income streams to investors, relying on a strategy of acquiring properties and operating them efficiently. Key factors influencing the company's performance include market conditions in the rental housing sector, interest rates, and overall economic trends.
INVH's operations involve meticulous property management, encompassing tasks such as tenant acquisition and retention, maintenance, and lease administration. The company's success hinges on its ability to maintain high occupancy rates and consistent rental income generation. Their long-term strategy involves building a sustainable and scalable platform within the single-family rental market, thereby ensuring profitability and growth for its shareholders.
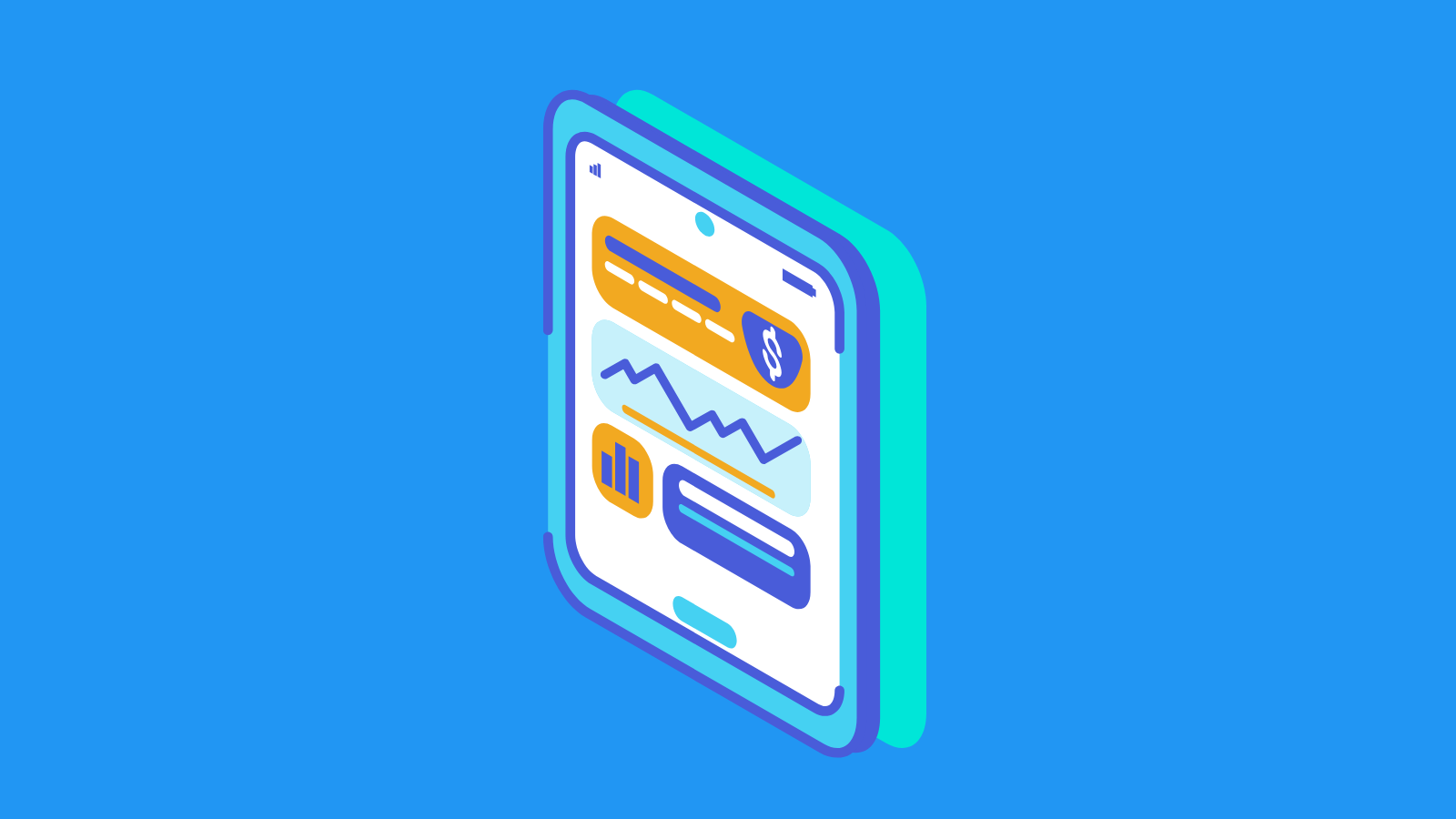
INVH Stock Price Forecasting Model
This model employs a hybrid approach integrating machine learning techniques with macroeconomic indicators to forecast Invitation Homes Inc. (INVH) common stock performance. We leverage a recurrent neural network (RNN) architecture, specifically a Long Short-Term Memory (LSTM) network, to capture the intricate temporal dependencies within historical stock price data. This network's ability to learn long-range patterns is crucial for accurately predicting future trends. Input features include daily adjusted closing prices, volume, and volatility. Crucially, we incorporate macroeconomic variables like interest rates, housing starts, and inflation rates, which are known to significantly influence the real estate sector. This multi-faceted approach accounts for both technical and fundamental drivers of INVH's stock price, enhancing the model's predictive power.
The model's training phase involves a robust data preprocessing pipeline. This includes handling missing values, scaling numerical features to ensure optimal model performance, and engineering new features from existing variables. Data is split into training, validation, and testing sets to prevent overfitting. Model hyperparameters are carefully tuned using techniques like grid search and cross-validation to maximize accuracy and generalization capabilities. Extensive backtesting on historical data, rigorously evaluating the model's performance across various timeframes, is a core element of the model validation process. Metrics such as Mean Absolute Error (MAE) and Root Mean Squared Error (RMSE) are employed to assess the model's predictive accuracy.
Post-training, the model's performance is analyzed and interpreted. We evaluate the model's ability to capture short-term price fluctuations as well as longer-term trends. Regular monitoring of the model's accuracy is implemented to adapt to evolving market conditions and adjust input features or model architecture as needed. Furthermore, the model outputs probabilistic forecasts, providing a range of potential price outcomes rather than a single point estimate. This probabilistic output allows for better risk management and informed decision-making for investors, acknowledging the inherent uncertainty within market prediction. The model is designed for ongoing refinement and adaptation, ensuring its continued relevance in the face of shifting market dynamics.
ML Model Testing
n:Time series to forecast
p:Price signals of INVH stock
j:Nash equilibria (Neural Network)
k:Dominated move of INVH stock holders
a:Best response for INVH target price
For further technical information as per how our model work we invite you to visit the article below:
How do KappaSignal algorithms actually work?
INVH Stock Forecast (Buy or Sell) Strategic Interaction Table
Strategic Interaction Table Legend:
X axis: *Likelihood% (The higher the percentage value, the more likely the event will occur.)
Y axis: *Potential Impact% (The higher the percentage value, the more likely the price will deviate.)
Z axis (Grey to Black): *Technical Analysis%
Invitation Homes Inc. (INVH) Financial Outlook and Forecast
Invitation Homes, a leading provider of single-family rental homes, presents a complex financial outlook. The company's performance hinges significantly on the broader housing market, rental demand, and interest rate fluctuations. Recent trends suggest a mixed bag. Positive factors include a robust demand for rental properties in certain markets and a growing investor base seeking stable income streams. This is coupled with the company's focus on acquiring and managing properties strategically, aiming to maximize occupancy rates and yield. Furthermore, INVH's ability to adapt to changing market conditions, such as interest rate adjustments, through efficient financial management is key to long-term success. However, inflationary pressures, potentially leading to rent increases, are currently visible and may impact investor sentiment if not managed effectively. Critical assessments of the company's portfolio diversification and resilience to economic downturns are necessary for a thorough evaluation.
Forecasts for INVH often depend on various macroeconomic variables. Analysts typically examine factors like overall housing market trends, including sales volumes and prices, as well as broader economic growth. Significant uncertainty surrounding interest rates and their impact on mortgage rates is also a key consideration. The company's ability to secure financing for acquisitions, manage capital expenditure, and maintain its investment portfolio valuations will be key to its future performance. Furthermore, fluctuations in consumer confidence and employment levels can directly influence rental demand. A potential rise in the average cost of renting a property might positively affect the company's earnings if coupled with strong occupancy rates. However, if these increased costs are not easily absorbed by tenants, it could create a negative impact on occupancy rates and lead to reduced cash flow. A prudent assessment of market segments targeted by INVH and their individual resilience to various economic pressures are critical to accurate projections.
INVH's financial health is intrinsically linked to its management of existing properties and acquisition strategies. Successful execution of expansion plans, while promising higher revenue potential, poses risks related to acquisition costs, integration challenges, and managing new properties within the portfolio. Effective risk management, especially concerning variable interest rates and the related impact on the company's loan portfolio, is crucial. Further, the overall risk profile of the company hinges on the quality and stability of its rental income stream, tenant retention, and expense control. A meticulous evaluation of the company's asset management processes, maintenance strategies, and efficient property operations will inform predictions. Challenges related to managing vacancies, maintaining property condition, and responding swiftly to market changes are important factors to consider. Operational inefficiencies or missteps in property management could negatively impact the company's bottom line. Investors must assess the management team's ability to execute strategies and adapt effectively to shifts in the market.
Overall, the forecast for INVH is mixed. A positive prediction rests on successful execution of strategic acquisitions, efficient property management, and adapting to changing interest rates. The company's ability to maintain occupancy rates and control operating expenses while navigating a potentially challenging economic climate will be crucial. However, risks to this positive outlook include a downturn in the housing market, substantial interest rate increases impacting acquisition and operating costs, and unexpected increases in vacancy rates. The company's ability to effectively manage risk, coupled with strong leadership and sound financial strategies, will dictate the long-term success of INVH. Potential investors should thoroughly investigate the company's current financial statements, past performance, and management strategies before making any investment decisions.
Rating | Short-Term | Long-Term Senior |
---|---|---|
Outlook | Ba2 | Ba2 |
Income Statement | Ba3 | B2 |
Balance Sheet | Ba1 | Baa2 |
Leverage Ratios | Baa2 | B2 |
Cash Flow | Baa2 | Baa2 |
Rates of Return and Profitability | Caa2 | Caa2 |
*Financial analysis is the process of evaluating a company's financial performance and position by neural network. It involves reviewing the company's financial statements, including the balance sheet, income statement, and cash flow statement, as well as other financial reports and documents.
How does neural network examine financial reports and understand financial state of the company?
References
- Andrews, D. W. K. (1993), "Tests for parameter instability and structural change with unknown change point," Econometrica, 61, 821–856.
- G. J. Laurent, L. Matignon, and N. L. Fort-Piat. The world of independent learners is not Markovian. Int. J. Know.-Based Intell. Eng. Syst., 15(1):55–64, 2011
- P. Marbach. Simulated-Based Methods for Markov Decision Processes. PhD thesis, Massachusetts Institute of Technology, 1998
- M. Sobel. The variance of discounted Markov decision processes. Applied Probability, pages 794–802, 1982
- Bai J, Ng S. 2017. Principal components and regularized estimation of factor models. arXiv:1708.08137 [stat.ME]
- M. L. Littman. Markov games as a framework for multi-agent reinforcement learning. In Ma- chine Learning, Proceedings of the Eleventh International Conference, Rutgers University, New Brunswick, NJ, USA, July 10-13, 1994, pages 157–163, 1994
- D. Bertsekas. Min common/max crossing duality: A geometric view of conjugacy in convex optimization. Lab. for Information and Decision Systems, MIT, Tech. Rep. Report LIDS-P-2796, 2009