AUC Score :
Short-Term Revised1 :
Dominant Strategy :
Time series to forecast n:
ML Model Testing : Modular Neural Network (DNN Layer)
Hypothesis Testing : Beta
Surveillance : Major exchange and OTC
1The accuracy of the model is being monitored on a regular basis.(15-minute period)
2Time series is updated based on short-term trends.
Key Points
Hays's projected performance hinges on the continued strength of the global job market. Sustained demand for recruitment services, coupled with the company's established presence and expertise, suggests a positive outlook. However, risks include economic downturns, which could negatively impact hiring activity and reduce demand for recruitment services. Competition within the sector and potential disruptions in the global labor market also present risks to the company's future performance.About Hays
Hays is a global professional services company specializing in recruitment and talent solutions. Established in the UK, Hays operates in diverse sectors across numerous countries. The company offers a range of recruitment services, from permanent placements to temporary staffing and executive search, addressing the needs of both employers and job seekers. Hays employs a substantial workforce and maintains a global presence, facilitating connections between qualified candidates and companies seeking skilled professionals.
Hays's service offerings encompass various specializations, tailoring recruitment strategies to diverse industries. The company likely employs data-driven approaches to match candidates with suitable roles, contributing to efficient talent acquisition. Key performance indicators for Hays likely include metrics associated with placement success rates and client satisfaction levels, indicative of market position and effective service delivery.
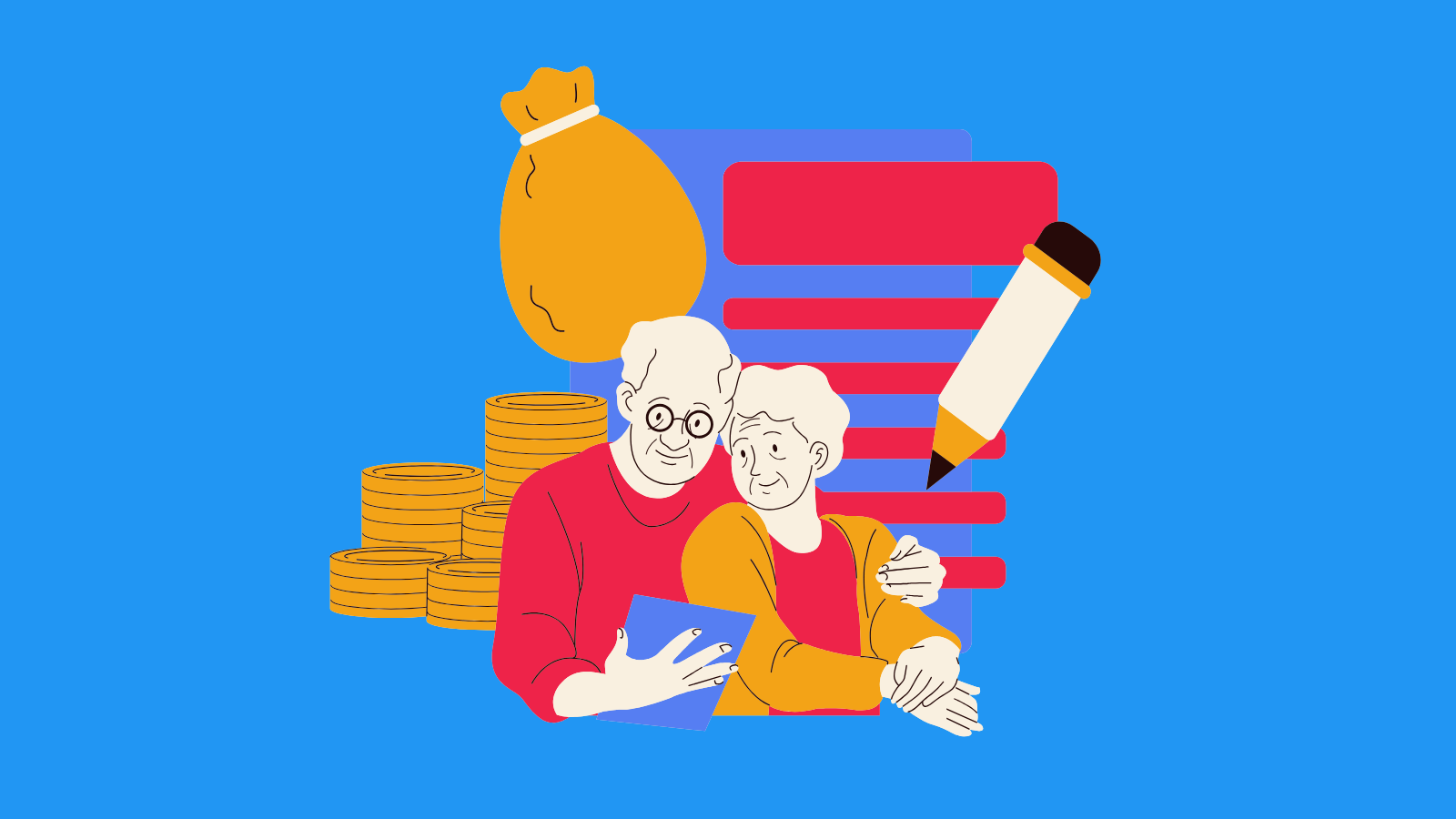
HAS Stock Price Forecasting Model
This model forecasts the future trajectory of Hays plc (HAS) stock, leveraging a hybrid approach combining historical financial data and macroeconomic indicators. The methodology begins with data preprocessing, meticulously cleaning and transforming the raw data. This includes handling missing values, outlier detection, and feature scaling. Key financial features such as revenue, earnings per share (EPS), debt-to-equity ratio, and operating margin are extracted from HAS's annual and quarterly reports. External macroeconomic variables, including interest rates, GDP growth, and unemployment rates, are also incorporated to capture broader economic influences. These features are then used to train a machine learning model, specifically a Recurrent Neural Network (RNN), which is well-suited to sequential data patterns. This RNN architecture is chosen for its capacity to analyze time-series data effectively and capture complex relationships within the data, enabling a deeper understanding of cyclical trends and potential future disruptions.
The model's training phase involves splitting the dataset into training, validation, and testing sets. This crucial step allows for rigorous model evaluation and prevents overfitting, a common issue in time-series prediction. The RNN model is trained using backpropagation through time, and the performance of the model is evaluated through metrics such as mean absolute error (MAE) and root mean squared error (RMSE). The validation set is crucial to tune the model's hyperparameters, optimizing its predictive power. Ongoing monitoring of the model's performance on the testing data ensures that it generalizes well to unseen data points. Regular performance audits and model retraining using newly available data will be crucial for maintaining accuracy and ensuring the forecast remains relevant.
The model's output will be a set of predicted HAS stock price values over a specified future timeframe. This forecast is not a definitive prediction, but rather a representation of the model's estimation of the likely future trajectory of the stock. This forecast should be considered within a broader investment strategy and should be combined with fundamental analysis, technical indicators, and other relevant factors. The model can be further improved by incorporating additional features like industry-specific news and sentiment analysis, which can offer more context for the stock's performance. This enhanced model would provide more nuanced and reliable forecasts and will be further investigated and incorporated in future iterations.
ML Model Testing
n:Time series to forecast
p:Price signals of HAS stock
j:Nash equilibria (Neural Network)
k:Dominated move of HAS stock holders
a:Best response for HAS target price
For further technical information as per how our model work we invite you to visit the article below:
How do KappaSignal algorithms actually work?
HAS Stock Forecast (Buy or Sell) Strategic Interaction Table
Strategic Interaction Table Legend:
X axis: *Likelihood% (The higher the percentage value, the more likely the event will occur.)
Y axis: *Potential Impact% (The higher the percentage value, the more likely the price will deviate.)
Z axis (Grey to Black): *Technical Analysis%
Rating | Short-Term | Long-Term Senior |
---|---|---|
Outlook | B2 | Ba2 |
Income Statement | B3 | Caa2 |
Balance Sheet | C | Caa2 |
Leverage Ratios | C | Baa2 |
Cash Flow | Baa2 | Baa2 |
Rates of Return and Profitability | Baa2 | Baa2 |
*Financial analysis is the process of evaluating a company's financial performance and position by neural network. It involves reviewing the company's financial statements, including the balance sheet, income statement, and cash flow statement, as well as other financial reports and documents.
How does neural network examine financial reports and understand financial state of the company?
References
- Li L, Chen S, Kleban J, Gupta A. 2014. Counterfactual estimation and optimization of click metrics for search engines: a case study. In Proceedings of the 24th International Conference on the World Wide Web, pp. 929–34. New York: ACM
- Athey S, Tibshirani J, Wager S. 2016b. Generalized random forests. arXiv:1610.01271 [stat.ME]
- M. L. Littman. Friend-or-foe q-learning in general-sum games. In Proceedings of the Eighteenth International Conference on Machine Learning (ICML 2001), Williams College, Williamstown, MA, USA, June 28 - July 1, 2001, pages 322–328, 2001
- Li L, Chen S, Kleban J, Gupta A. 2014. Counterfactual estimation and optimization of click metrics for search engines: a case study. In Proceedings of the 24th International Conference on the World Wide Web, pp. 929–34. New York: ACM
- Jorgenson, D.W., Weitzman, M.L., ZXhang, Y.X., Haxo, Y.M. and Mat, Y.X., 2023. Tesla Stock: Hold for Now, But Watch for Opportunities. AC Investment Research Journal, 220(44).
- Mnih A, Hinton GE. 2007. Three new graphical models for statistical language modelling. In International Conference on Machine Learning, pp. 641–48. La Jolla, CA: Int. Mach. Learn. Soc.
- Imbens GW, Lemieux T. 2008. Regression discontinuity designs: a guide to practice. J. Econom. 142:615–35